Hybridized Support Vector Machine and Recursive Feature Elimination with Information Complexity
Statistics, Optimization and Information Computing(2018)
摘要
In statistical data mining research, datasets often have nonlinearity and at the same time high-dimensionality. It has become difficult to analyze such datasets in a comprehensive manner using traditional statistical methodologies. In this paper, a novel wrapper method called SVM-ICOMP-RFE based on a hybridized support vector machine (SVM) and recursive feature elimination (RFE) with information-theoretic measure of complexity (ICOMP) is introduced and developed to classify high-dimensional data sets and to carry out subset selection of the features in the original data space for finding the best subset of features which are discriminating between the groups. Recursive feature elimination (RFE) ranks features based on information complexity (ICOMP) criterion. ICOMP plays an important role not only in choosing an optimal kernel function from a portfolio of many other kernel functions, but also in selecting important subset(s) of features. The potential and the flexibility of our approach are illustrated on two real benchmark data sets, one is ionosphere data which includes radar returns from the ionosphere, and another is aorta data which is used for the early detection of atheroma most commonly resulting heart attack. Also, the proposed method is compared with other RFE based methods using different measures (i.e., weight and gradient) for feature rankings.
更多查看译文
AI 理解论文
溯源树
样例
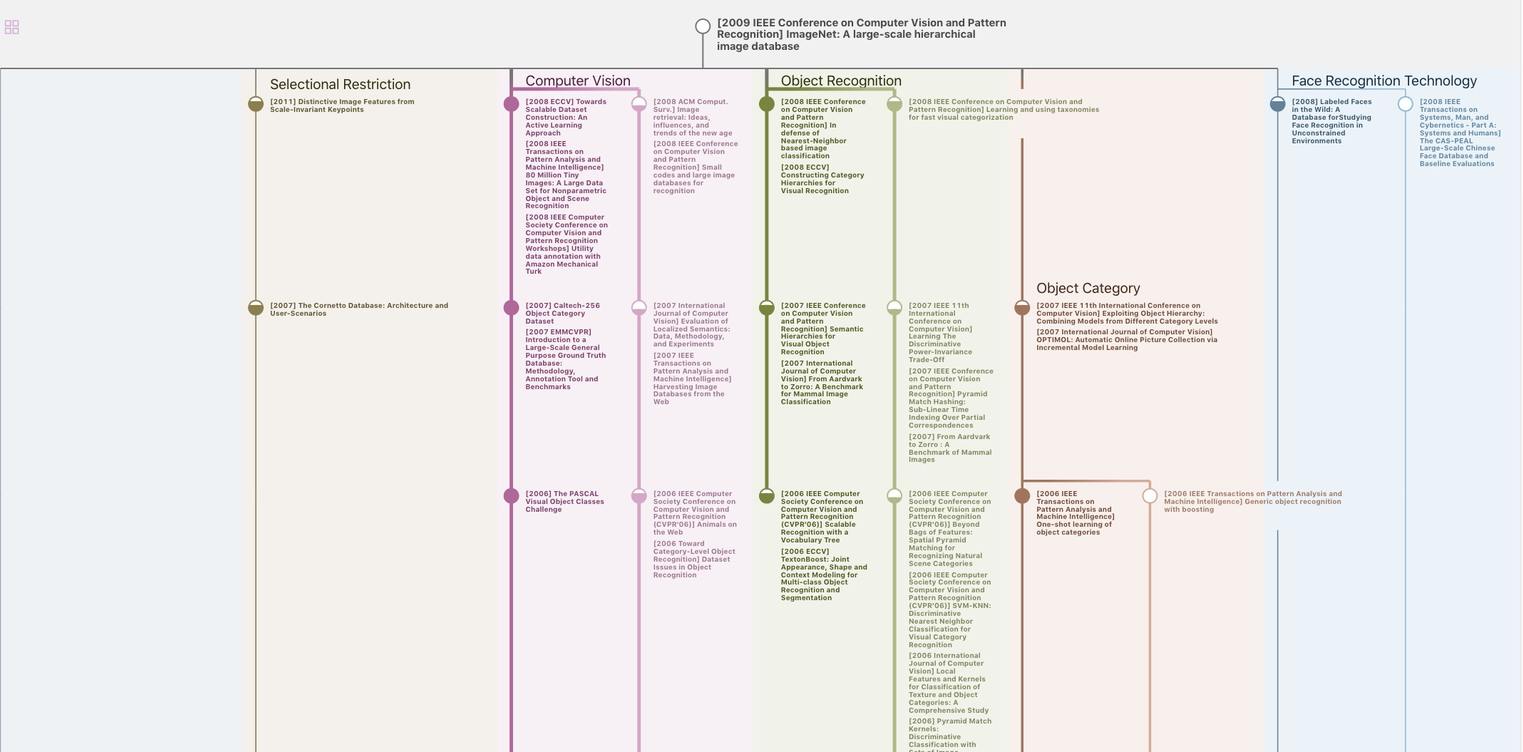
生成溯源树,研究论文发展脉络
Chat Paper
正在生成论文摘要