Bayesian Sparse Regularization For Multiple Force Identification And Location In Time Domain
INVERSE PROBLEMS IN SCIENCE AND ENGINEERING(2019)
摘要
In this work, reconstruction and location in time domain of multiple forces acting on a linear elastic structure are achieved through a Bayesian approach to solve an inverse problem. The Bayesian solution of the inverse problem is provided in the form of a posterior probability density function. The unknown forces are determined through Markov chain Monte Carlo method, the Gibbs algorithm. This posterior density integrating both the likelihood and prior information was considered for the particular case of a linear elastic structure. The measurements are affected by an additive random noise. Two particular cases were analysed: unperturbed and uncertain model representing the structure. The unperturbed model was used to identify a single force. When the model is uncertain, compressed sensing technique was used to provide an adequate sparse representation of the inverse problem through a wavelet basis. With this sparse representation, the possibility of achieving automatic location of the forces was investigated. This requires to identify all the degrees of freedom along with the identified actions are not vanishing. Also, the possibility of force identification with less sensors than forces was studied. The proposed approach is illustrated and validated on numerical examples. This proposed approach is compared with classical approach of force identification based on Tikhonov regularization associated with the GCV criterion.
更多查看译文
关键词
Multi-force identification, Bayesian regularization, regularization parameter, time domain, compressed sensing, sparse, inverse problem
AI 理解论文
溯源树
样例
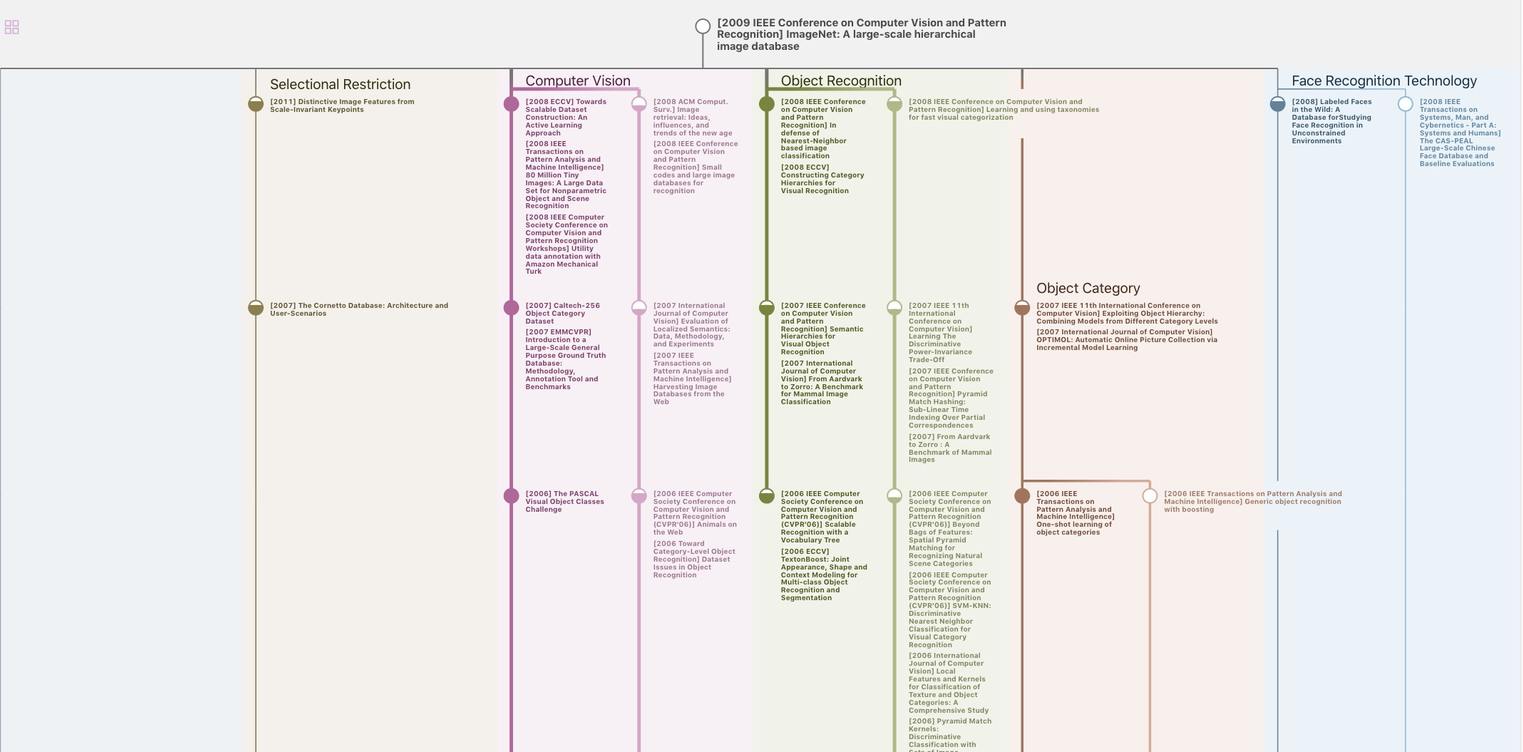
生成溯源树,研究论文发展脉络
Chat Paper
正在生成论文摘要