Relaxed support vector regression
Annals of Operations Research(2018)
摘要
Datasets with outliers pose a serious challenge in regression analysis. In this paper, a new regression method called relaxed support vector regression (RSVR) is proposed for such datasets. RSVR is based on the concept of constraint relaxation which leads to increased robustness in datasets with outliers. RSVR is formulated using both linear and quadratic loss functions. Numerical experiments on benchmark datasets and computational comparisons with other popular regression methods depict the behavior of our proposed method. RSVR achieves better overall performance than support vector regression (SVR) in measures such as RMSE and R^2_adj while being on par with other state-of-the-art regression methods such as robust regression (RR) . Additionally, RSVR provides robustness for higher dimensional datasets which is a limitation of RR, the robust equivalent of ordinary least squares regression. Moreover, RSVR can be used on datasets that contain varying levels of noise.
更多查看译文
关键词
Regression, Relaxed support vector regression, Outliers, Relaxed support vector machines, Support vector regression
AI 理解论文
溯源树
样例
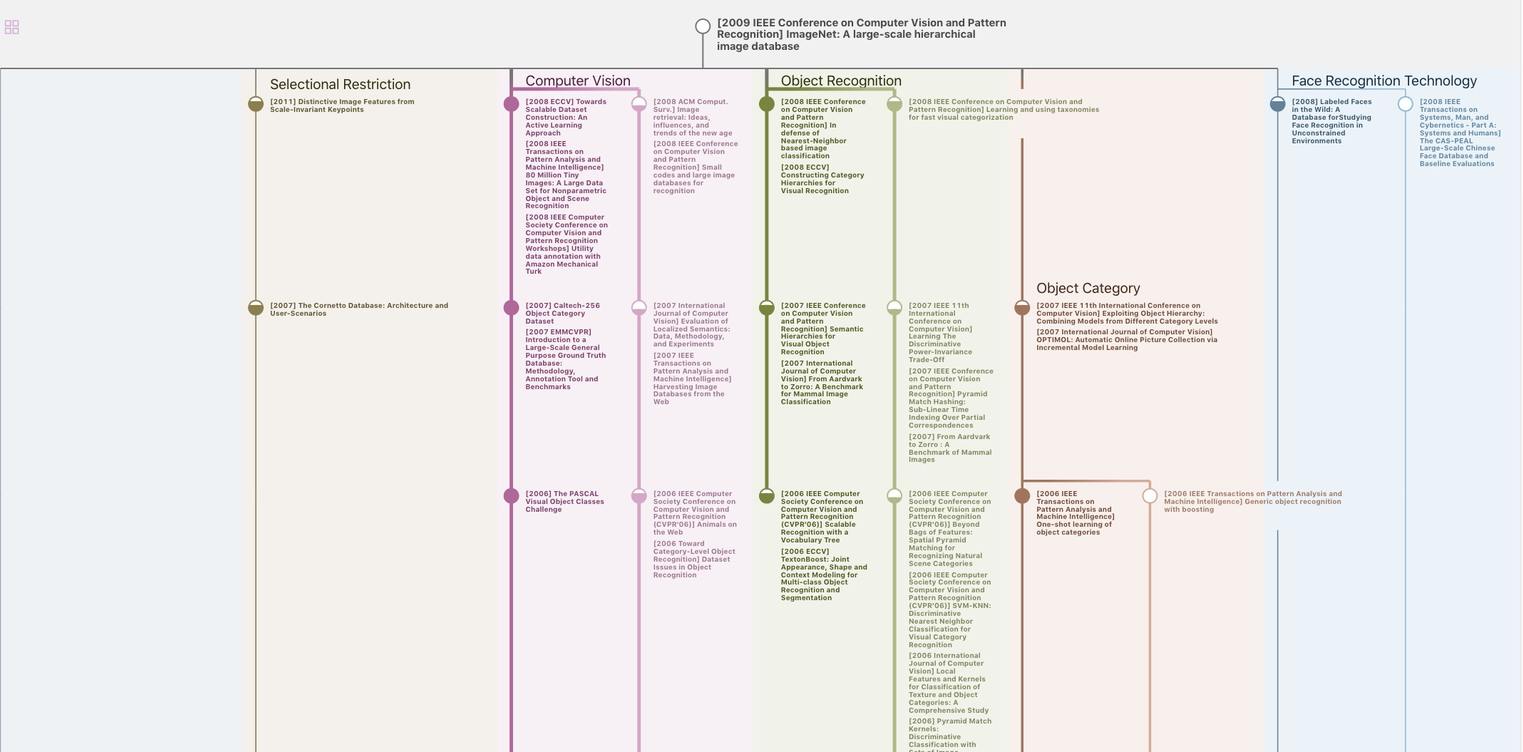
生成溯源树,研究论文发展脉络
Chat Paper
正在生成论文摘要