Prediction of Response and Toxicity to Immune Checkpoint Inhibitor Therapies (ICI) in Melanoma Using Deep Neural Networks Machine Learning.
Journal of clinical oncology(2018)
Abstract
9529 Background: Challenges in treating melanoma patients with ICI include treatment resistance and adverse events, both of which can lead to discontinuation of treatment. Histopathology of metastatic melanoma lymph nodes (LN) reveal varying histological composition(s) of malignant melanocytes and host LN reactions to tumor. Here we tested the hypothesis that deep machine learning on H&E images of metastatic melanoma LN prior to ICI can predict response and/or toxicity. Methods: H&E slides of metastatic and normal LN resected from melanoma patients (n = 45) prior to receiving ICI were digitized and annotated for regions of interest. The Inception v3 Convolutional Neural Network (CNN) was first trained to distinguish tumor LN (n = 56) from independent normal LN (n = 57). Images were tiled (299x299 pixels) at 20X magnification and partitioned into training (70%), validation (15%) and testing (15%) sets. CNNs were next trained on two classifications: 1) complete/partial response (n = 15) vs. progression of disease (n = 30) and 2) no (n = 14) vs. severe (n = 12) toxicity. Images from response (n = 45) and toxicity (n = 26) datasets were partitioned into 80% training and 20% testing sets followed by 5-fold cross validation. Predictive accuracy was measured by area under the curve (AUC) of receiver operating characteristics (ROC) plots. Sensitivity (SEN) and specificity (SPEC) were calculated at the optimal cut-off point of ROC curves. Results: Melanoma-infiltrated LNs were unambiguously distinguished from normal LNs with AUC = 1.00 per slide. Three-class training (tumor vs. lymphocytes vs. connective tissue) reported an average per-slide AUC of 0.99 [CI, 0.98-1.00], with 0.96 SEN & SPEC. In predicting response to ICI, machine learning algorithm showed AUC of 0.76 with 0.71 SEN & 0.64 SPEC. Severe toxicity was predicted with AUC of 0.70 and with 0.71 SEN & 0.64 SPEC. The AUC standard error of mean (SEM) was 0.09 for both analyses. Conclusions: Our data suggest that deep neural networks have the potential to predict patient response and toxicity to ICI with an accuracy of 70%. Independent larger datasets in clinical trial setting are pre-requisite to support validity of this novel approach.
MoreTranslated text
AI Read Science
Must-Reading Tree
Example
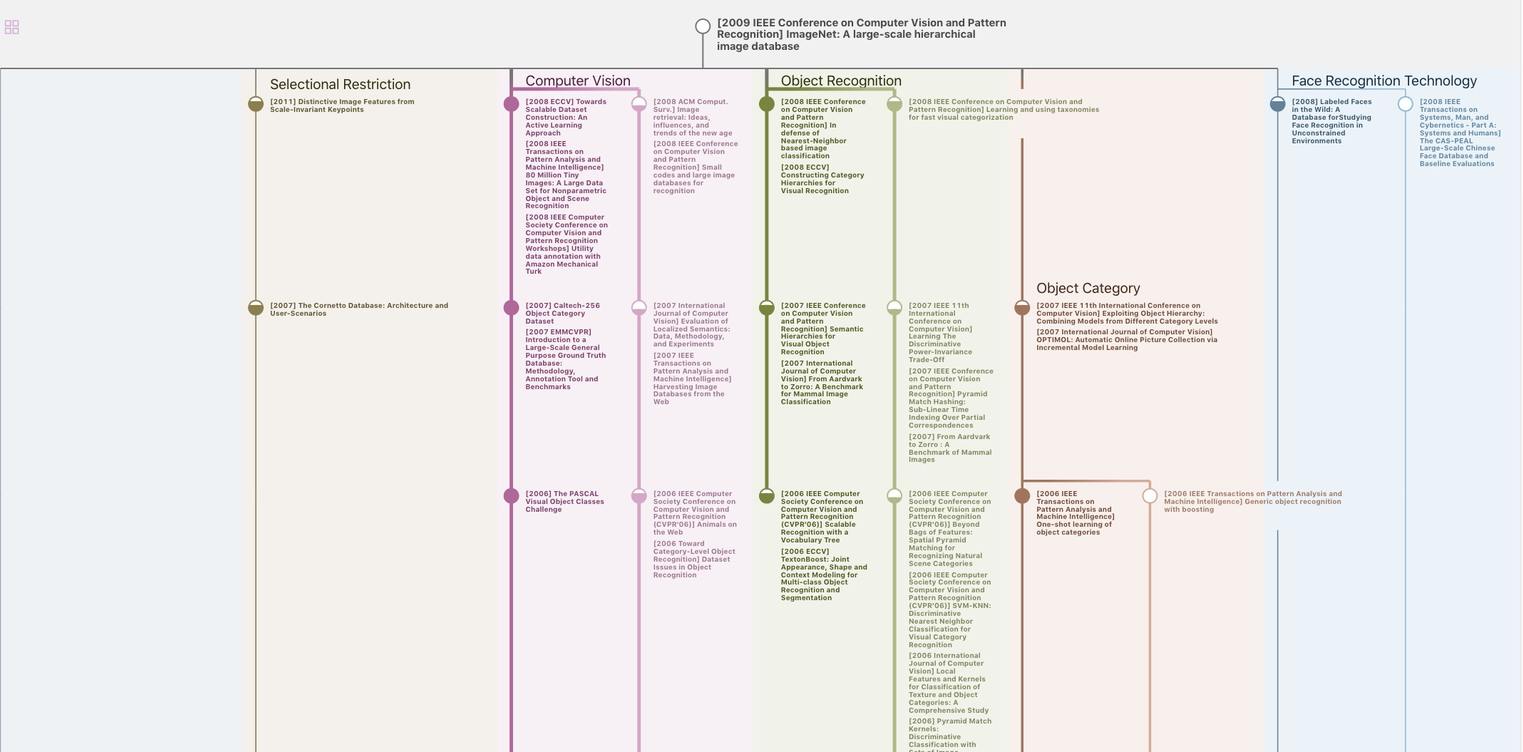
Generate MRT to find the research sequence of this paper
Chat Paper
Summary is being generated by the instructions you defined