Models that learn how humans learn: the case of depression and bipolar disorders
bioRxiv(2018)
摘要
Computational models of learning and decision-making processes in the brain play an important role in many domains. Such models typically have a constrained structure and make specific assumptions about the underlying human learning processes; these may make them underfit observed behaviours. Here we suggest an alternative method based on learning-to-learn approaches, using recurrent neural networks (RNNs) as a flexible family of models that have sufficient capacity to represent the complex learning and decision-making strategies used by humans. In this approach, an RNN is trained to predict the next action that a subject will take in a decision-making task, and in this way, learns to imitate the processes underlying subjects9 choices and their learning abilities. We demonstrate the benefits of this approach with a new dataset containing behaviour of uni-polar depression (n=34), bipolar (n=33) and control (n=34) participants in a two-armed bandit task. The results indicate that the new approach is better than baseline reinforcement-learning methods in terms of overall performance and its capacity to predict subjects9 choices. We show that the model can be interpreted using off-policy simulations, and thereby provide a novel clustering of subjects9 learning processes -- something that often eludes traditional approaches to modelling and behavioural analysis.
更多查看译文
AI 理解论文
溯源树
样例
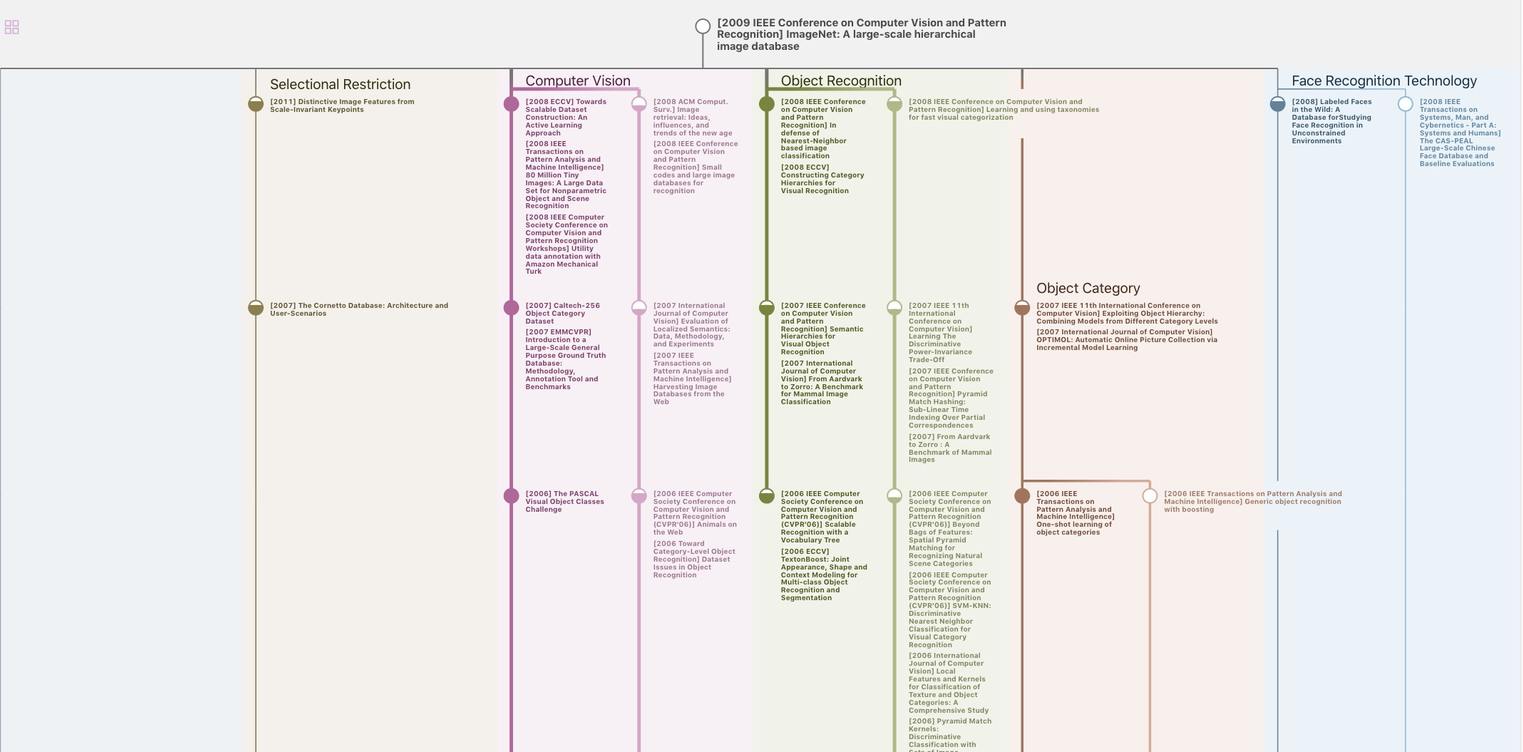
生成溯源树,研究论文发展脉络
Chat Paper
正在生成论文摘要