Participant-dependent and Participant-independent Classification of Emotions Using EEG Signals
2017 International Conference on Wireless Communications, Signal Processing and Networking (WiSPNET)(2017)
摘要
Emotion recognition has gained importance and significant attention as a part of the human-computer interface (HCI), and is envisioned to provide an "emotional dimension" to HCI. In this paper, we propose a novel method for participant-dependent and participant-independent emotion recognition using electroencephalogram (EEG) signals. We aim to distinguish between positive and negative valence emotions on the valencearousal graph, i.e., considering a binary classification problem. The EEG signals of DEAP dataset, where forty different videos were used to stimulate 32 participants is considered for the classification problem. Participant-dependent features are extracted in order to classify emotions for each participant, where a perfect classification score is shown to be achieved for one of the participants. Next, we consider generalization across participants and find participant-independent features. Applying the widely used or conventional method in this regard, we observe that the training time increases many fold with increase in participants. We propose a technique called averaging of features and show that the computational complexity of the proposed technique is significantly low as compared to the conventional method with increase in participants. The results obtained by detailed experiments and evaluation procedure indicate that averaging of features technique yields nearly the same performance as the conventional approach, but scarcely consumes computational time. The maximum classification accuracy for 32 participants was obtained to be 81.03% and 77.46% using conventional and averaging approaches respectively.
更多查看译文
关键词
emotion recognition,emotional dimension,positive valence emotions,negative valence emotions,participant-dependent features,participant-independent features,perfect classification score
AI 理解论文
溯源树
样例
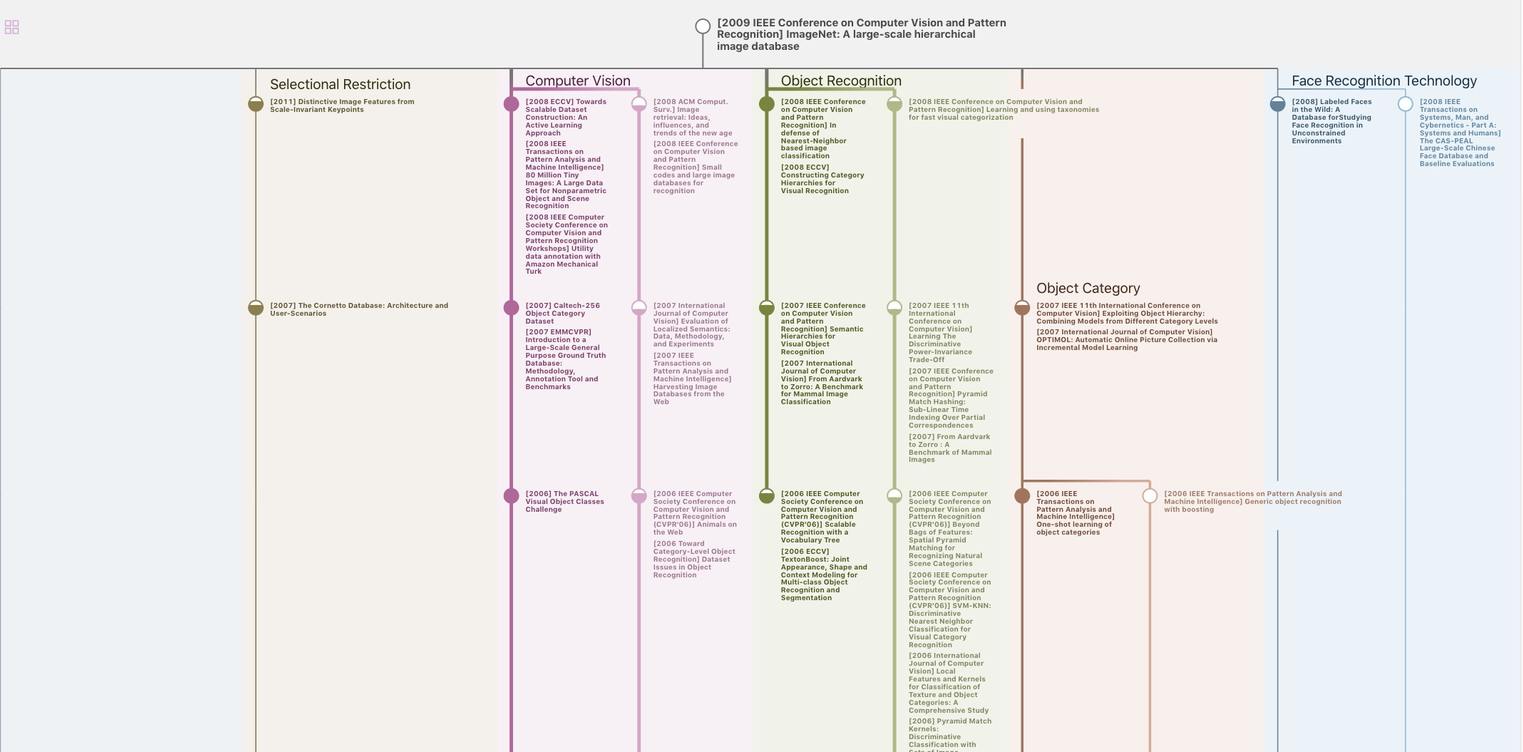
生成溯源树,研究论文发展脉络
Chat Paper
正在生成论文摘要