Nesterov-Aided Stochastic Gradient Methods Using Laplace Approximation For Bayesian Design Optimization
COMPUTER METHODS IN APPLIED MECHANICS AND ENGINEERING(2020)
摘要
Finding the best setup for experiments is the primary concern for Optimal Experimental Design (OED). Here, we focus on the Bayesian experimental design problem of finding the setup that maximizes the Shannon expected information gain. We use the stochastic gradient descent and its accelerated counterpart, which employs Nesterov's method, to solve the optimization problem in OED. We adapt a restart technique, originally proposed for the acceleration in deterministic optimization, to improve stochastic optimization methods. We combine these optimization methods with three estimators of the objective function: the double-loop Monte Carlo estimator (DLMC), the Monte Carlo estimator using the Laplace approximation for the posterior distribution (MCLA) and the double-loop Monte Carlo estimator with Laplace-based importance sampling (DLMCIS). Using stochastic gradient methods and Laplace-based estimators together allows us to use expensive and complex models, such as those that require solving partial differential equations (PDEs). From a theoretical viewpoint, we derive an explicit formula to compute the gradient estimator of the Monte Carlo methods, including MCLA and DLMCIS. From a computational standpoint, we study four examples: three based on analytical functions and one using the finite element method. The last example is an electrical impedance tomography experiment based on the complete electrode model. In these examples, the accelerated stochastic gradient descent method using MCLA converges to local maxima with up to five orders of magnitude fewer model evaluations than gradient descent with DLMC. (C) 2020 Elsevier B.V. All rights reserved.
更多查看译文
关键词
Optimal experimental design,Bayesian inference,Laplace approximation,Stochastic optimization,Accelerated gradient descent,Importance sampling
AI 理解论文
溯源树
样例
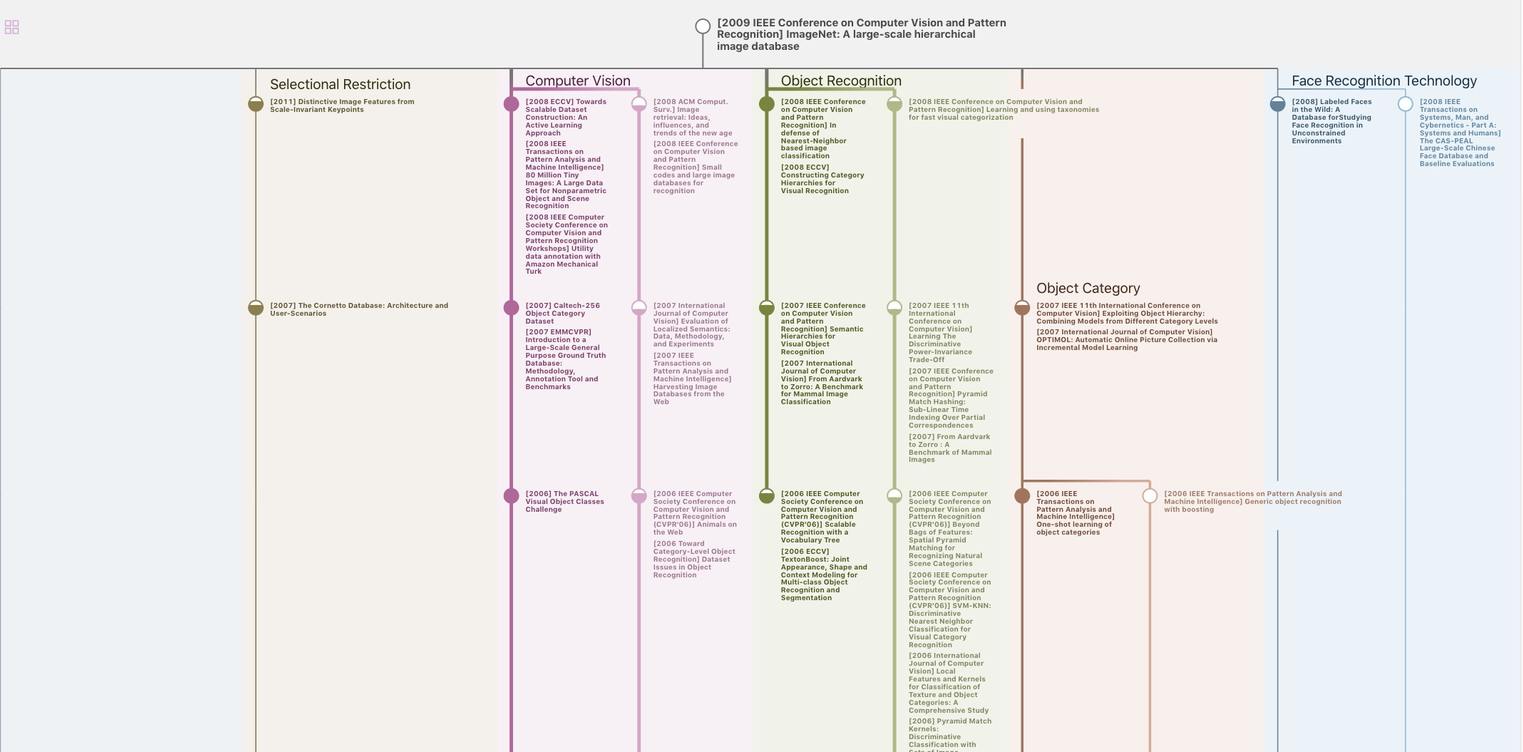
生成溯源树,研究论文发展脉络
Chat Paper
正在生成论文摘要