Fatigue Status Recognition in a Post-Stroke Rehabilitation Exercise with Semg Signal
Iranian Conference on Biomedical Engineering (ICBME)(2017)
Abstract
Exercise therapy is considered as one of the main rehabilitation treatments for post-stroke patients, especially by utilizing modern technologies, such as virtual and/or augmented reality. However, in order to design an appropriate exercise program, which prolongs the exercise duration and maximize the patient's improvement, the fatigue status needs to be detected and used for the program adjustment. In the previous fatigue recognition works, only exercises for healthy and athlete subjects have been taken into account. In this paper, fatigue status classification has been accomplished in a rehabilitation exercise for poststroke patients. To do so, the reaching task, as a basic rehabilitation exercise, was performed by post-stroke patients, utilizing Xbox Kinect; and surface EMG signal and Maximum voluntary contraction (MVC) of the subjects were collected during the exercises. The MVC values were used as the reference for fatigue status. Several features were determined and extracted from the sEMG and finally, classification of fatigue status on the sE MG was performed by two well-known classifiers: Hidden Markov Model (HMM) and Artificial Neural Network (ANN). An accuracy of 95.3% was achieved by HMM, which is a promising step toward an automated fatigue status recognition system in post-stroke rehabilitation exercises.
MoreTranslated text
Key words
stroke rehabilitation,Fatigue recognition,reaching task,Xbox kinect,sEMG signal,Artificial Neural Networks,Hidden Markov Model
求助PDF
上传PDF
View via Publisher
AI Read Science
AI Summary
AI Summary is the key point extracted automatically understanding the full text of the paper, including the background, methods, results, conclusions, icons and other key content, so that you can get the outline of the paper at a glance.
Example
Background
Key content
Introduction
Methods
Results
Related work
Fund
Key content
- Pretraining has recently greatly promoted the development of natural language processing (NLP)
- We show that M6 outperforms the baselines in multimodal downstream tasks, and the large M6 with 10 parameters can reach a better performance
- We propose a method called M6 that is able to process information of multiple modalities and perform both single-modal and cross-modal understanding and generation
- The model is scaled to large model with 10 billion parameters with sophisticated deployment, and the 10 -parameter M6-large is the largest pretrained model in Chinese
- Experimental results show that our proposed M6 outperforms the baseline in a number of downstream tasks concerning both single modality and multiple modalities We will continue the pretraining of extremely large models by increasing data to explore the limit of its performance
Upload PDF to Generate Summary
Must-Reading Tree
Example
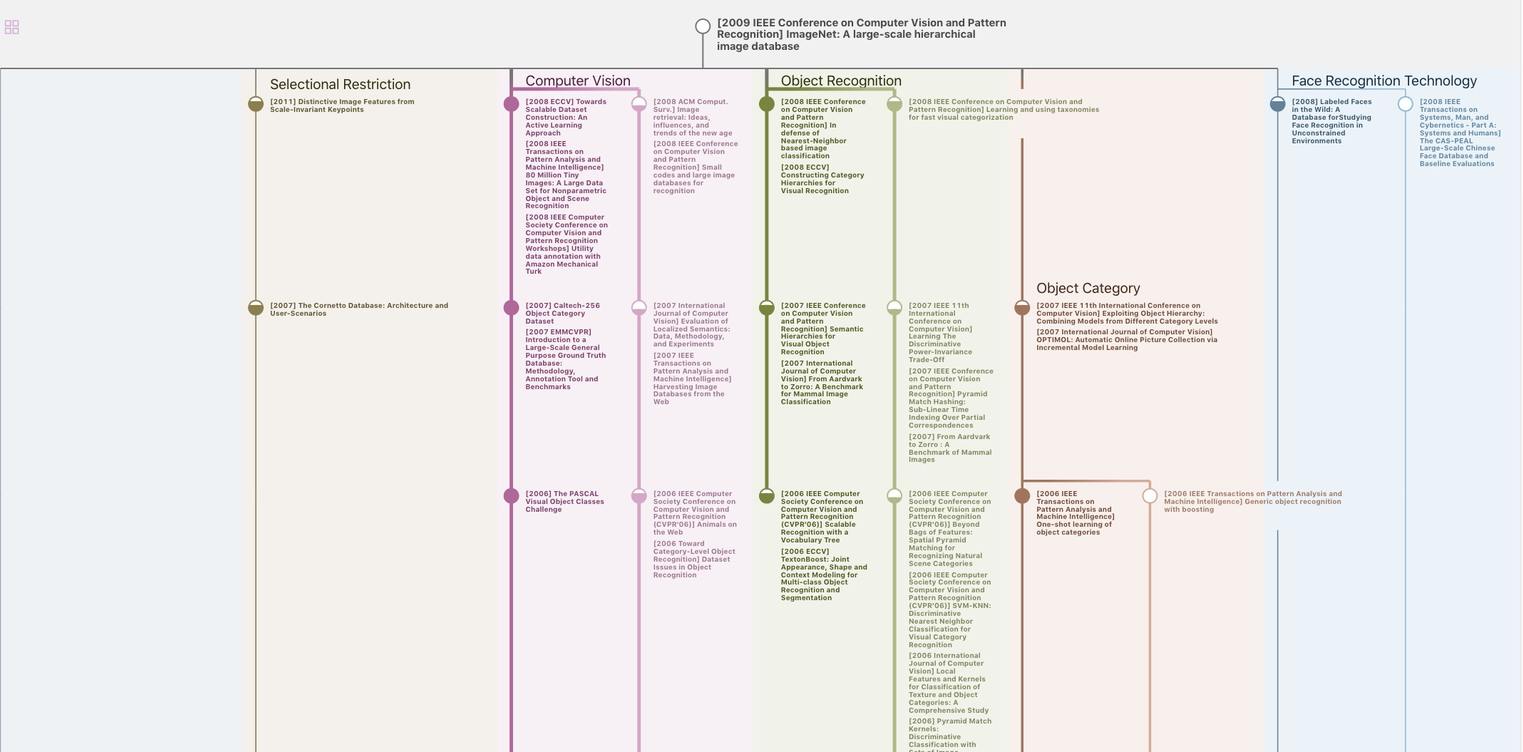
Generate MRT to find the research sequence of this paper
Related Papers
PubMed 2020
被引用1
BMC Medical Informatics and Decision Making 2022
被引用8
Application of Surface Electromyography in Exercise Fatigue: A Review
FRONTIERS IN SYSTEMS NEUROSCIENCE 2022
被引用12
Frontiers in Neurorobotics 2023
被引用0
Data Disclaimer
The page data are from open Internet sources, cooperative publishers and automatic analysis results through AI technology. We do not make any commitments and guarantees for the validity, accuracy, correctness, reliability, completeness and timeliness of the page data. If you have any questions, please contact us by email: report@aminer.cn
Chat Paper
GPU is busy, summary generation fails
Rerequest