Multi-sensor fusion for the determination of several soil properties in the Yangtze River Delta, China: Predictions based on multi-sensor fusion
EUROPEAN JOURNAL OF SOIL SCIENCE(2019)
摘要
Soil organic matter (SOM), total nitrogen (TN), available nitrogen (AN), available phosphorus (AP), available potassium (AK) and pH are key chemical properties for evaluating soil fertility and quality. This study involved the integration of four soil sensors, visible near-infrared (vis-NIR) spectrometer, mid-infrared (mid-IR) spectrometer, portable X-ray fluorescence (PXRF) analyser and laser-induced breakdown spectroscopy (LIBS), to achieve rapid measurement of these soil properties. A genetic algorithm and partial least-squares regression (GA-PLSR) were used to select characteristic bands to reduce data redundancy. We then calibrated models from three aspects: models using partial least-squares regression (PLSR) based on single sensor data, models using PLSR based on fused sensor data, involving data combined from the four sensors into a new dataset to create a data fusion (DF) model, and models with Bayesian model averaging (BMA) based on prediction results of fused sensor data, involving prediction results combined from the four sensors into a new dataset to form the BMA model. The results showed the following. (i) For the single sensor, the predictive performance decreased as follows: mid-IR> vis-NIR > LIBS > PXRF. (ii) Compared with the single sensor approach, the DF approach slightly improved or even reduced prediction accuracy and caused a large amount of redundancy. We suggest that this approach is not able to improve predictive ability. (iii) The BMA approach achieved the best prediction for the six soil properties. Our findings suggest that model averaging of vis-NIR, mid-IR and LIBS could be a reliable and stable approach for the fast measurement of soil properties.
更多查看译文
AI 理解论文
溯源树
样例
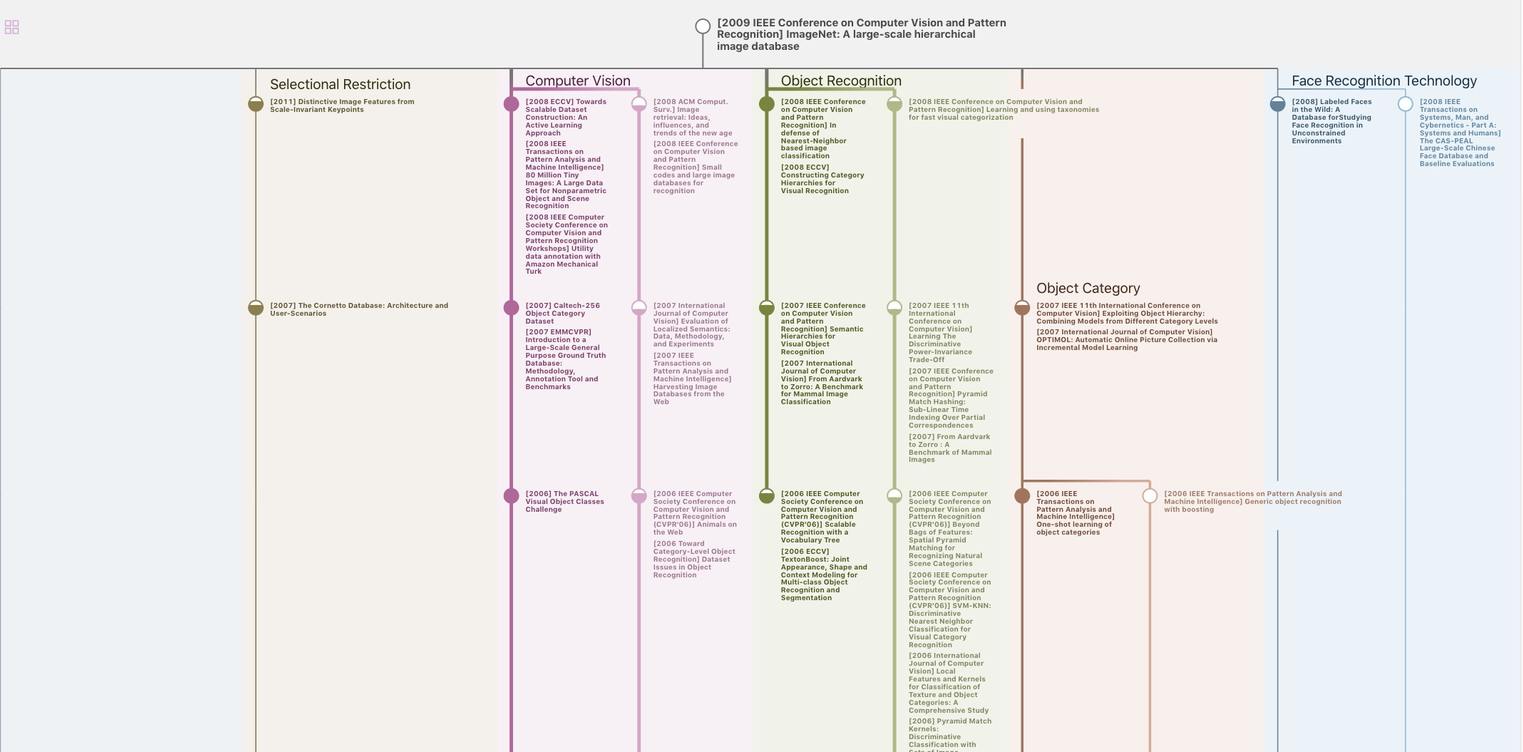
生成溯源树,研究论文发展脉络
Chat Paper
正在生成论文摘要