Comparing Neural Architectures For Demand Response Through Model-Free Reinforcement Learning For Heat Pump Control
2018 IEEE INTERNATIONAL ENERGY CONFERENCE (ENERGYCON)(2018)
摘要
As batch reinforcement learning algorithms reach maturity and neural networks are used increasingly in reinforcement learning, a performance comparison of these models should be performed. This paper discusses the implementation of a heat pump agent in a demand response setting and its cost effectiveness when implemented with different neural network types. The agent maintains the interior air temperature of a building between pre-set temperature constraints, with four actions at its disposal. The agent is incentivized to shift loads in a day-ahead market in order to minimize daily electricity costs. The simulation considered a multilayer perceptron (MLP), a convolutional neural network (CNN) and a long short-term memory neural network (LSTM) to model the environment dynamics. All architectures outperform a trivial thermostat controller and shift loads successfully after 20-25 days. For this particular setup, there is no significant difference between the MLP and the LSTM, while they do outperform the CNN model. The MLP is preferred as it requires far less computation time.
更多查看译文
关键词
fitted Q-iteration, neural networks, reinforcement learning, residential demand response
AI 理解论文
溯源树
样例
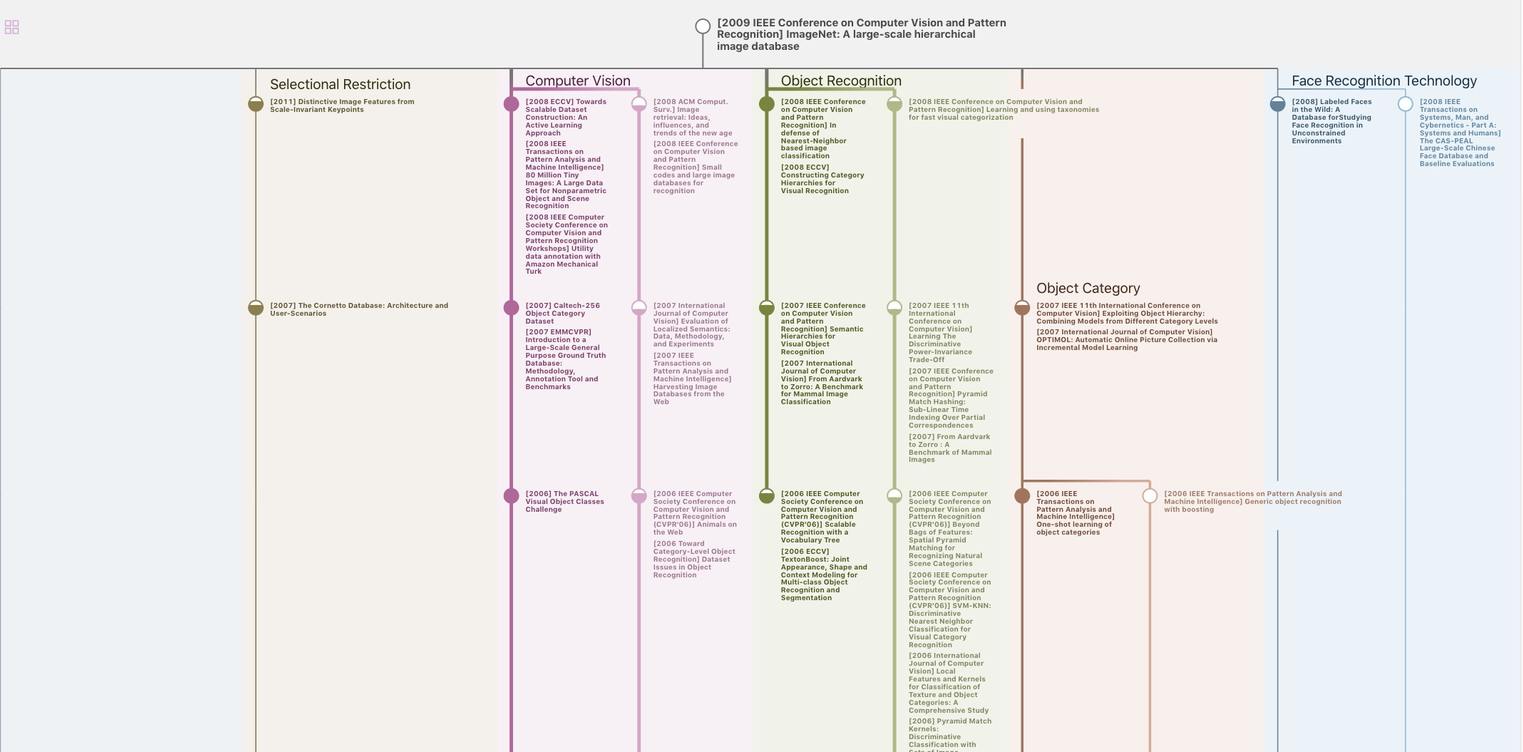
生成溯源树,研究论文发展脉络
Chat Paper
正在生成论文摘要