Lung Nodule Detection Based on the Combination of Morphometric and Texture Features
JOURNAL OF MEDICAL IMAGING AND HEALTH INFORMATICS(2018)
摘要
Lung cancer is one type of cancer with highest mortality rate in the world. Early detection and diagnosis could significantly increase the survival rate of lung cancer patients. In order to assist specialists in this search and identification of lung nodules in CT images, we propose a lung nodule detection framework where the combination of morphometric and texture features is used. Threshold is applied in the lung segmentation and candidate segmentation stage. This work has the purpose of developing a methodology for automatic detection of small lung nodules (with sizes between 3 and 10 mm). Training and tuning of all modules in our CADe system is done using the dataset created by the Lung Image Database Consortium (LIDC). 77 nodules are segmented from 80 nodules with an accuracy rate of 96.25%, and we use Tsallis entropy to distinguish them. After the segmentation stage, 2D and 3D features are computed for every candidate nodule. To distinguish between nodules and non-nodules more effectively, A sequential forward selection process is used to determine the optimum subset of features for SVM classifiers. Finally, leave-one-out method is adopted to train and test the segmented nodules with SVM classifier, and the detection system showed a sensitivity of 86.36% with 5.4 false positives per case.
更多查看译文
关键词
Computer-Aided Detection,LIDC,Lung Nodules,Medical Image,Tsallis Entropy,Support Vector Machine
AI 理解论文
溯源树
样例
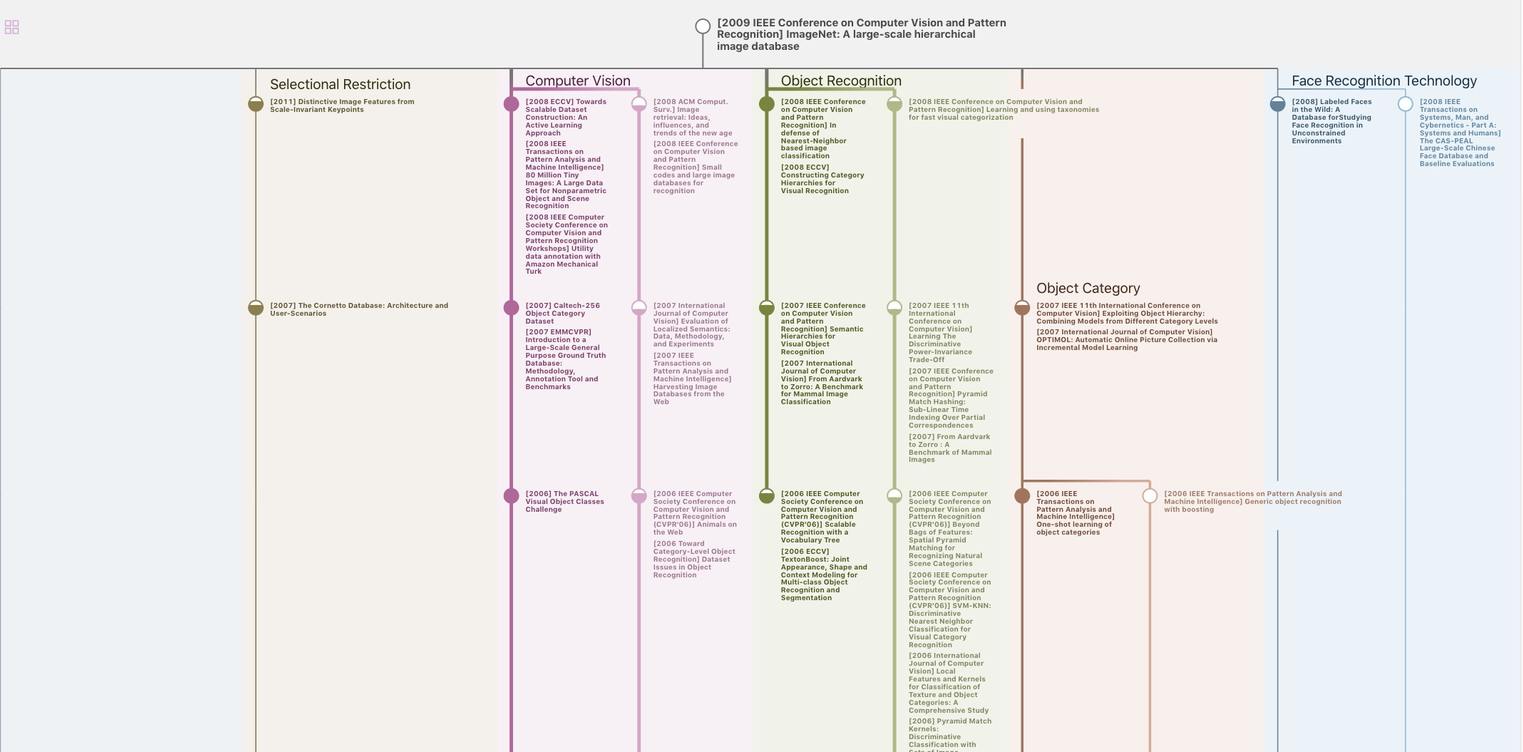
生成溯源树,研究论文发展脉络
Chat Paper
正在生成论文摘要