Developing a machine learning framework for estimating soil moisture with VNIR hyperspectral data
ISPRS TC I MID-TERM SYMPOSIUM INNOVATIVE SENSING - FROM SENSORS TO METHODS AND APPLICATIONS(2018)
摘要
In this paper, we investigate the potential of estimating the soil-moisture content based on VNIR hyperspectral data combined with LWIR data. Measurements from a multi-sensor field campaign represent the benchmark dataset which contains measured hyperspectral, LWIR, and soil-moisture data conducted on grassland site. We introduce a regression framework with three steps consisting of feature selection, preprocessing, and well-chosen regression models. The latter are mainly supervised machine learning models. An exception are the self-organizing maps which combine unsupervised and supervised learning. We analyze the impact of the distinct preprocessing methods on the regression results. Of all regression models, the extremely randomized trees model without preprocessing provides the best estimation performance. Our results reveal the potential of the respective regression framework combined with the VNIR hyperspectral data to estimate soil moisture measured under real-world conditions. In conclusion, the results of this paper provide a basis for further improvements in different research directions.
更多查看译文
关键词
Hyperspectral data, Machine learning, Regression, Soil moisture, VNIR, Field campaign
AI 理解论文
溯源树
样例
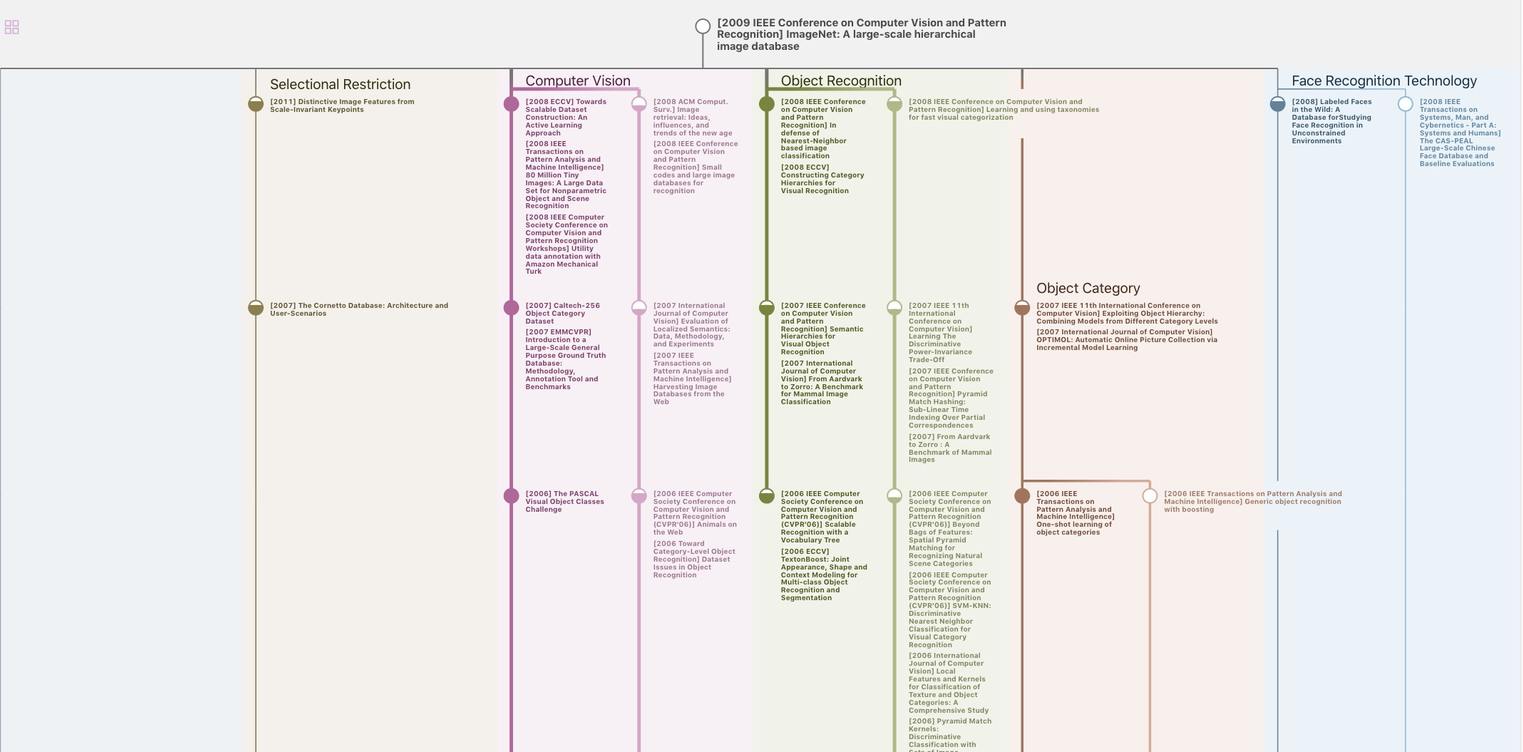
生成溯源树,研究论文发展脉络
Chat Paper
正在生成论文摘要