Detecting Seam-Carved Image by Extreme Learning Machines Using Patch Analysis Method, Jury Voting, and Combinatorial Fusion
IEEE Transactions on Systems, Man, and Cybernetics(2020)
摘要
Seam carving is a content-aware image processing approach that has been successfully applied to resizing or removing objects from digital images. Seam-carved images are hard to identify from the original image, making this detection method an important and attractive research topic. Existing methods are based on steganography attacks or statistical features, and their trained and tested models are all constructed using support vector machines (SVMs). The trained models of these methods take a long time to build from a limited number of images. This paper presents a new patch-based sobel operator (PSO) method using SVM and extreme learning machines (ELMs) based on the patch analysis method with square-based features. The ELM adopts five types of neurons to obtain five prediction results, which are then combined using the jury voting scheme in order to improve the accuracy. In addition, combinatorial fusion is first used to choose two of five prediction results according to the diversity rank/score graph, and these two results are then combined to make the final decision. The PSO method in the ELM with jury voting and combinatorial fusion achieves accuracies of 70.60–98.80% and 95.58–99.36% for 10–50% seam-carved and seam-insertion images, respectively. Additionally, for the PSO method, the ELM required only 1.2% of the training time required by the SVM. In conclusion, the PSO method in the ELM is useful for constructing trained models from a large number of images.
更多查看译文
关键词
Support vector machines,Feature extraction,Task analysis,Training,Testing,Neurons,Nonlinear distortion
AI 理解论文
溯源树
样例
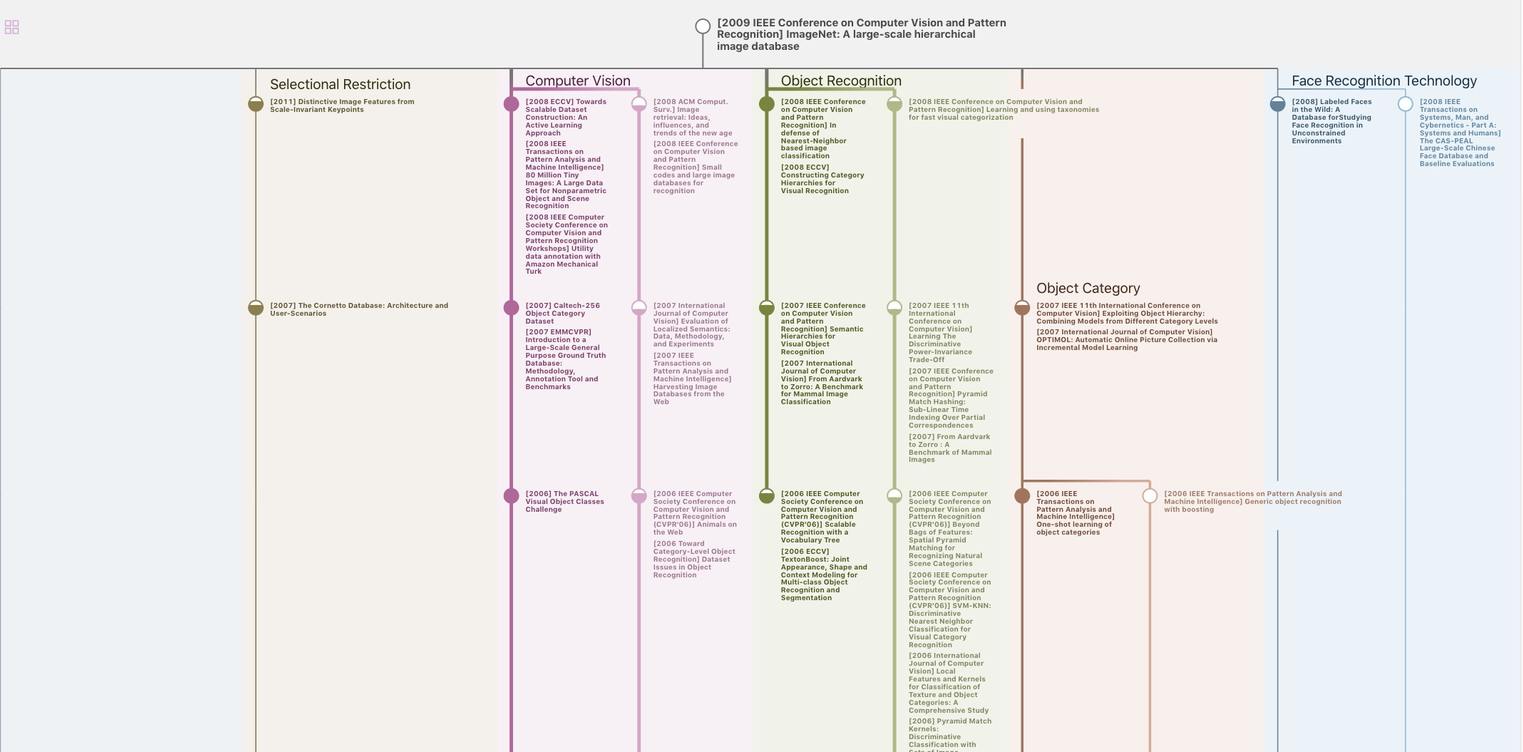
生成溯源树,研究论文发展脉络
Chat Paper
正在生成论文摘要