Multivariate Prediction Intervals for Photovoltaic Power Generation
2017 IEEE INNOVATIVE SMART GRID TECHNOLOGIES - ASIA (ISGT-ASIA)(2018)
摘要
The current literature in probabilistic forecasting is focused on quantifying the uncertainty of each random variable individually. This leads to the failure in informing about interdependence structure of uncertainty at different locations and/or different lead times. When there is a positive or negative association between a number of random variables, the prediction regions for them should be reflected by multivariate or joint uncertainty sets. The existing literature is very primitive in the area of multivariate uncertainty sets modeling. In this paper, uncertainty regions are generated in the form of multivariate prediction intervals. We will examine the performance of Gaussian and R-Vine copulas in characterizing the correlated behavior of PV power generations at successive lead-times. Copulas are compared based on goodness-of-fit metrics as well as skill scores. A framework is elaborated to generate multivariate prediction intervals out of the scenarios generated from Gaussian and R-vine multivariate densities. The resultant multivariate prediction intervals are evaluated based on their calibration and sharpness. The approaches are tested on a real-world dataset including PV power measurements and weather forecasts. This paper provides a series of useful analyses and comparative results for multivariate uncertainty modeling of PV power that can serve as a basis for future works in the area.
更多查看译文
关键词
Forecasting, Gaussian copula, multivariate prediction interval, photovoltaic power, R-Vine copula
AI 理解论文
溯源树
样例
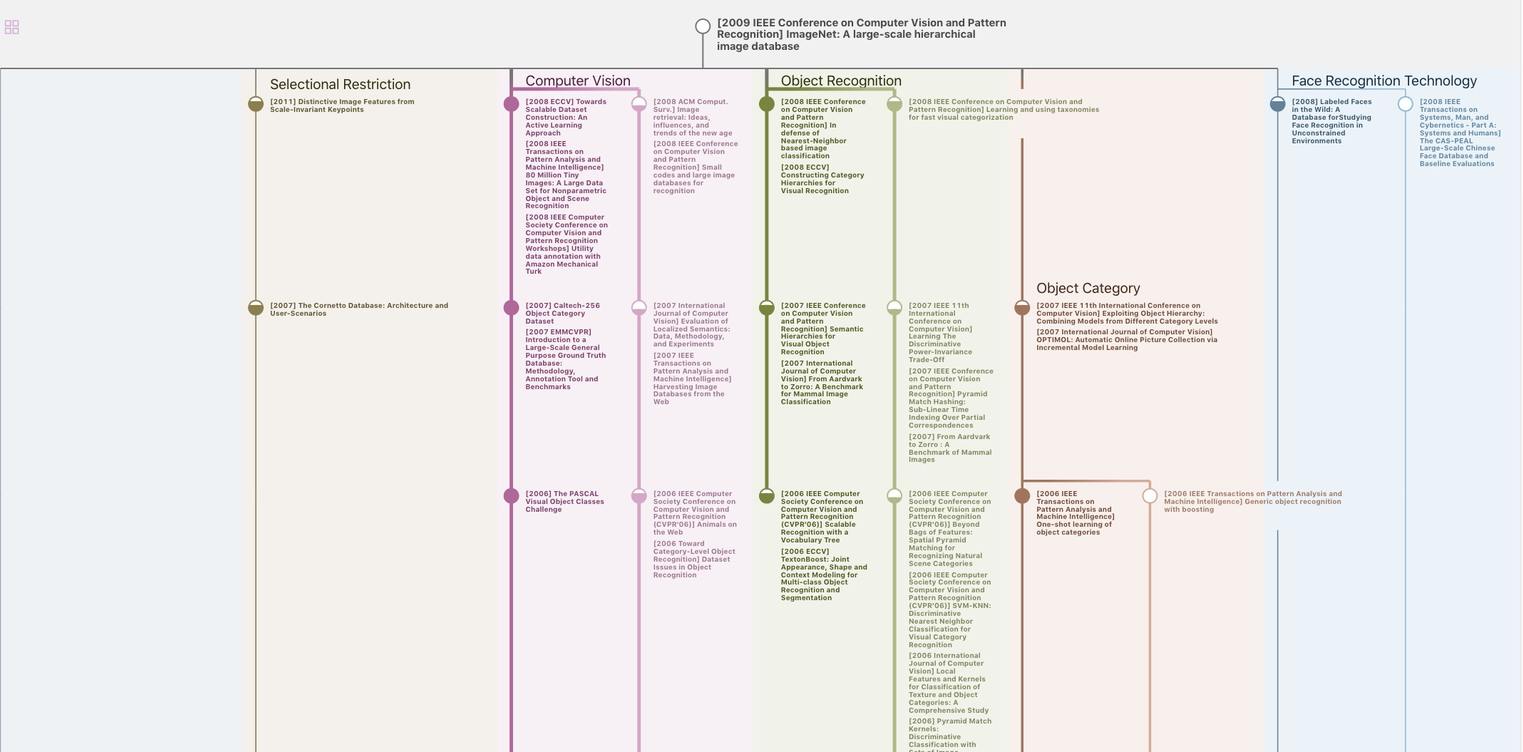
生成溯源树,研究论文发展脉络
Chat Paper
正在生成论文摘要