A framework of community detection based on individual labels in attribute networks
Physica A: Statistical Mechanics and its Applications(2018)
摘要
Community detection is an important problem for understanding the structure and function of complex networks and has attracted a lot of attention in recent decades. Most community detection algorithms only focus on the topology of networks. However, there is still much valuable information hidden in the networks, such as the attributes or content of the nodes and the useful prior information. Obviously, taking full advantage of these resources can improve the effectiveness of community detection. In this paper, we present a semi-supervised community detection framework named SCDAN (Semi-supervised Community Detection in Attribute Networks), in which a non-negative matrix factorization model is utilized to effectively integrate network topology, node attributes and individual labels simultaneously. The comparative experiments on real-world networks show that SCDAN significantly improves the performance of community detection and provides semantic interpretation of communities.
更多查看译文
关键词
Community detection,Attribute network,Individual label,Non-negative matrix factorization
AI 理解论文
溯源树
样例
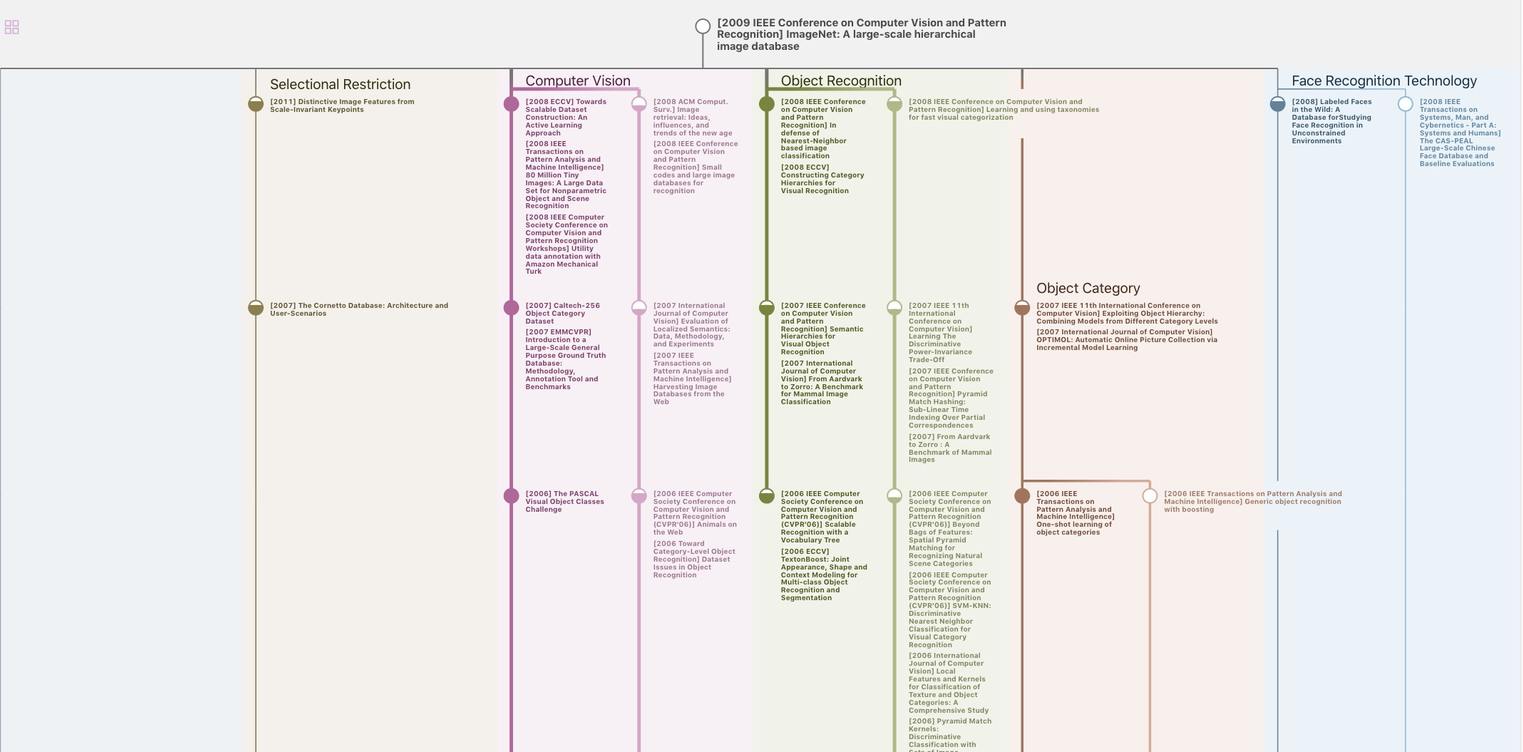
生成溯源树,研究论文发展脉络
Chat Paper
正在生成论文摘要