The Block-Poisson Estimator for Optimally Tuned Exact Subsampling MCMC
JOURNAL OF COMPUTATIONAL AND GRAPHICAL STATISTICS(2021)
摘要
Speeding upMarkov chainMonte Carlo (MCMC) for datasets withmany observations by data subsampling has recently received considerable attention. A pseudo-marginalMCMCmethod is proposed that estimates the likelihood by data subsampling using a block-Poisson estimator. The estimator is a product of Poisson estimators, allowing us to update a single block of subsample indicators in each MCMC iteration so that a desired correlation is achieved between the logs of successive likelihood estimates. This is important since pseudo-marginal MCMC with positively correlated likelihood estimates can use substantially smaller subsamples without adversely affecting the sampling efficiency. The block-Poisson estimator is unbiased but not necessarily positive, so the algorithm runs the MCMC on the absolute value of the likelihood estimator and uses an importance sampling correction to obtain consistent estimates of the posterior mean of any function of the parameters. Our article derives guidelines to select the optimal tuning parameters for our method and shows that it compares very favorably to regular MCMC without subsampling, and to two other recently proposed exact subsampling approaches in the literature. Supplementary materials for this article are available online.
更多查看译文
关键词
Bayesian inference, Control variates, Data subsampling, Exact inference, Poisson estimator, Pseudo-marginal MCMC
AI 理解论文
溯源树
样例
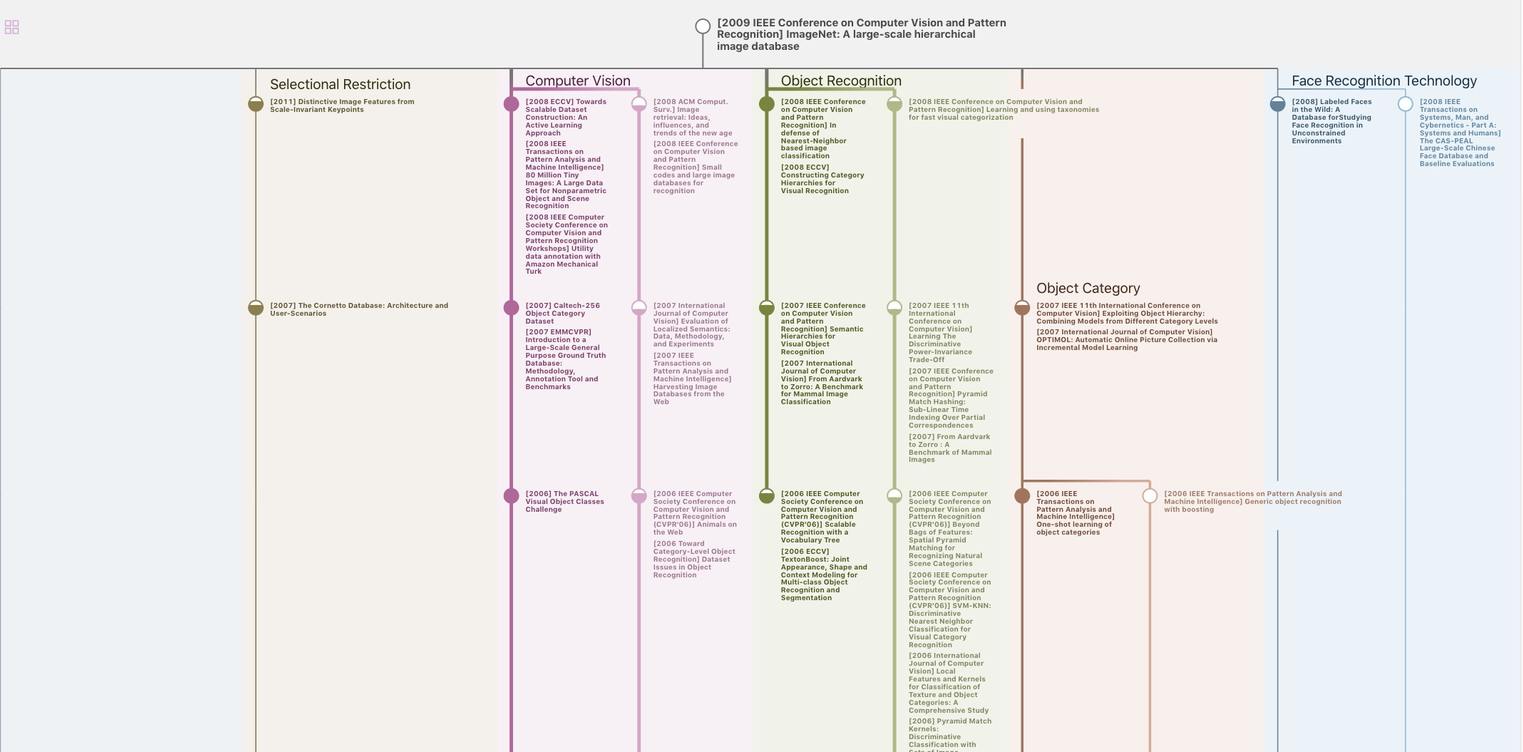
生成溯源树,研究论文发展脉络
Chat Paper
正在生成论文摘要