Machine-learning techniques for fast and accurate holographic particle tracking
arXiv: Soft Condensed Matter(2018)
摘要
Quantitative analysis of holographic microscopy images yields thethree-dimensional positions of micrometer-scale colloidal particleswith nanometer precision, while simultaneously measuring theparticlesu0027 sizes and refractive indexes. Extracting this informationbegins by detecting and localizing features of interest withinindividual holograms. Conventionally approached with heuristicalgorithms, this image analysis problem can be solved faster and moregenerally with machine-learning techniques. We demonstrate that twopopular machine-learning algorithms, cascade classifiers and deepconvolutional neural networks (CNN), can solve the particle-trackingproblem orders of magnitude faster than current state-of-the-arttechniques. Our CNN implementation localizes holographic featuresprecisely enough to bootstrap more detailed analyses based on the Lorenz-Mie theory of light scattering. The wavelet-based Haar cascadeperforms less well at detecting and localizing particles, but is socomputationally efficient that it creates new opportunities for applicationsthat emphasize speed and low cost. We demonstrate its use as a real-time targeting system for holographic optical trapping.
更多查看译文
AI 理解论文
溯源树
样例
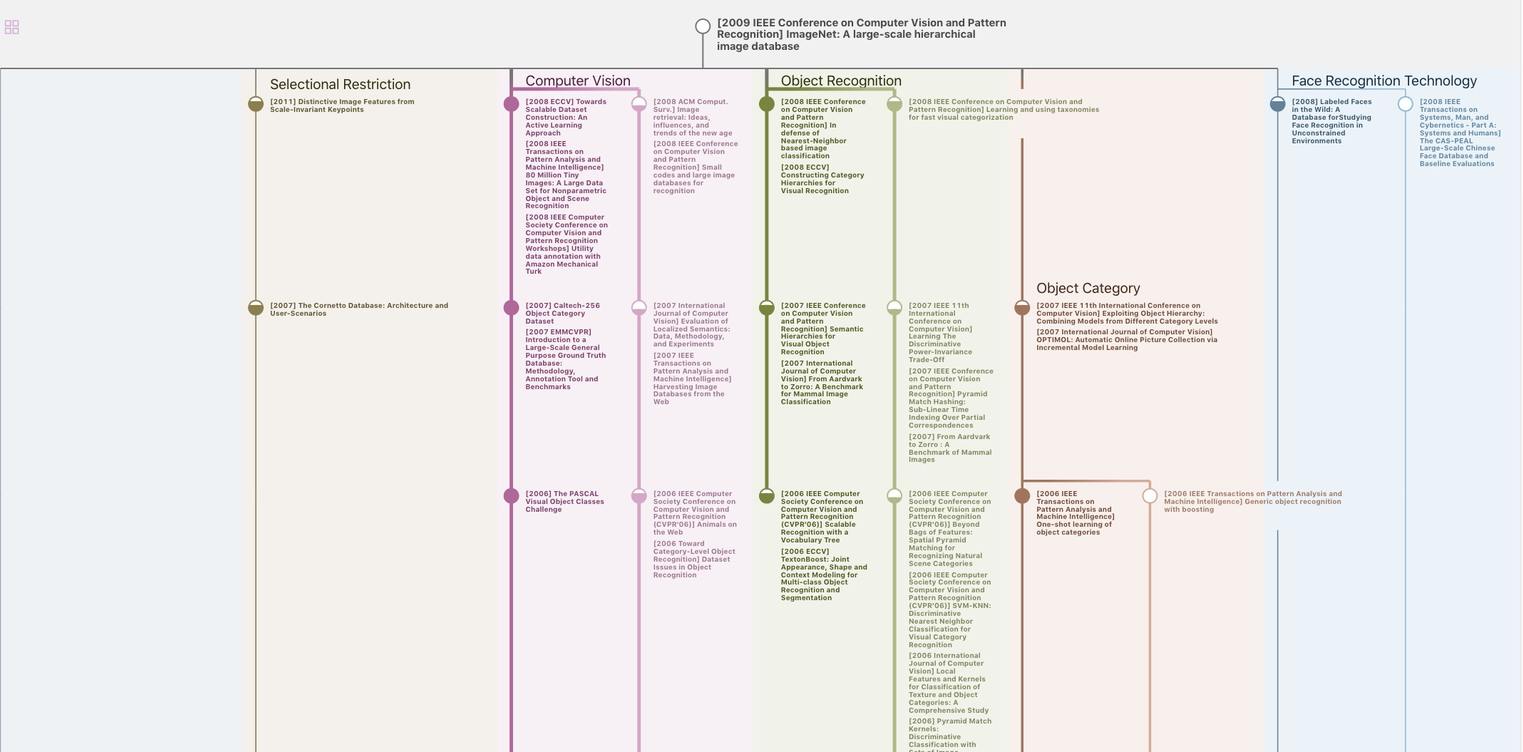
生成溯源树,研究论文发展脉络
Chat Paper
正在生成论文摘要