Multivariate Extremes of a Random Number of Maxima.
arXiv: Methodology(2018)
摘要
The classical multivariate extreme-value theory concerns the modeling of extremes in a multivariate random sample. The observations with large values in at least one component is an example of this. The theory suggests the use of max-stable distributions. In this work the classical theory is extended to the case where aggregated data, such as maxima of a random number of observations, are the actual interest. A new limit theorem concerning the domain of attraction for the distribution of the aggregated data is derived, which boils down to a new family of max-stable distributions. A practical implication of our result is, for instance, the derivation of an approximation of the joint upper tail probability for the aggregated data. The connection between the extremal dependence structure of classical max-stable distributions and that of our new family of max-stable distributions is established. By means of the so-called inverse problem, a semiparametric composite-estimator for the extremal dependence of the unobservable data is derived, starting from a preliminary estimator of the extremal dependence obtained with the aggregated data. The large-sample theory of the composite-estimator is developed and its finite-sample performance is illustrated by means of a simulation study.
更多查看译文
AI 理解论文
溯源树
样例
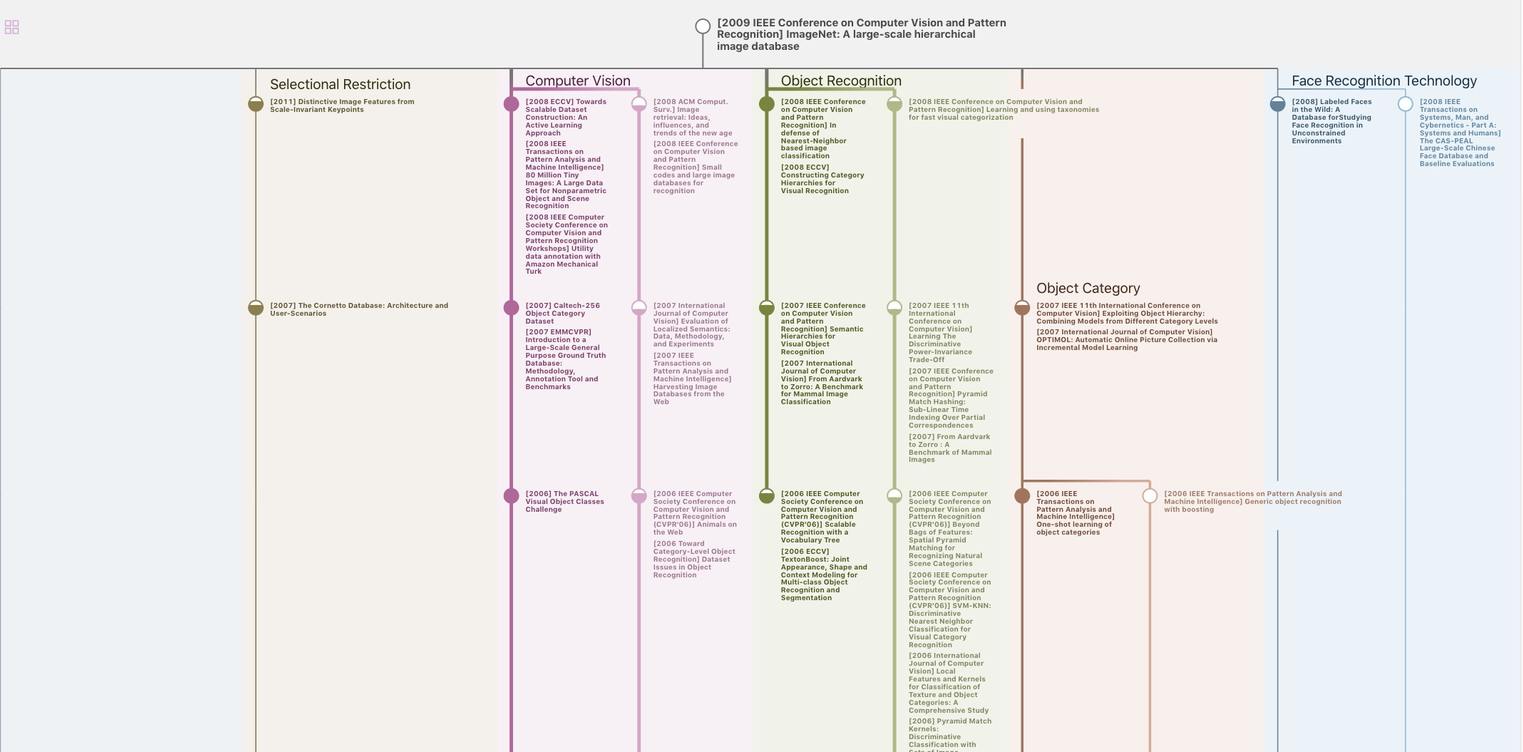
生成溯源树,研究论文发展脉络
Chat Paper
正在生成论文摘要