Extremal distributions under approximate majorization
JOURNAL OF PHYSICS A-MATHEMATICAL AND THEORETICAL(2018)
摘要
Although an input distribution may not majorize a target distribution, it may majorize a distribution which is close to the target. Here we consider a notion of approximate majorization. For any distribution, and given a distance delta, we find the approximate distributions which majorize (are majorized by) all other distributions within the distance delta. We call these the steepest and flattest approximation. This enables one to compute how close one can get to a given target distribution under a process governed by majorization. We show that the flattest and steepest approximations preserve ordering under majorization. Furthermore, we give a notion of majorization distance. This has applications ranging from thermodynamics, entanglement theory, and economics.
更多查看译文
关键词
majorization,state optimisation,approximate transformations
AI 理解论文
溯源树
样例
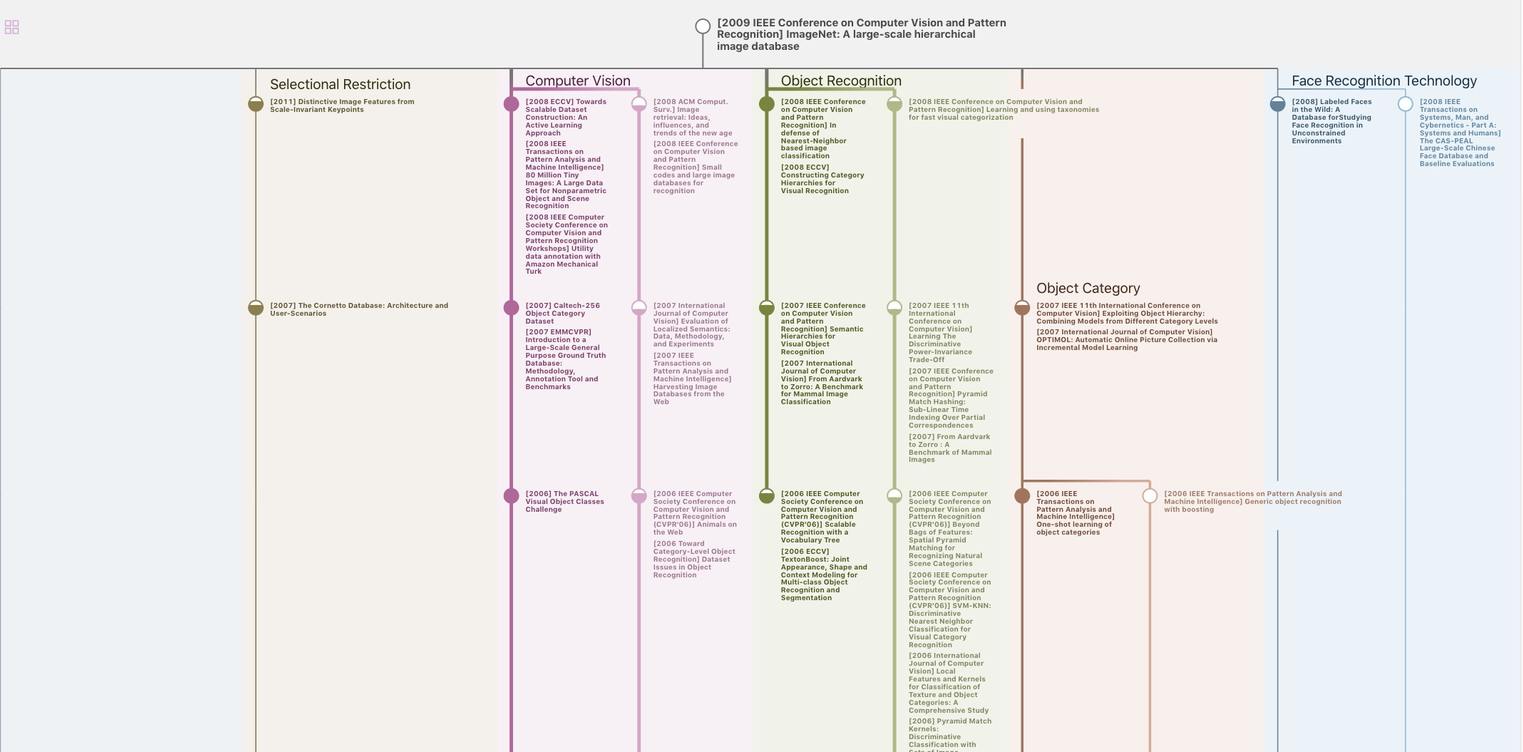
生成溯源树,研究论文发展脉络
Chat Paper
正在生成论文摘要