On the Feasibility of Natural Language Processing for Standardized Data Extraction from Electronic Medical Records of Epilepsy Patients
Neurology(2018)
摘要
Objective: To assess the validity of Natural Language Processing (NLP) of electronic medical records (EMR). Background: NLP is the process by which an algorithm can extract information from the natural language of humans. While human readers can readily infer meaning from culturally specific natural language, human review is resource-limited and inefficient for large EMR datasets. Design/Methods: An NLP algorithm was created to identify and extract a series of high-yield variables specific to epilepsy research from patients’ EMRs. The algorithm searched both structured fields and free text, including documents such as radiology reports, clinician notes, and EEG reports. It is specific to epilepsy-associated phenotypes and was developed in parallel with human data abstraction on a training dataset. To assess validity, we compared the NLP-extracted phenotypes with human-extracted phenotypes (agreed upon by two reviewers) for 100 independent samples. Results: NLP was least sensitive for variables that required assessment of the clinicians’ thought process, including variables such as type of MRI lesion, epilepsy syndrome, and clinicians’ indication for ordering long-term EEG (e.g. differential diagnosis, classification, quantification, pre-surgical evaluation). NLP was reasonably sensitive for most other variables such as EEG abnormality, identification of psychogenic non-epileptic spells (PNES) on EEG, current anti-epileptic drugs (AEDs), prior AEDs, and AED allergies. Specificity was relatively high for all variables. Cost analysis revealed that for most variables, the total time cost required by NLP drops below that of human reviewers when 300 or more charts are reviewed, with multi-fold improvement in performance when 1,000s of charts are reviewed. Conclusions: A gradient of phenotype-related variables can be assessed through NLP. Medications and structured information can be extracted with relative ease. Extracting information from free-text documents is less accurate because it requires insight into a clinical thought process. With larger study sizes in genetic studies and precision medicine trials, NLP will be essential for epilepsy phenotyping. Disclosure: Dr. Khankhanian has nothing to disclose. Dr. Kosaraju has nothing to disclose. Dr. Pathmanathan has nothing to disclose. Dr. Ellis has nothing to disclose. Dr. Helbig has nothing to disclose. Dr. Litt has nothing to disclose. Dr. Pollard has received compensation for serving on the Board of Directors of Cognizance Biomarkers. Dr. Davis has nothing to disclose.
更多查看译文
AI 理解论文
溯源树
样例
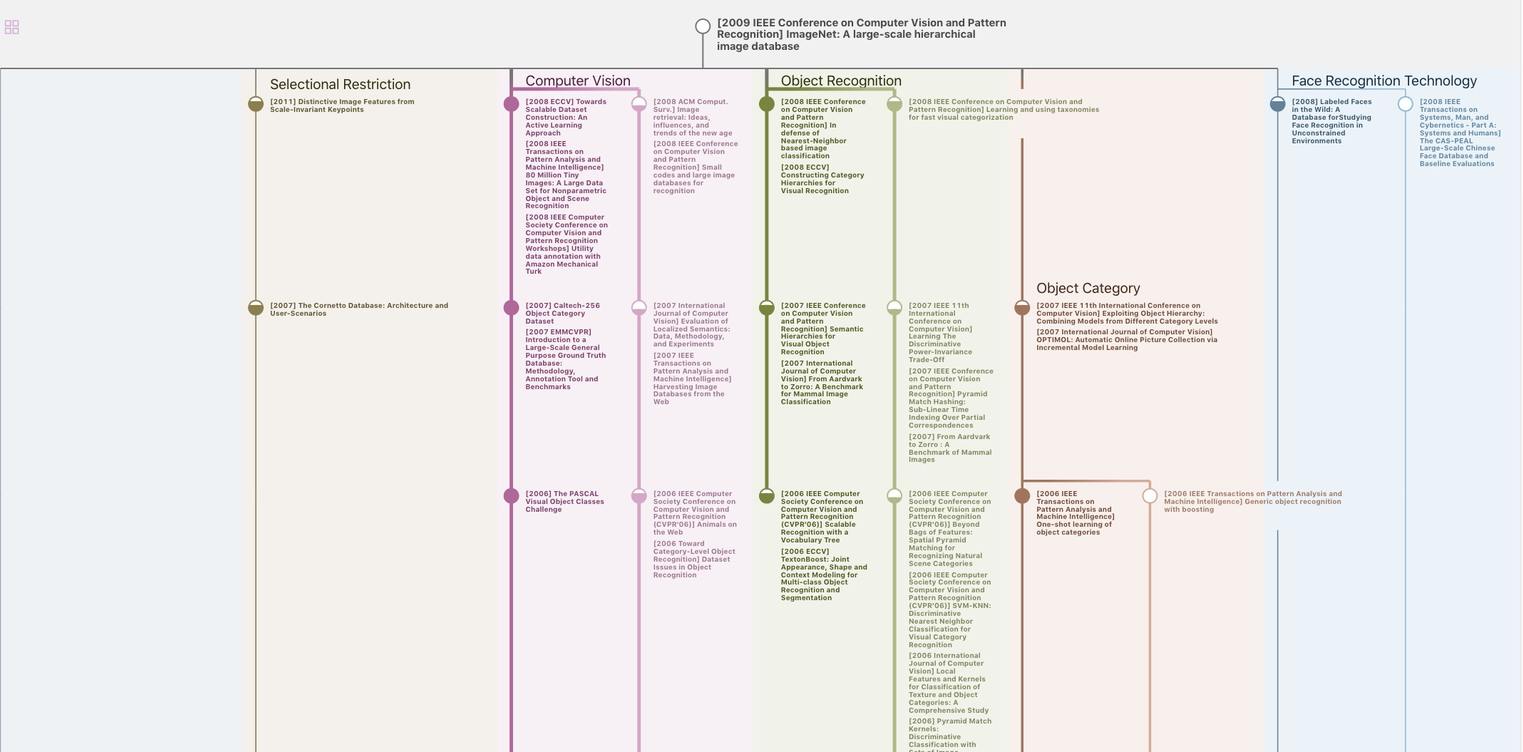
生成溯源树,研究论文发展脉络
Chat Paper
正在生成论文摘要