Covariate Powered Cross-Weighted Multiple Testing
JOURNAL OF THE ROYAL STATISTICAL SOCIETY SERIES B-STATISTICAL METHODOLOGY(2021)
摘要
A fundamental task in the analysis of data sets with many variables is screening for associations. This can be cast as a multiple testing task, where the objective is achieving high detection power while controlling type I error. We consider m hypothesis tests represented by pairs ((Pi,Xi))1 <= i <= m of p-values Pi and covariates Xi, such that Pi perpendicular to Xi if Hi is null. Here, we show how to use information potentially available in the covariates about heterogeneities among hypotheses to increase power compared to conventional procedures that only use the Pi. To this end, we upgrade existing weighted multiple testing procedures through the independent hypothesis weighting (IHW) framework to use data-driven weights that are calculated as a function of the covariates. Finite sample guarantees, for example false discovery rate control, are derived from cross-weighting, a data-splitting approach that enables learning the weight-covariate function without overfitting as long as the hypotheses can be partitioned into independent folds, with arbitrary within-fold dependence. IHW has increased power compared to methods that do not use covariate information. A key implication of IHW is that hypothesis rejection in common multiple testing setups should not proceed according to the ranking of the p-values, but by an alternative ranking implied by the covariate-weighted p-values.
更多查看译文
关键词
Benjamini-Hochberg, empirical Bayes, false discovery rate, Independent Hypothesis Weighting, multiple testing, p-value weighting
AI 理解论文
溯源树
样例
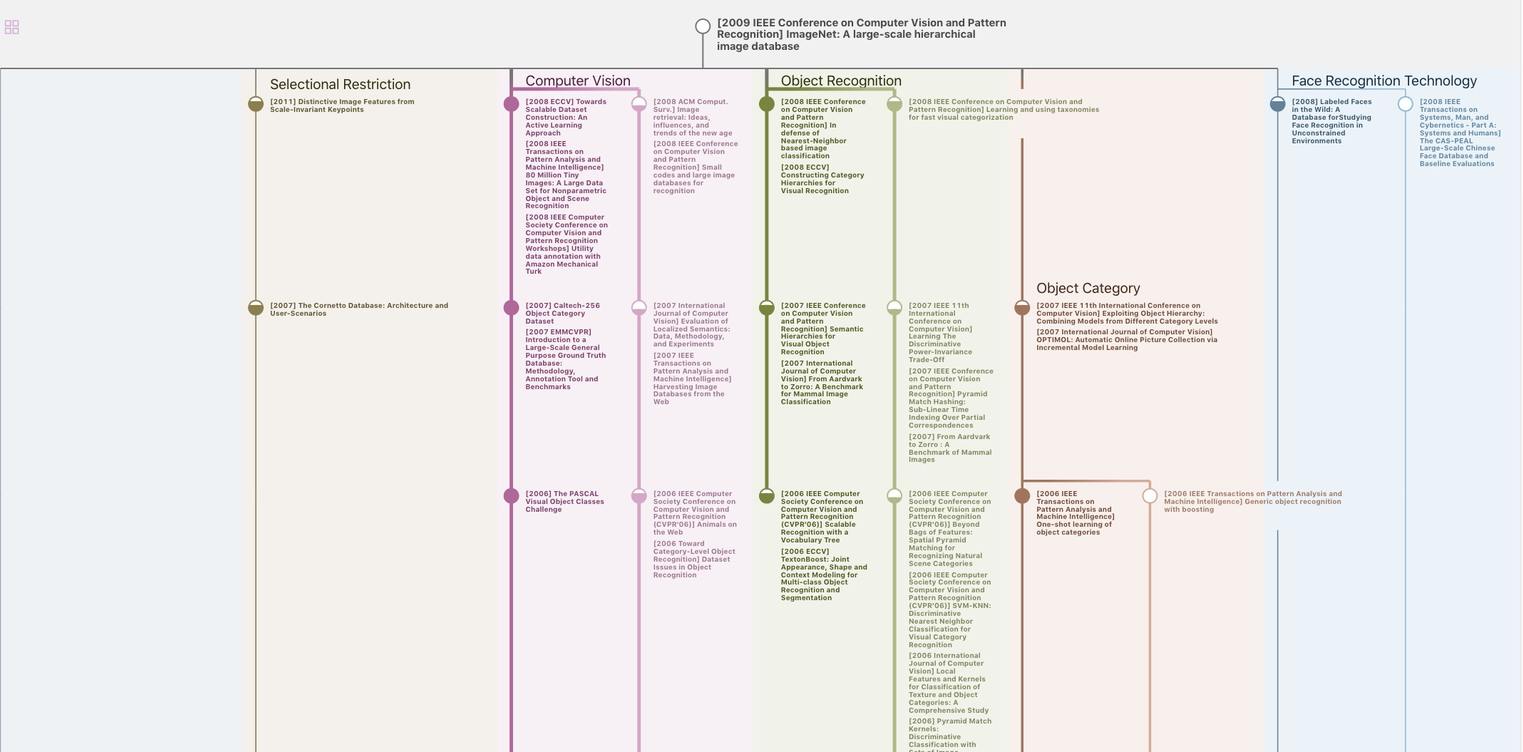
生成溯源树,研究论文发展脉络
Chat Paper
正在生成论文摘要