Multiple testing when many p-values are uniformly conservative, with application to testing qualitative interaction in educational interventions
JOURNAL OF THE AMERICAN STATISTICAL ASSOCIATION(2019)
摘要
In the evaluation of treatment effects, it is of major policy interest to know if the treatment is beneficial for some and harmful for others, a phenomenon known as qualitative interaction. We formulate this question as a multiple testing problem with many conservative null p-values, in which the classical multiple testing methods may lose power substantially. We propose a simple technique-conditioning-to improve the power. A crucial assumption we need is uniform conservativeness, meaning for any conservative p-value p, the conditional distribution (p/tau) vertical bar p <= tau is stochastically larger than the uniform distribution on (0, 1) for any tau. We show this property holds for one-sided tests in a one-dimensional exponential family (e.g., testing for qualitative interaction) as well as testing vertical bar mu vertical bar <= eta using a statistic Y similar to N(mu, 1) (e.g., testing for practical importance with threshold eta). We propose an adaptive method to select the threshold tau. Our theoretical and simulation results suggest that the proposed tests gain significant power when many p-values are uniformly conservative and lose little power when no p-value is uniformly conservative. We apply our method to two educational intervention datasets.
更多查看译文
关键词
Global null,Meta-analysis,Multisite study,Selective inference,Treatment effect heterogeneity,Uniform conditional stochastic order
AI 理解论文
溯源树
样例
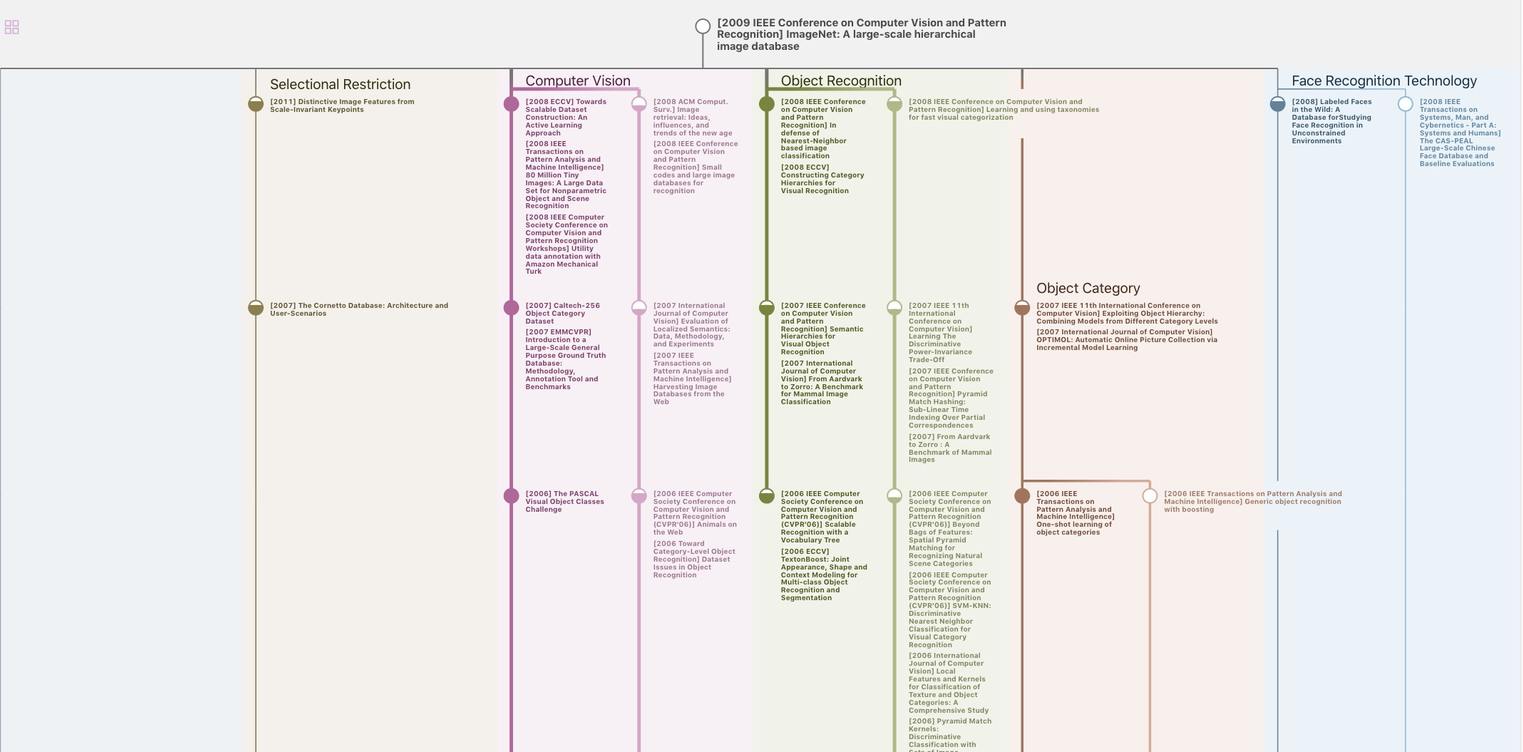
生成溯源树,研究论文发展脉络
Chat Paper
正在生成论文摘要