Variational Recursive Dual Filtering
arXiv: Machine Learning(2017)
摘要
State space models provide an interpretable framework for complex time series by combining an intuitive dynamical system model with a probabilistic observation model. We developed a flexible online learning framework for latent nonlinear state dynamics and filtered latent states. Our method utilizes the stochastic gradient variational Bayes method to jointly optimize the parameters of the nonlinear dynamics, observation model, and the black-box recognition model. Unlike previous approaches, our framework can incorporate non-trivial observation noise models and infer in real-time. We test our method on point process observations driven by continuous attractor dynamics, demonstrating its ability to recover the phase portrait, filtered trajectory, and produce long-term predictions for real-time machine learning.
更多查看译文
AI 理解论文
溯源树
样例
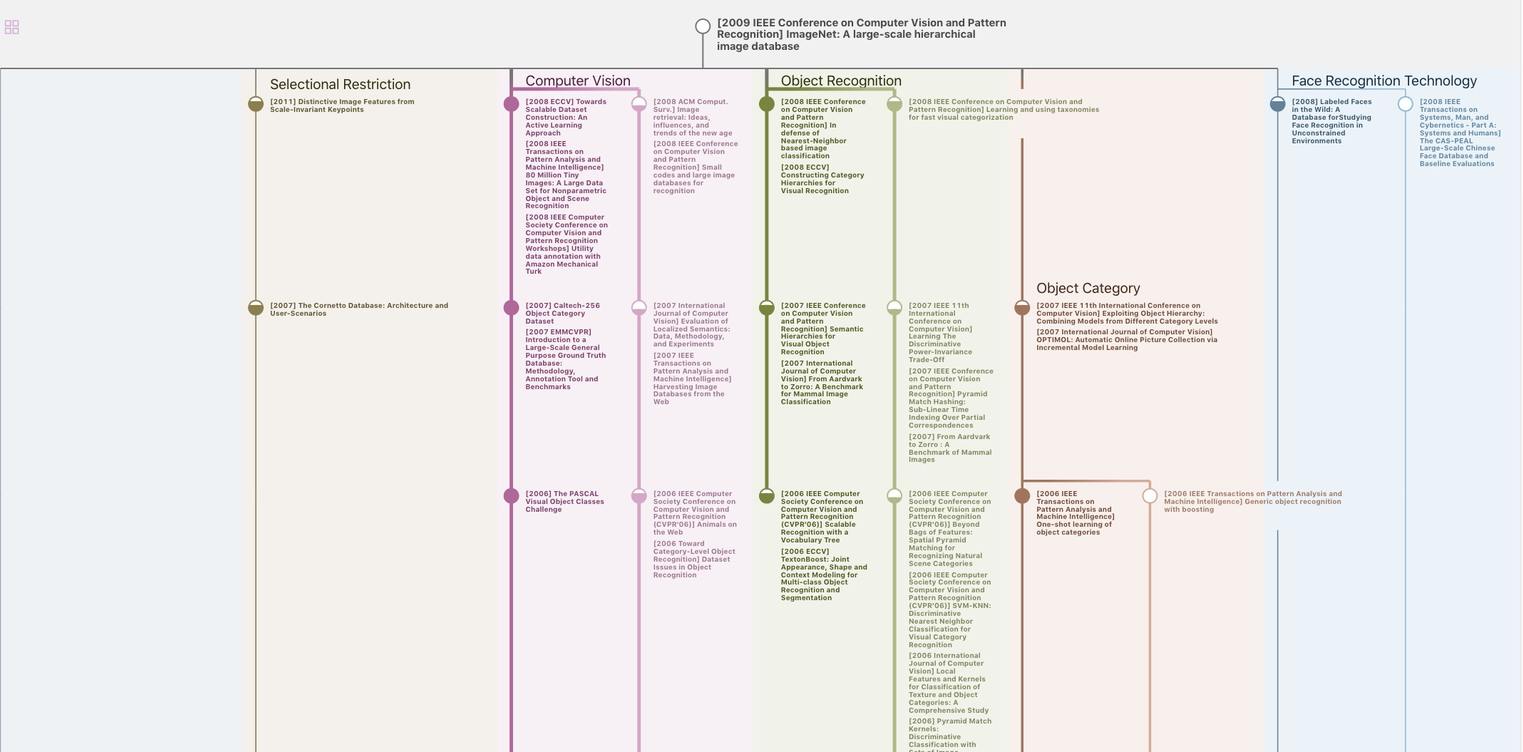
生成溯源树,研究论文发展脉络
Chat Paper
正在生成论文摘要