Comparison of regression functions in a shallow convolutional neural network for natural image sharpness assessment
Proceedings of SPIE(2018)
摘要
Our previous study proposed a shallow convolutional neural network (CNN) to quantify the sharpness of natural images. The network utilized a multilayer perceptron (MLP) as its regression function in the full-connection layer. In this paper, we make use of a polynomial mapping (the logistic map, LM) as the regression function in the natural image sharpness assessment (NISA). First, the coefficient of logistic map is experimentally determined based on the database of LIVE-II. Then, the prediction performance is evaluated on Gaussian blurred images from CSIQ and TID2013. After that, three regression functions, LM, BCF (the basic cubical function) and MLP, are evaluated with Pearson linear correlation coefficient (PLCC) and Spearman rank-order correlation coefficient (SROCC). In addition, eleven state-of-the-art NISA models are compared. Based on the same shallow CNN architecture, experimental results indicate that MLP achieves the best performance, followed by BCF and LM. Furthermore, its performance is rival to or better than other NISA models. Conclusively, in comparison to LM and BCF, MLP is relatively better as a regression function for automatic network optimization and numerical regression. Meanwhile, it achieves the state-of-the-art performance in NISA task.
更多查看译文
关键词
Convolutional neural network,image sharpness assessment,logistic map,multi-layer perception
AI 理解论文
溯源树
样例
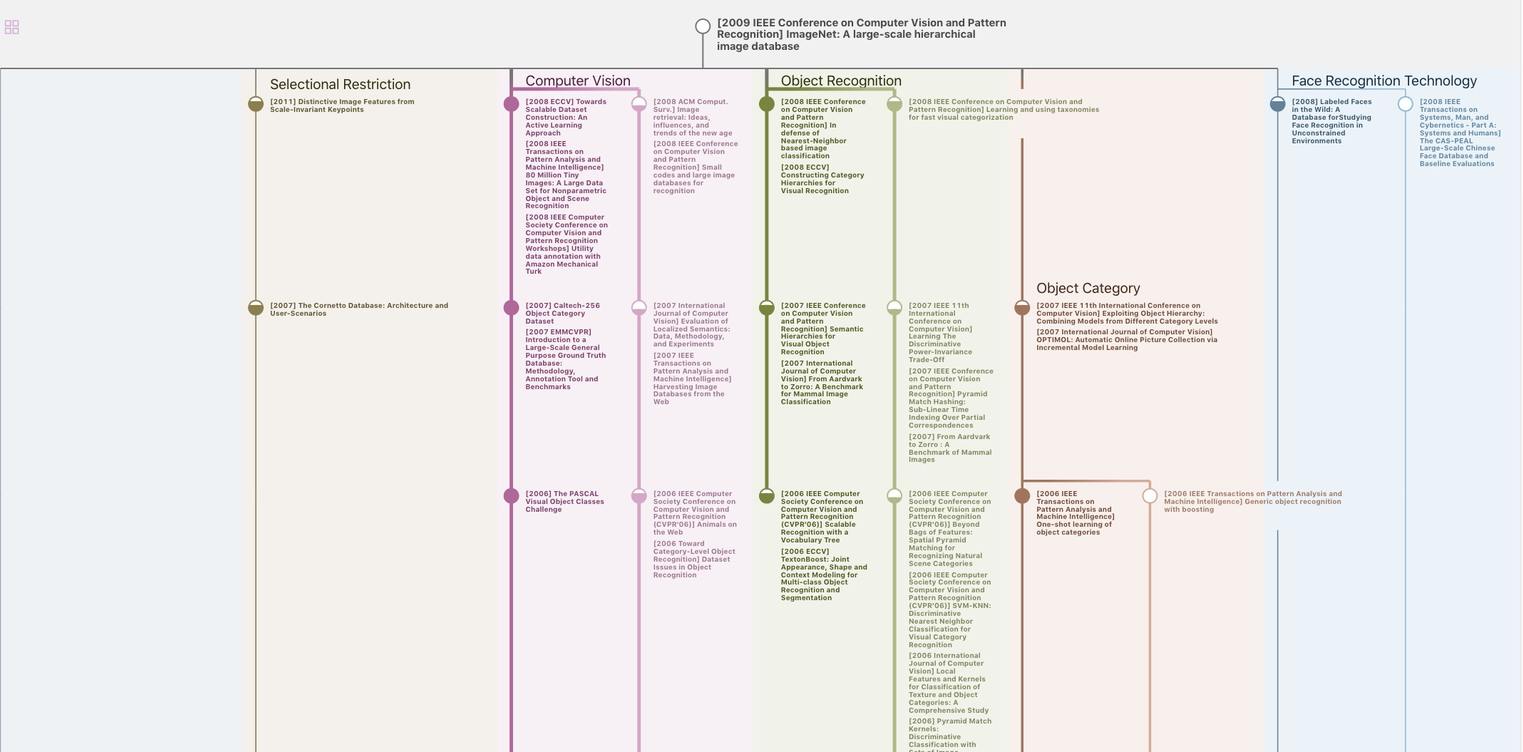
生成溯源树,研究论文发展脉络
Chat Paper
正在生成论文摘要