Deep social collaborative filtering by trust
international conference on big data(2018)
摘要
The primary purpose of recommender system is providing valuable and pertinent information actively according to usersu0027 preference. Collaborative filtering (CF) is a successful approach among different recommendation techniques. However, CF-based methods usually suffer from limited performance due to the sparsity of rating data. To address such issues, utilizing auxiliary information such as content information and social networks is a very promising approach. This paper proposes an effective Bayesian hierarchical framework called Deep Trust Recommender (DTR), which aims to improve the quality of recommender systems by integrating user-item rating information and social trust network into the embedding representation. Specifically, we first apply deep learning methods like stacked denoising auto-encoders to learn the approximation representation of the users preference from the users trust network, and then take this representation to train with rating information collaboratively. Extensive experiments on two real-world datasets reveal that our approach performs much better than several widely adopted state-of-the-art recommendation methods.
更多查看译文
AI 理解论文
溯源树
样例
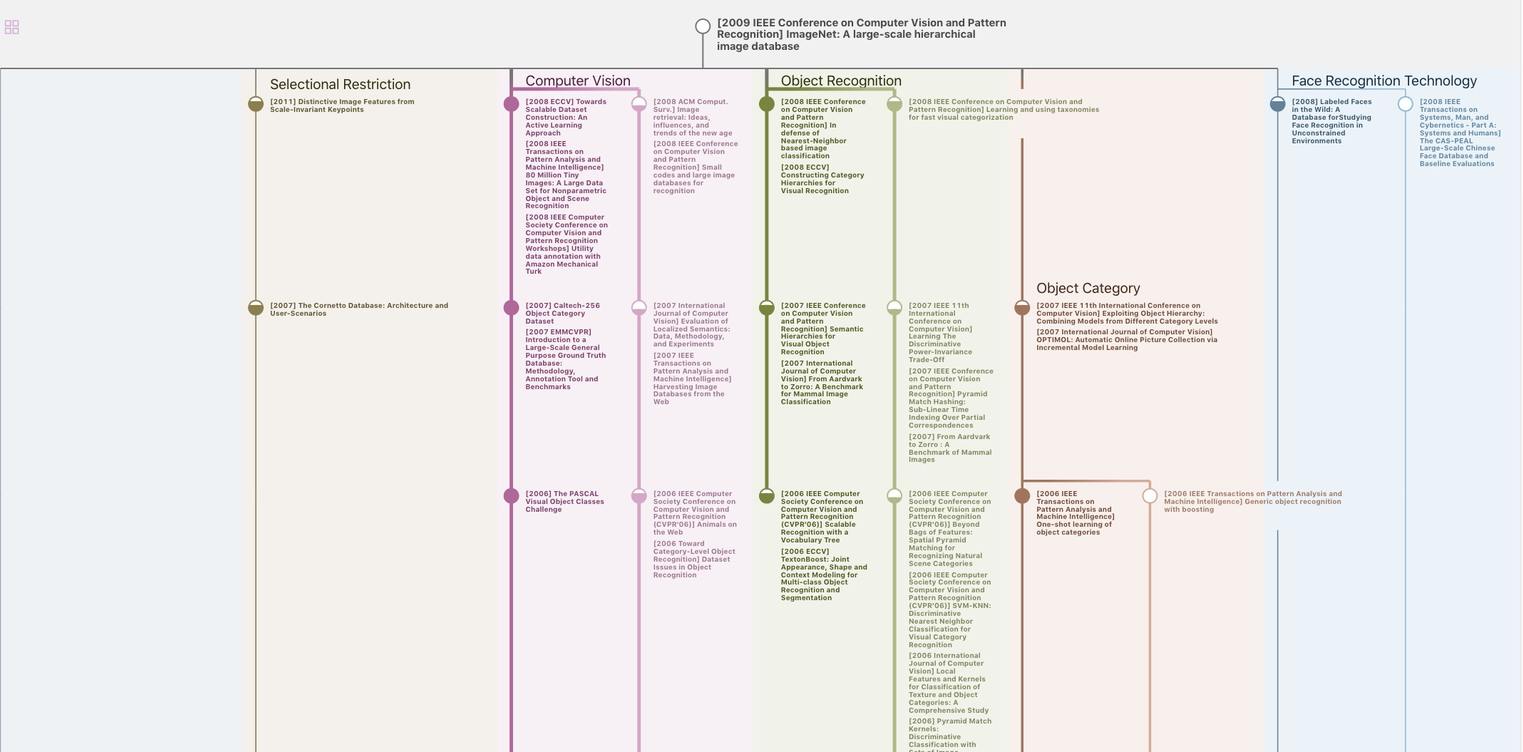
生成溯源树,研究论文发展脉络
Chat Paper
正在生成论文摘要