Pedestrian Detection Using Improved Proposal Boxes And Grayscale Convolutional Learning
2017 10TH IRANIAN CONFERENCE ON MACHINE VISION AND IMAGE PROCESSING (MVIP)(2017)
摘要
In this paper, a new method is presented to improve pedestrian detection accuracy in color images. A pedestrian detection system includes two main parts: proposal generator and accurate classifier. In this paper, LDCF detector and a convolutional neural network based on VGG19 are used for these parts. The first novelty of this paper is to use multiple new proposal boxes around LDCF proposals in the first part of the system leading to more proper inputs for the convolutional network. The second novelty of this paper is discussing the redundancy of color information in images used to train neural network of the second part of the system in the case of pedestrian detection. It is shown that using gray images results in more convergence and better training of the network. This paper achieves the result of 15.86% Miss Rate on 0.1 FPPI on Caltech pedestrian detection dataset that is comparable to other well-known methods in pedestrian detection.
更多查看译文
关键词
pedestrian detection, proposed bounding boxes, deep neural network, deep learning
AI 理解论文
溯源树
样例
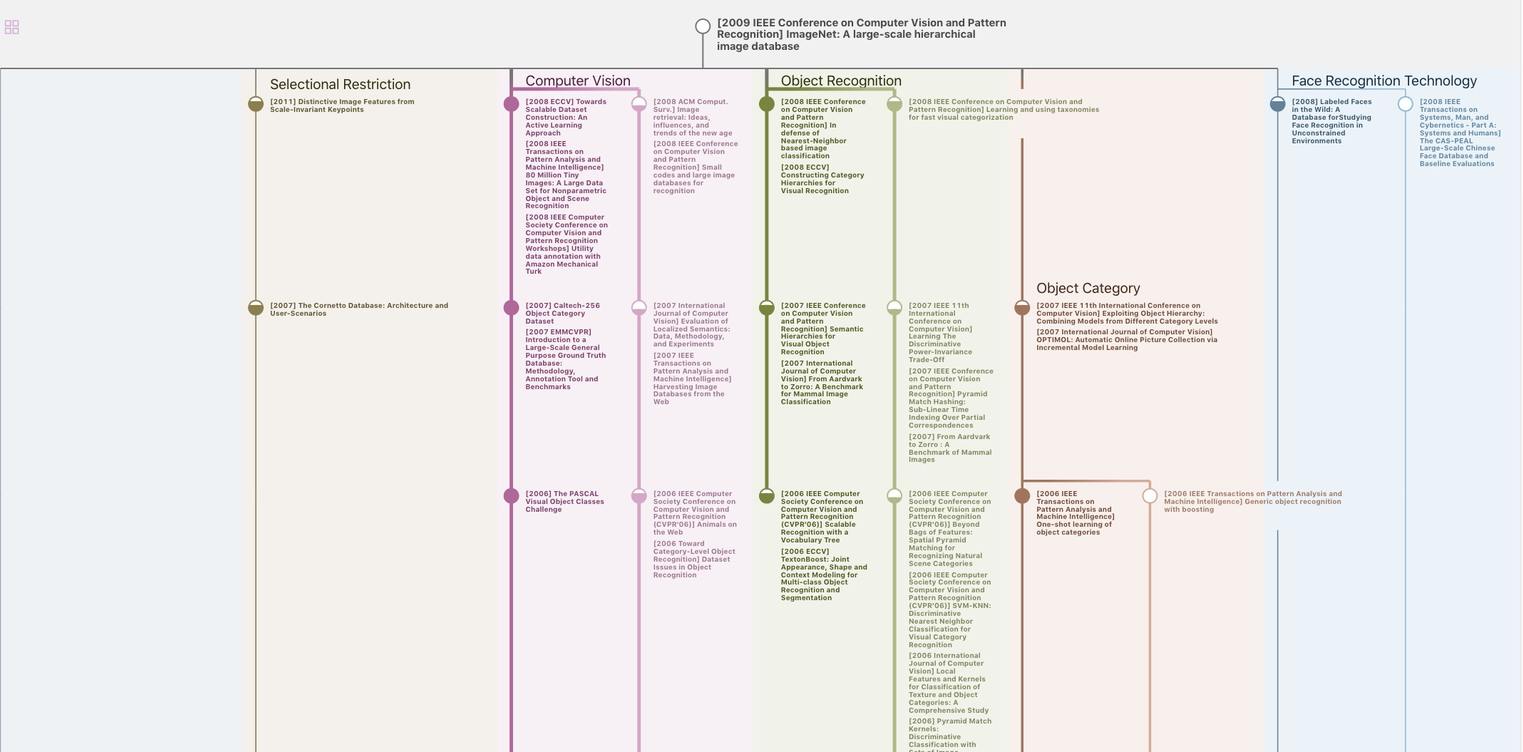
生成溯源树,研究论文发展脉络
Chat Paper
正在生成论文摘要