Land cover classification analysis of volcanic island in Aleutian Arc using an artificial neural network (ANN) and a support vector machine (SVM) from Landsat imagery
Geosciences Journal(2018)
摘要
Land cover (LC) mapping is an important research topic with many applications in remote sensing. Especially, for volcanic areas where direct field access is difficult, remote sensing data are needed to map LC. Volcanic areas are attractive targets for LC mapping because any spread of volcanic eruptions must be monitored. When creating LC maps, it is important to minimize errors because such errors compromise analyses using these maps. Here, we analyzed multispectral data from Mount Kanaga, Mount Fourpeaked, Mount Pavlof, and Mount Augustine using two different classifiers, an artificial neural network (ANN) and a support vector machine (SVM). To this end, we employed Landsat 8 imagery, which features four LC classes: outcrops (pyroclastic deposits, volcanic rock, sand, etc.), vegetation, water bodies, and snow. We found that the SVM was more accurate than the ANN. For Mount Kanaga, the SVM afforded the best classification accuracy (98.08%), 9.14% better than the ANN (88.94%); for the other volcanoes, the accuracy of the two methods did not differ significantly. Overall, both classifiers accurately distinguished products of volcanic eruption (outcrops) from other LC. Thus, both the ANN and SVM can be used for LC classification.
更多查看译文
关键词
land cover classification,Landsat imagery,Artificial Neural Network,Support Vector Machine
AI 理解论文
溯源树
样例
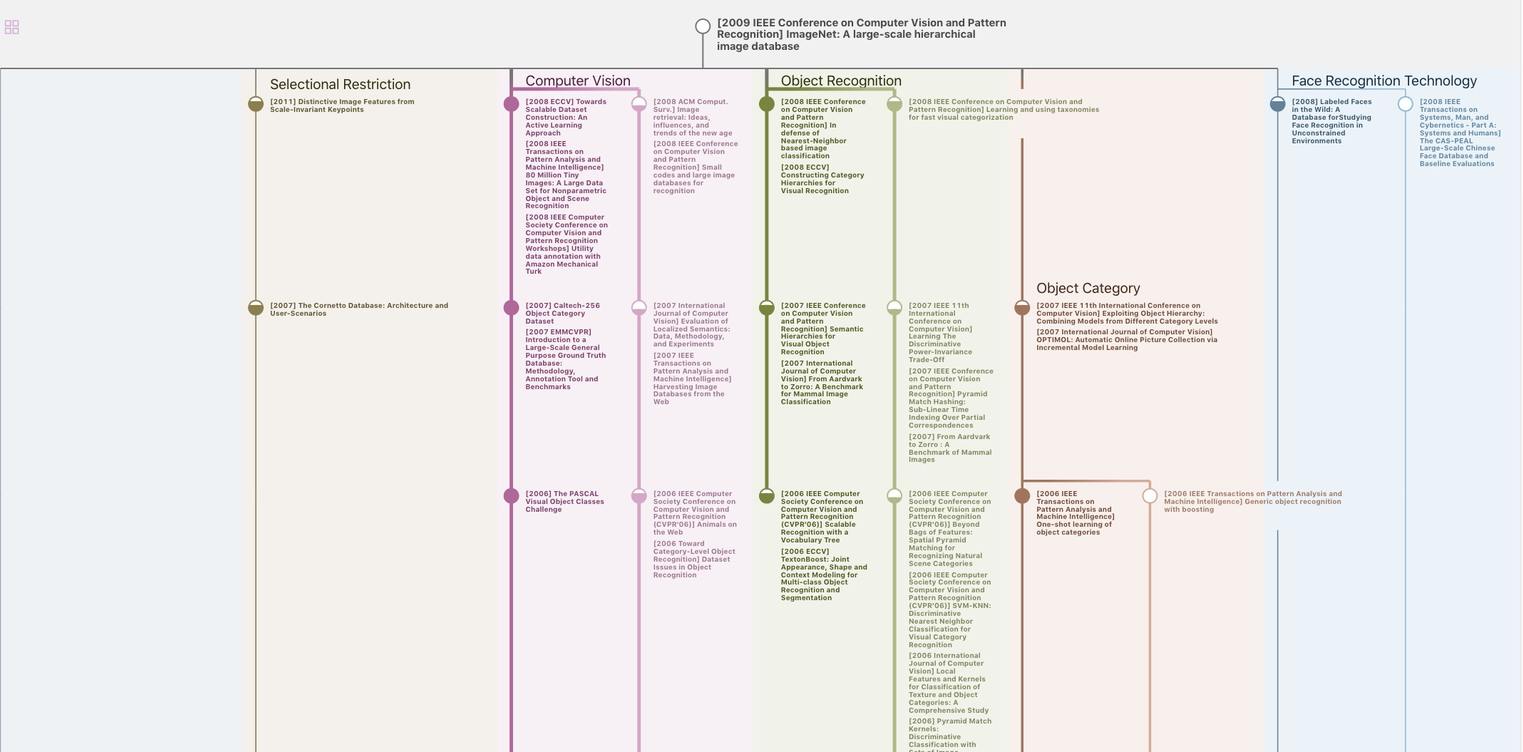
生成溯源树,研究论文发展脉络
Chat Paper
正在生成论文摘要