Representing Molecular and Materials Data for Unsupervised Machine Learning
Molecular simulation(2018)
摘要
Statistical analysis and machine learning can help us understand and predict the collective properties and performance of ensembles of molecules and nanostructures, while accounting for all the complexity and diversity of real world specimens. Combining data-driven techniques with robust and reliable simulation methods can provide insights that cannot be made any other way. However, not all statistical and machine learning methods are right for all occasions; testing, validation and perhaps some trial and error are needed. Domain knowledge alone is not sufficient to choose the right algorithms. Data representation methods that are best suited to machine learning are not necessarily scientifically intuitive. The best descriptors are not always the structural features or physiochemical properties that we are aiming to control, and the way our data is distributed can be as important as what it contains. In this review, we discuss the differences, advantages and disadvantages of some of the common data representation, reduction and classification methods applicable to molecular and materials modelling. Focussing on unsupervised methods, we highlight features of these algorithms that determine their suitability and can inform choices of which learning method to use and how to effectively prepare data. A case study is also provided to demonstrate how testing can be undertaken, and how methods can be combined.
更多查看译文
关键词
Machine learning,informatics,clustering,descriptors,screening
AI 理解论文
溯源树
样例
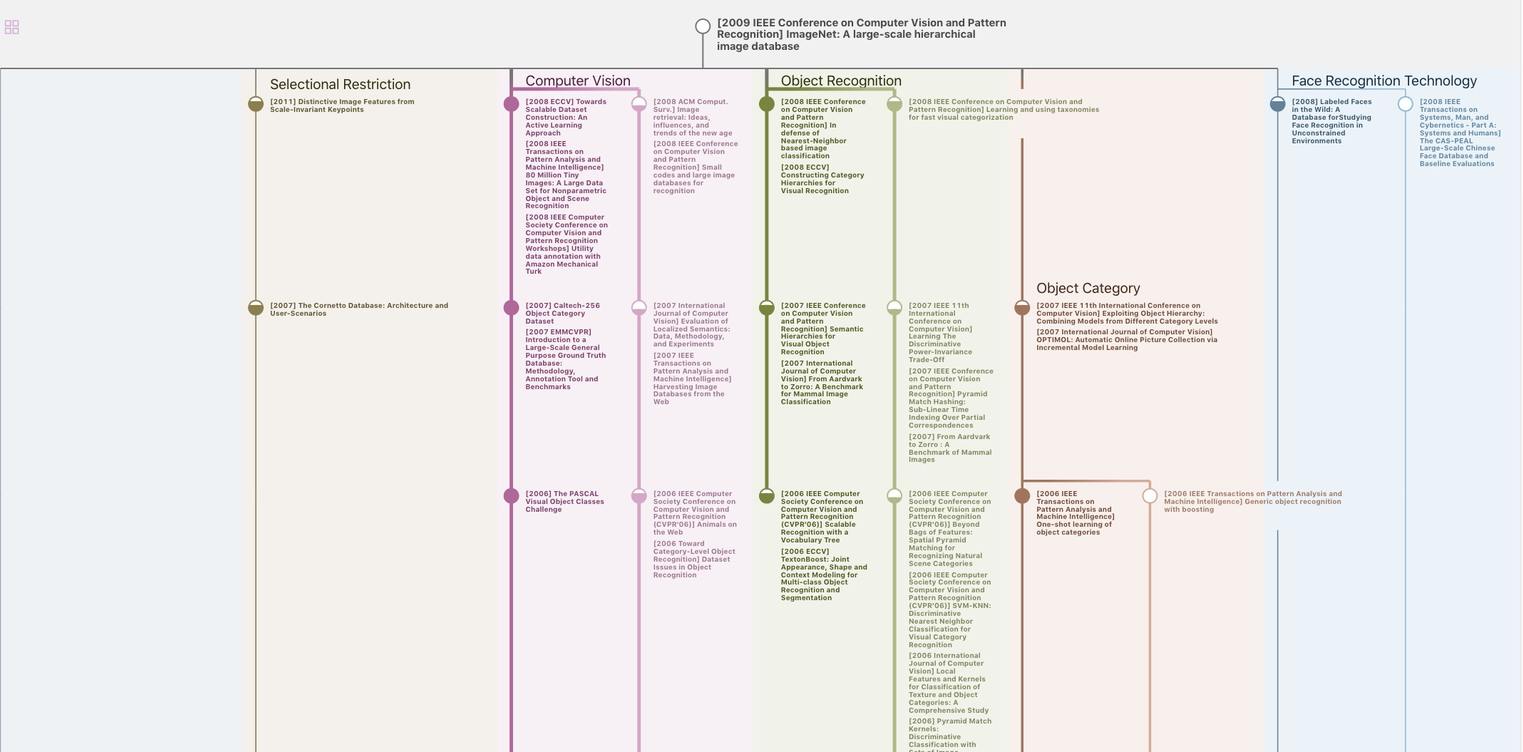
生成溯源树,研究论文发展脉络
Chat Paper
正在生成论文摘要