Constrained User Exposure Matrix Factorization In Recommendation System
INTERNATIONAL CONFERENCE ON MECHANICAL, ELECTRONIC AND INFORMATION TECHNOLOGY (ICMEIT 2018)(2018)
摘要
Due to the massive items and users limited scope, many existing recommendation systems suffer a more and more serious sparse problem on rating matrix. Most of the recommendation systems employ the content-based method or the collaborative filter model to abbreviate the sparse problem. While in our paper, based on the assumption that users who have rated similar sets of items are likely to have similar preference information, we present a constrained user exposure matrix factorization collaborative filter model and apply it to deal with the matrix sparsity problem, which it makes a better recommendation to users who have very few ratings. In detail, for the sake of capturing the effect of user having rated a particular item, we introduce a constrained matrix to user latent vector space. This effect will have an impact on the prior mean of user latent feature vector. After that, using the user feature vector and item feature vector to estimate the values of unrated items. The experimental results show that the proposed model gets higher prediction precision than the state-of-the-art models in providing recommendation for user having very few ratings.
更多查看译文
关键词
Recommendation system, Constrained matrix, Matrix factorization
AI 理解论文
溯源树
样例
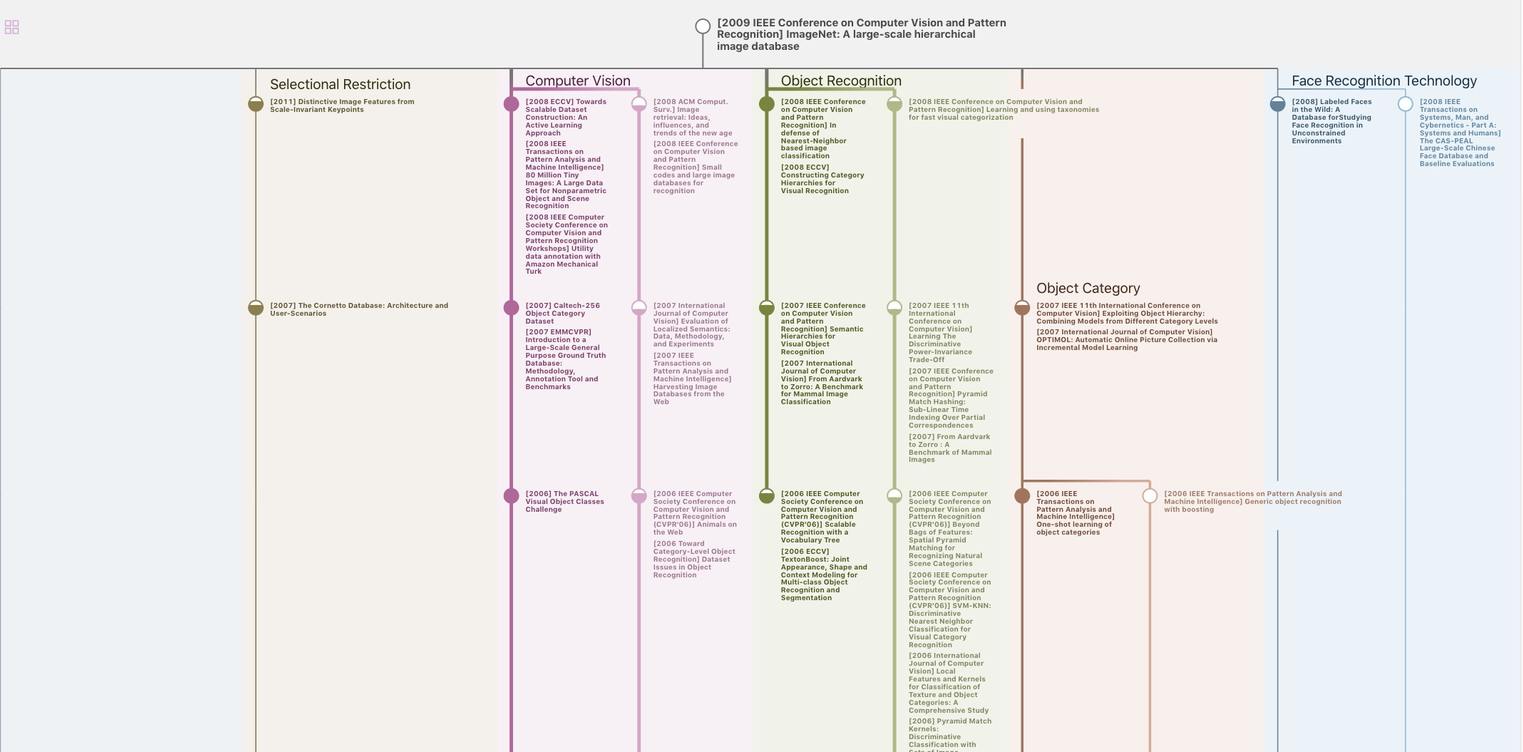
生成溯源树,研究论文发展脉络
Chat Paper
正在生成论文摘要