Multi-label Local Discriminative Embedding
Chinese Journal of Systems Engineering and Electronics(2017)
Abstract
Multi-label classification problems arise frequently in text categorization, and many other related applications. Like conventional categorization problems, multi-label categorization tasks suffer from the curse of high dimensionality. Existing multi-label dimensionality reduction methods mainly suffer from two limitations. First, latent nonlinear structures are not utilized in the input space. Second, the label information is not fully exploited. This paper proposes a new method, multi-label local discriminative embedding(MLDE), which exploits latent structures to minimize intraclass distances and maximize interclass distances on the basis of label correlations. The latent structures are extracted by constructing two sets of adjacency graphs to make use of nonlinear information. Non-symmetric label correlations, which are the case in real applications, are adopted. The problem is formulated into a global objective function and a linear mapping is achieved to solve out-of-sample problems. Empirical studies across 11 Yahoo sub-tasks, Enron and Bibtex are conducted to validate the superiority of MLDE to state-of-art multi-label dimensionality reduction methods.
MoreTranslated text
Key words
multi-label classification,dimensionality reduction,latent structure,label correlation
AI Read Science
Must-Reading Tree
Example
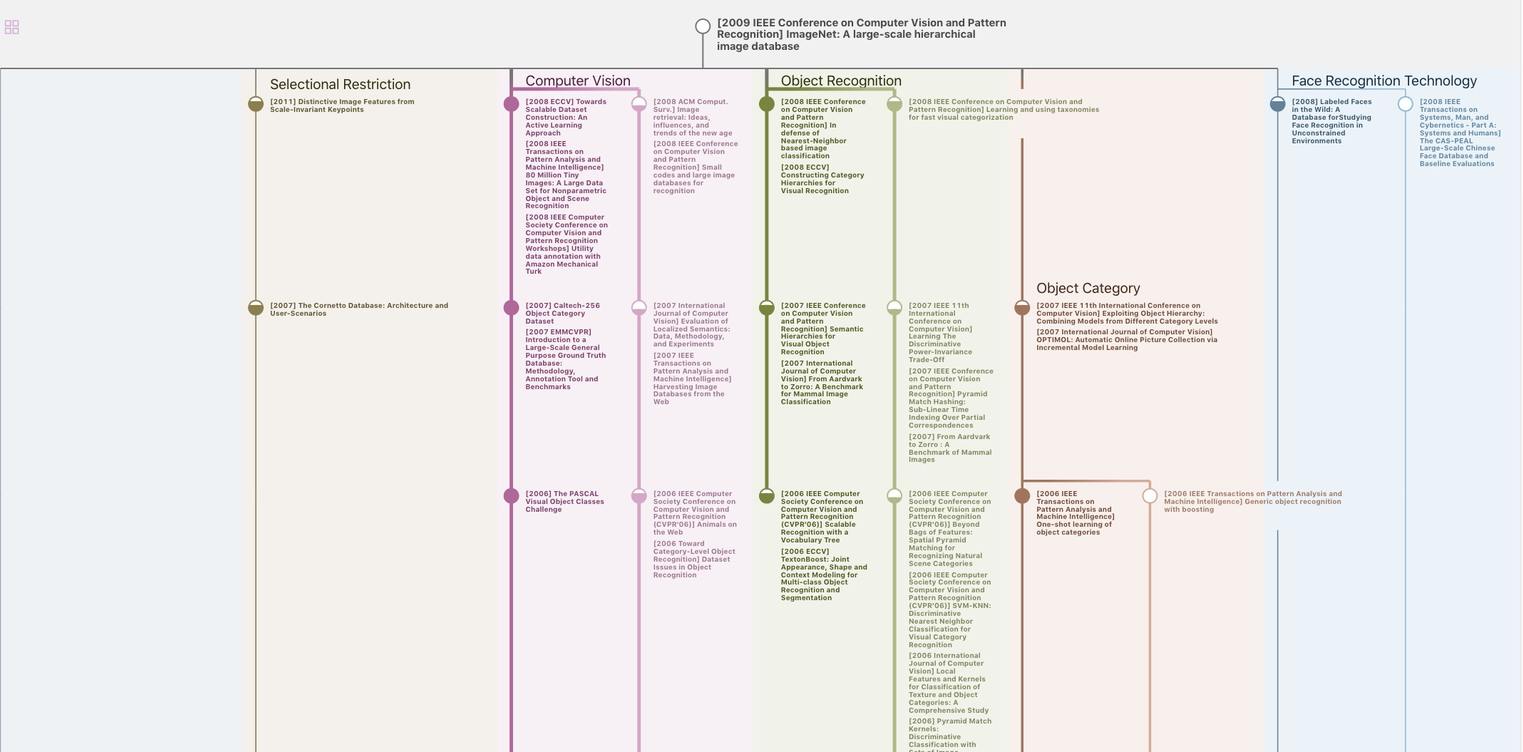
Generate MRT to find the research sequence of this paper
Chat Paper
Summary is being generated by the instructions you defined