Synchronization-based clustering on evolving data stream
Information Sciences(2019)
摘要
Clustering streams of data is of increasing importance in many applications. In this paper, we propose a new synchronization-based clustering approach for evolving data streams, called SyncTree, which maintains all micro-clusters at different levels of granularity depending upon the data recency. Instead of using a sliding window or decay function to focus on recent data, SyncTree summarizes all continuously-arriving objects as synchronized micro-clusters sequentially in a batch fashion. Owing to the powerful concept of synchronization, the derived micro-clusters truly reflect the intrinsic cluster structure rather than summarize statistics of data, and old micro-clusters can be intuitively summarized at a higher level by iterative clustering to fit memory constraints. Building upon the hierarchical micro-clusters, SyncTree allows investigating the cluster structure of the data stream between any two time stamps in the past, and also provides a principled way to analyze the cluster evolution. Empirical results demonstrate that our method has good performance compared to state-of-the-art algorithms.
更多查看译文
关键词
Data stream,Clustering,Synchronization,Evolving analysis
AI 理解论文
溯源树
样例
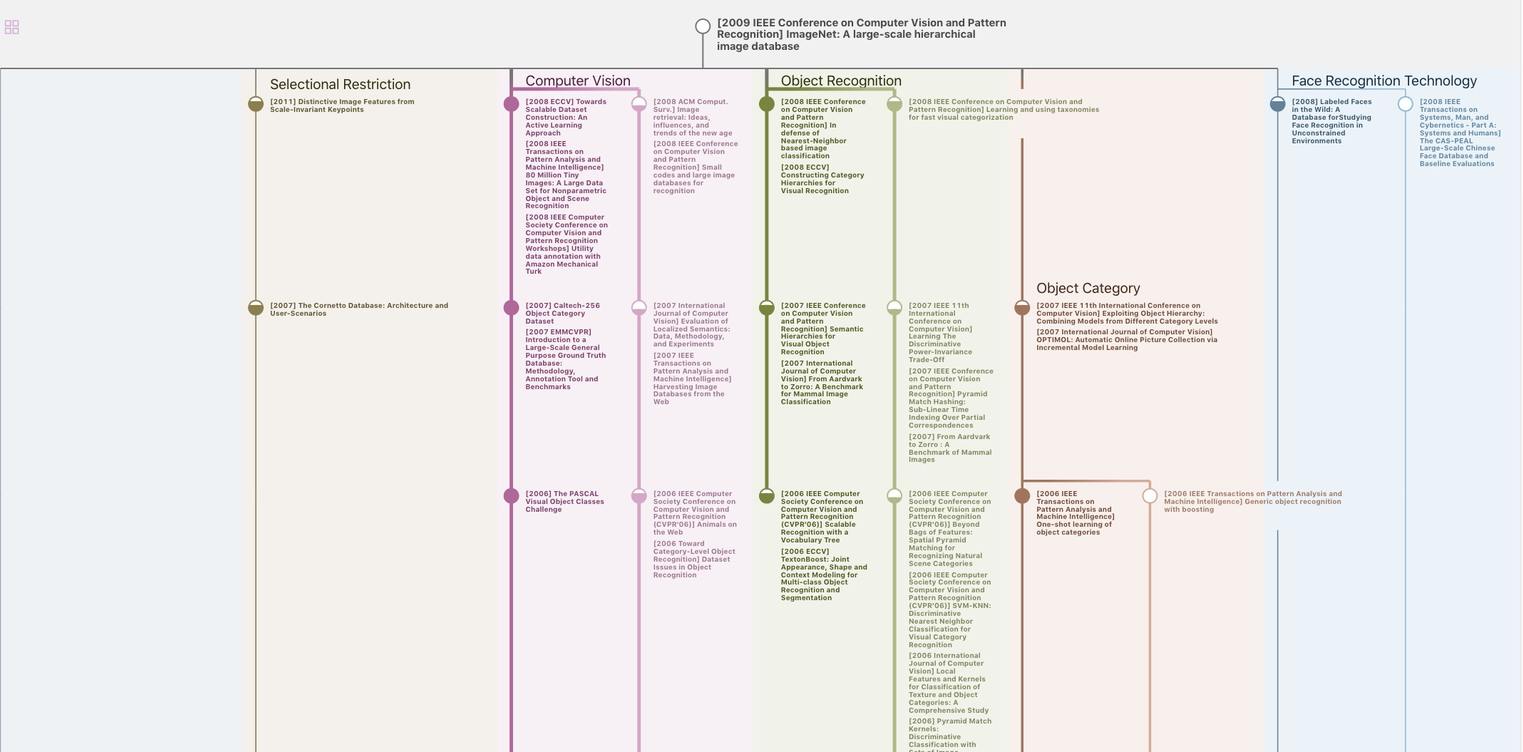
生成溯源树,研究论文发展脉络
Chat Paper
正在生成论文摘要