Using Reinforcement Learning For Optimizing Heat Pump Control In A Building Model In Modelica
2018 IEEE INTERNATIONAL ENERGY CONFERENCE (ENERGYCON)(2018)
摘要
With the increasing share of renewable energy sources in the electricity grid comes the need to exploit the available flexibility at the demand side. Demand response programs seek to exploit the flexibility of consumers by motivating end-users to shift demand based on grid signals. An important source of flexibility available at residential consumers are Thermostatically Controlled Loads (TCLs). Additionally, recent advances within the reinforcement learning area have made it possible to apply this technique to a large range of problems.Driven by these promising examples, a Batch Reinforcement Learning (BRL) algorithm is applied to a TCL. An important property of the complex optimization problem studied here, is its partial observability. The main contribution of this paper is the application of BRL to a detailed building and heating system model, implemented in Modelica. A detailed TCL model allows to perform an in-depth analysis of the effects of partial observability on the performance of the chosen control strategy.Ultimately, this paper illustrates that Modelica can be used to provide a detailed environment for a BRL algorithm. At the end, the learned control policy has been compared with two other control policies. The obtained policy outperforms both and is economically feasible after a limited amount of training days.
更多查看译文
关键词
Reinforcement Learning, Demand Response, Modelica, fitted Q-iteration
AI 理解论文
溯源树
样例
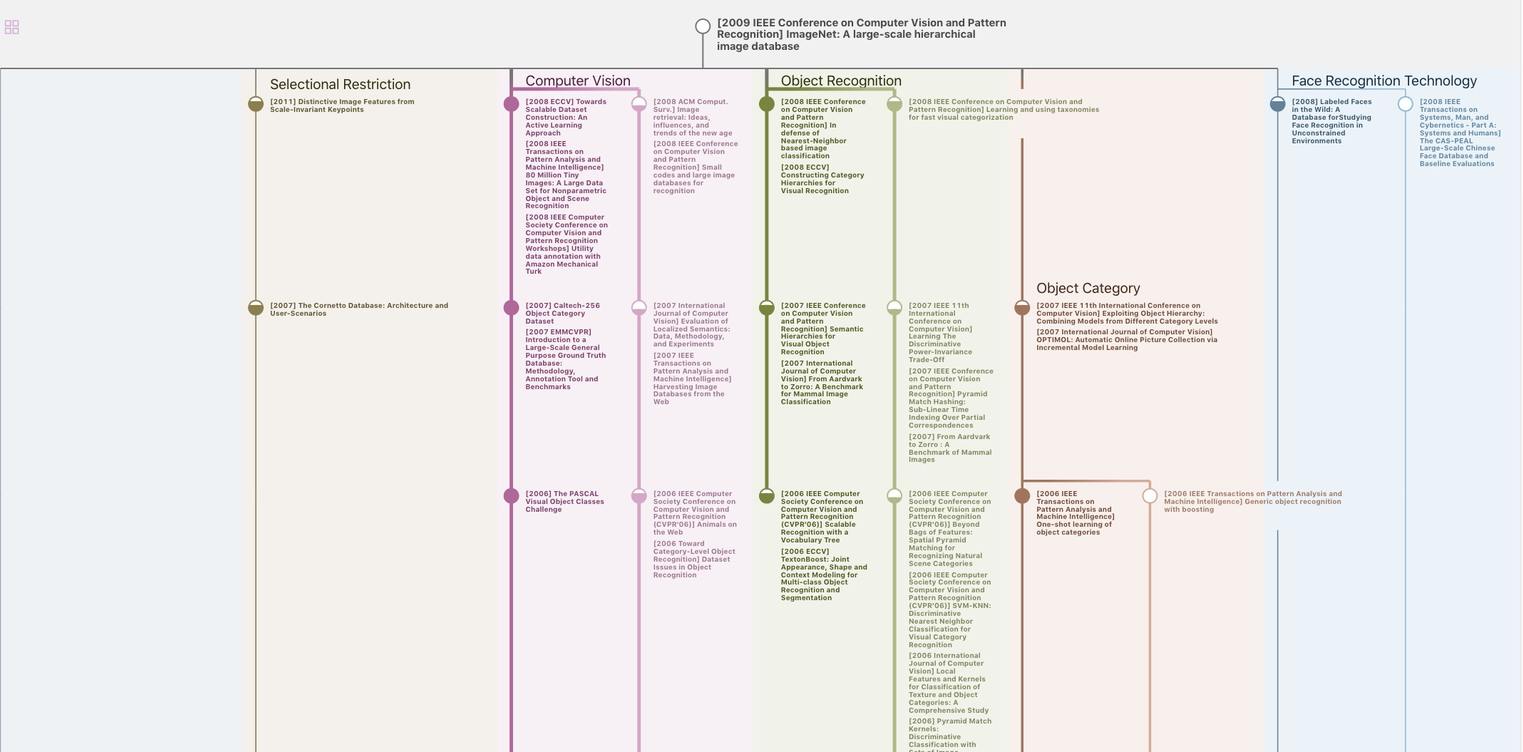
生成溯源树,研究论文发展脉络
Chat Paper
正在生成论文摘要