Low and high grade glioma segmentation in multispectral brain MRI data
Acta Universitatis Sapientiae: Informatica(2018)
摘要
Several hundreds of thousand humans are diagnosed with brain cancer every year, and the majority dies within the next two years. The chances of survival could be easiest improved by early diagnosis. This is why there is a strong need for reliable algorithms that can detect the presence of gliomas in their early stage. While an automatic tumor detection algorithm can support a mass screening system, the precise segmentation of the tumor can assist medical staff at therapy planning and patient monitoring. This paper presents a random forest based procedure trained to segment gliomas in multispectral volumetric MRI records. Beside the four observed features, the proposed solution uses 100 further features extracted via morphological operations and Gabor wavelet filtering. A neighborhood-based post-processing was designed to regularize and improve the output of the classifier. The proposed algorithm was trained and tested separately with the 54 low-grade and 220 high-grade tumor volumes of the MICCAI BRATS 2016 training database. For both data sets, the achieved accuracy is characterized by an overall mean Dice score > 83%, sensitivity > 85%, and specificity > 98%. The proposed method is likely to detect all gliomas larger than 10 mL.
更多查看译文
关键词
machine learning,random forest,image segmentation
AI 理解论文
溯源树
样例
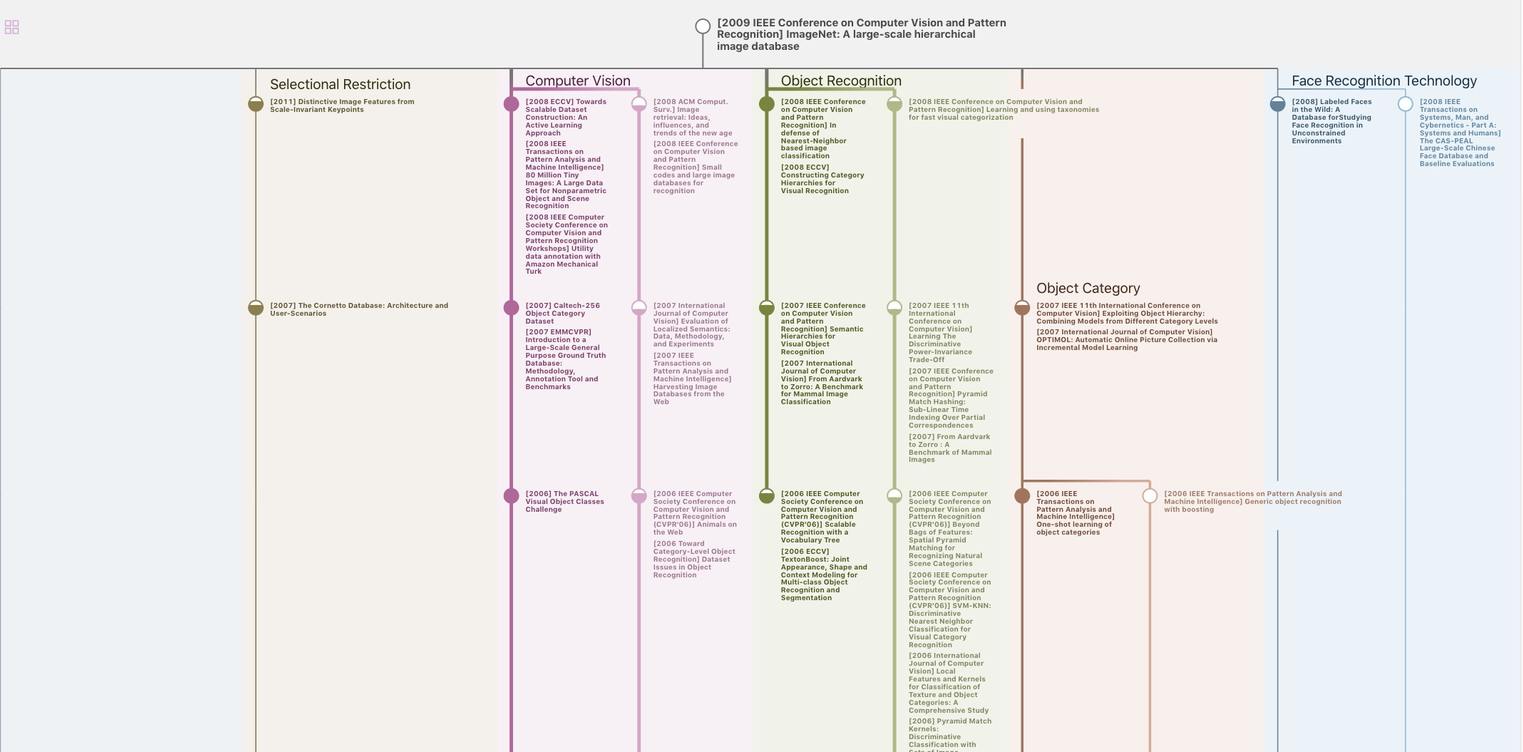
生成溯源树,研究论文发展脉络
Chat Paper
正在生成论文摘要