Bayesian Optimal Design for Ordinary Differential Equation Models With Application in Biological Science
JOURNAL OF THE AMERICAN STATISTICAL ASSOCIATION(2020)
摘要
Bayesian optimal design is considered for experiments where the response distribution depends on the solution to a system of nonlinear ordinary differential equations. The motivation is an experiment to estimate parameters in the equations governing the transport of amino acids through cell membranes in human placentas. Decision-theoretic Bayesian design of experiments for such nonlinear models is conceptually very attractive, allowing the formal incorporation of prior knowledge to overcome the parameter dependence of frequentist design and being less reliant on asymptotic approximations. However, the necessary approximation and maximization of the, typically analytically intractable, expected utility results in a computationally challenging problem. These issues are further exacerbated if the solution to the differential equations is not available in closed-form. This article proposes a new combination of a probabilistic solution to the equations embedded within a Monte Carlo approximation to the expected utility with cyclic descent of a smooth approximation to find the optimal design. A novel precomputation algorithm reduces the computational burden, making the search for an optimal design feasible for bigger problems. The methods are demonstrated by finding new designs for a number of common models derived from differential equations, and by providing optimal designs for the placenta experiment. for this article, including a standardized description of the materials available for reproducing the work, are available as an online supplement.
更多查看译文
关键词
Approximate coordinate exchange algorithm,Decision-theoretic design,Gaussian process emulation,Nonlinear design
AI 理解论文
溯源树
样例
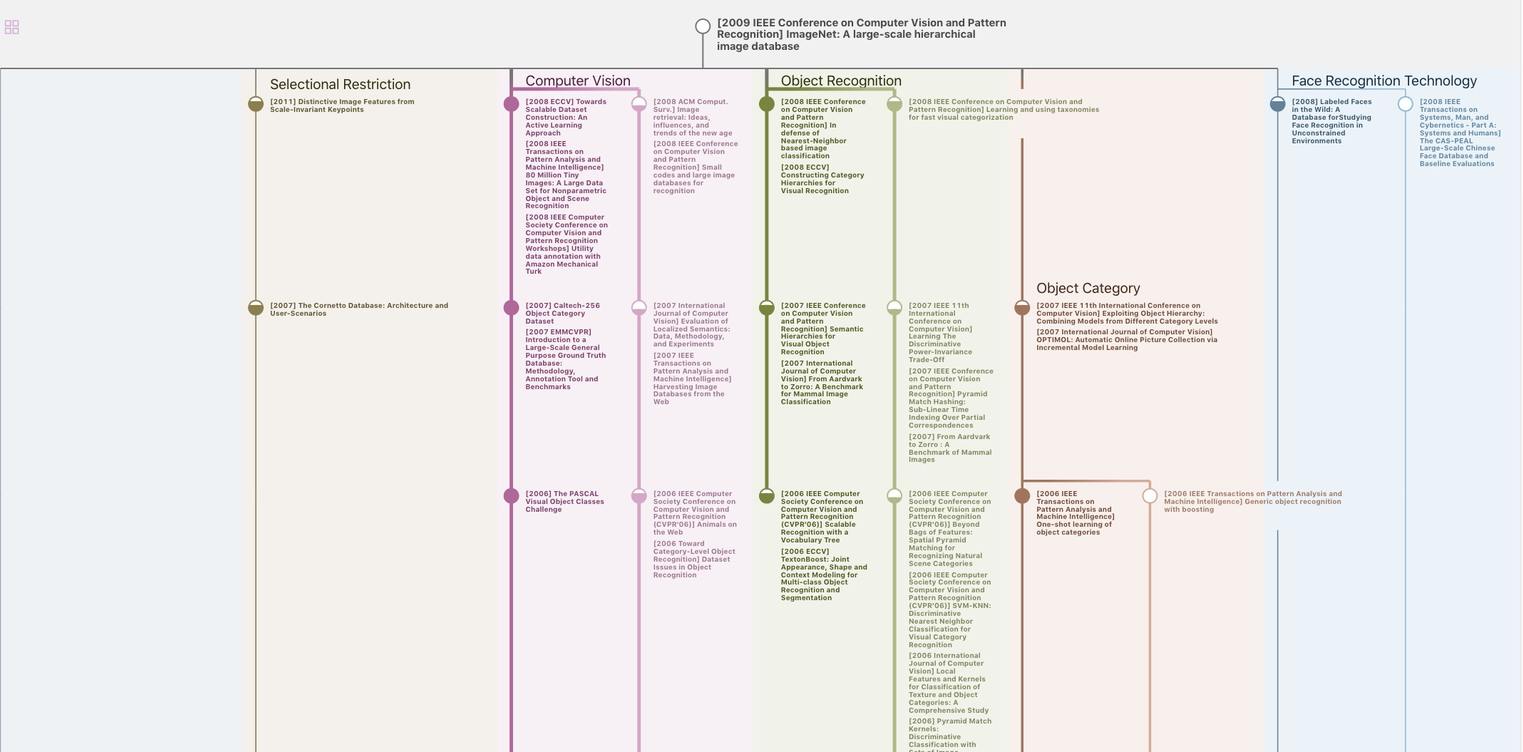
生成溯源树,研究论文发展脉络
Chat Paper
正在生成论文摘要