An adaptive learning control strategy for standalone PV system with battery-supercapacitor hybrid energy storage system
Journal of Power Sources(2018)
摘要
Contrary to Rule-based Controller and Fuzzy Logic Controller, which are usually restricted by pre-defined rules, the proposed control strategy implements power distribution algorithm to offer higher flexibility to the Battery-Supercapacitor Hybrid Energy Storage System by introducing a charging threshold and a discharging threshold. It allows the Hybrid Energy Storage System to be charge/discharged without constraints. The proposed optimization method, which comprises Self-Organizing Map and Particle Swarm Optimization, optimizes the parameters of power distribution algorithm every minute based on predicted one-hour power demand and supercapacitor state-of-charge to mitigate peak demand and short charge-discharge cycles of battery. The proposed optimization method initializes the initial population of particles within the range of optimal solution instead of randomizing the particles like the conventional method. This method offers faster convergence and reduces the optimization time up to 68.97% as compared to conventional method. The simulation results show the proposed control strategy can compensate the prediction error of prediction model and outperform the Filtration-based Controller and Particle Swarm Optimization-optimized Fuzzy Logic Controller in different scenarios. Moreover, it significantly increases the supercapacitor utilization by 7.33 times and reduces the mean absolute rate of change of battery power and battery peak demand up to 91.94% and 61.36%, respectively.
更多查看译文
关键词
Supercapacitor,Control strategy,Battery,Hybrid energy storage system,Particle swarm optimization,Self-Organizing Map
AI 理解论文
溯源树
样例
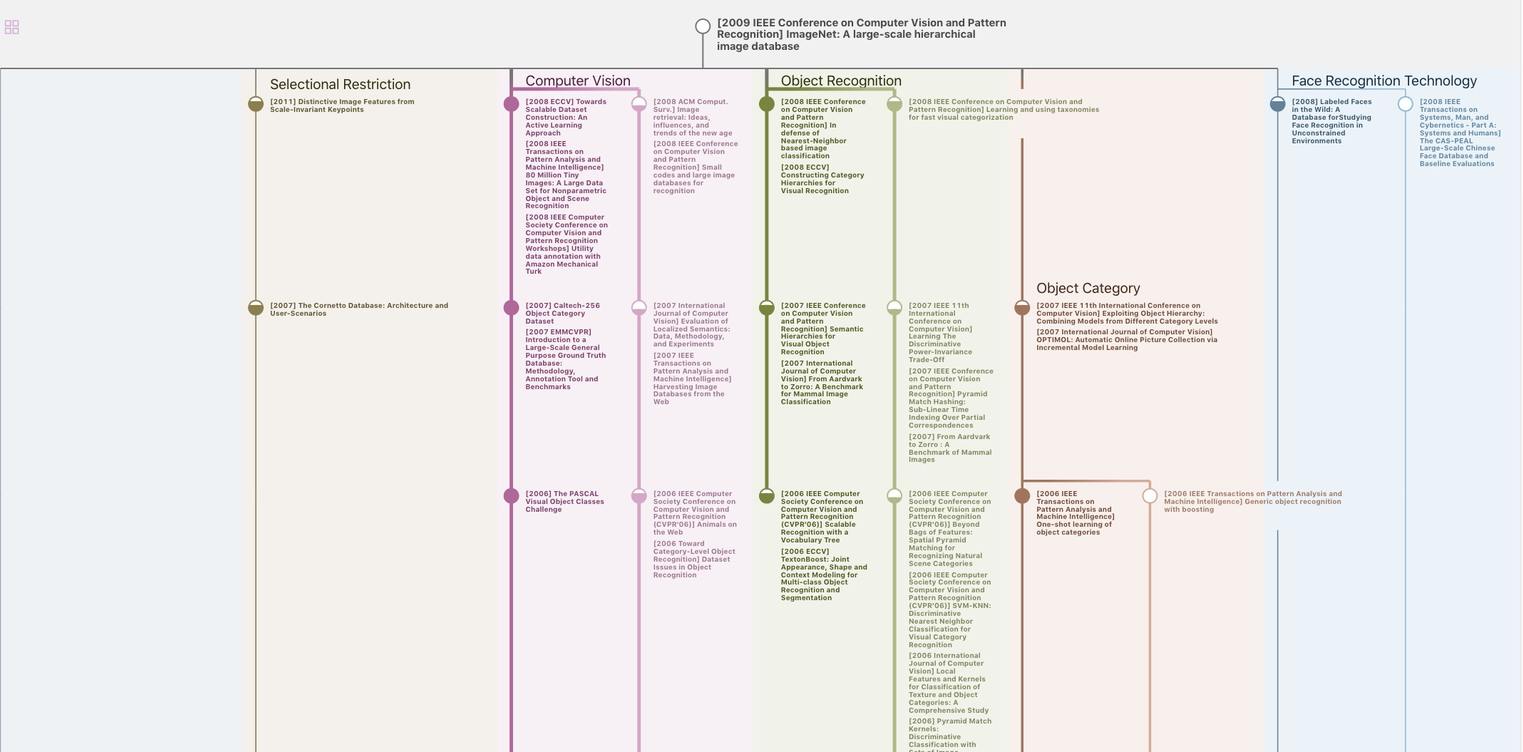
生成溯源树,研究论文发展脉络
Chat Paper
正在生成论文摘要