Spatial detrending revisited: Modelling local trend patterns in NO2-concentration in Belgium and Germany
Spatial Statistics(2018)
摘要
Short-term predictions of air pollution require spatial modelling of trends, heterogeneities, and dependencies. Two-step methods allow real-time computations by separating spatial detrending and spatial extrapolation into two steps. Existing methods discuss trend models for specific environments and require specification search. Given more complex environments, specification search gets complicated by potential nonlinearities and heterogeneities. This research embeds a nonparametric trend modelling approach in real-time two-step methods. Form and complexity of trends are allowed to vary across heterogeneous environments. The proposed method avoids ad hoc specifications and potential generated predictor problems in previous contributions. Examining Belgian and German air quality and land use data, local trend patterns are investigated in a data driven way and are compared to results computed with existing methods and variations thereof. An important aspect of our empirical illustration is the heterogeneity and superior performance of local trend patterns for both research regions. The findings suggest that a nonparametric spatial trend modelling approach is a valuable tool for real-time predictions of pollution variables: it avoids specification search, provides useful exploratory insights and reduces computational costs.
更多查看译文
关键词
Stationarity,RIO model,Air pollution,Land use,Nonparametrics
AI 理解论文
溯源树
样例
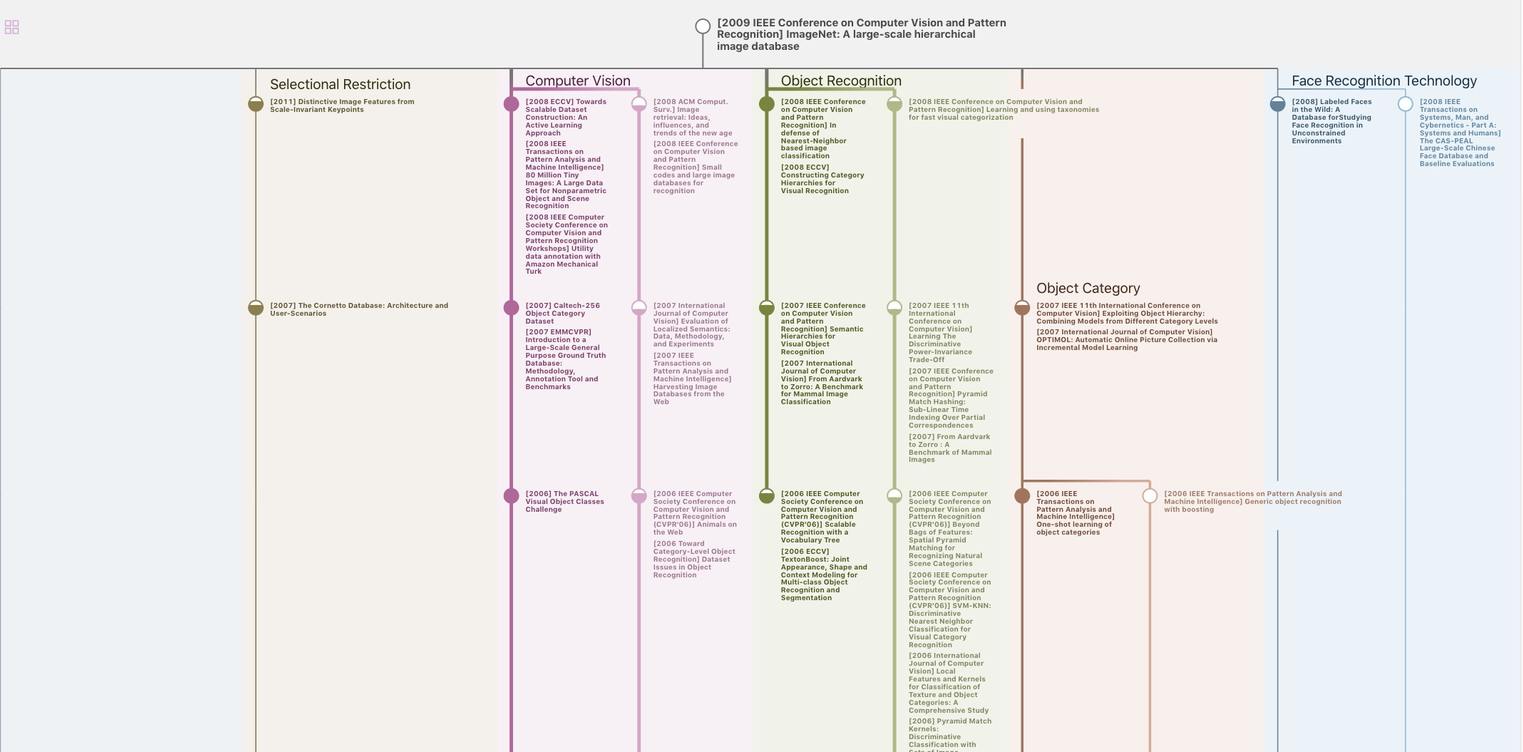
生成溯源树,研究论文发展脉络
Chat Paper
正在生成论文摘要