Dissecting heterogeneous cell-populations across signaling and disease conditions with PopAlign
bioRxiv(2020)
摘要
Single-cell measurement techniques can now probe gene expression in heterogeneous cell populations from the human body across a range of environmental and physiological conditions. However, new mathematical and computational methods are required to represent and analyze gene expression changes that occur in complex mixtures of single cells as they respond to signals, drugs, or disease states. Here, we introduce a mathematical modeling platform, PopAlign, that automatically identifies subpopulations of cells within a heterogeneous mixture, and tracks gene expression and cell abundance changes across subpopulations by constructing and comparing probabilistic models. We apply PopAlign to discover specific categories of signaling responses within primary human immune cells as well as patient-specific disease signatures in multiple myeloma that are obscured by techniques like tSNE. We anticipate that PopAlign will enable large scale studies of natural and engineered cell populations as they respond to drugs, signals or physiological change.
更多查看译文
AI 理解论文
溯源树
样例
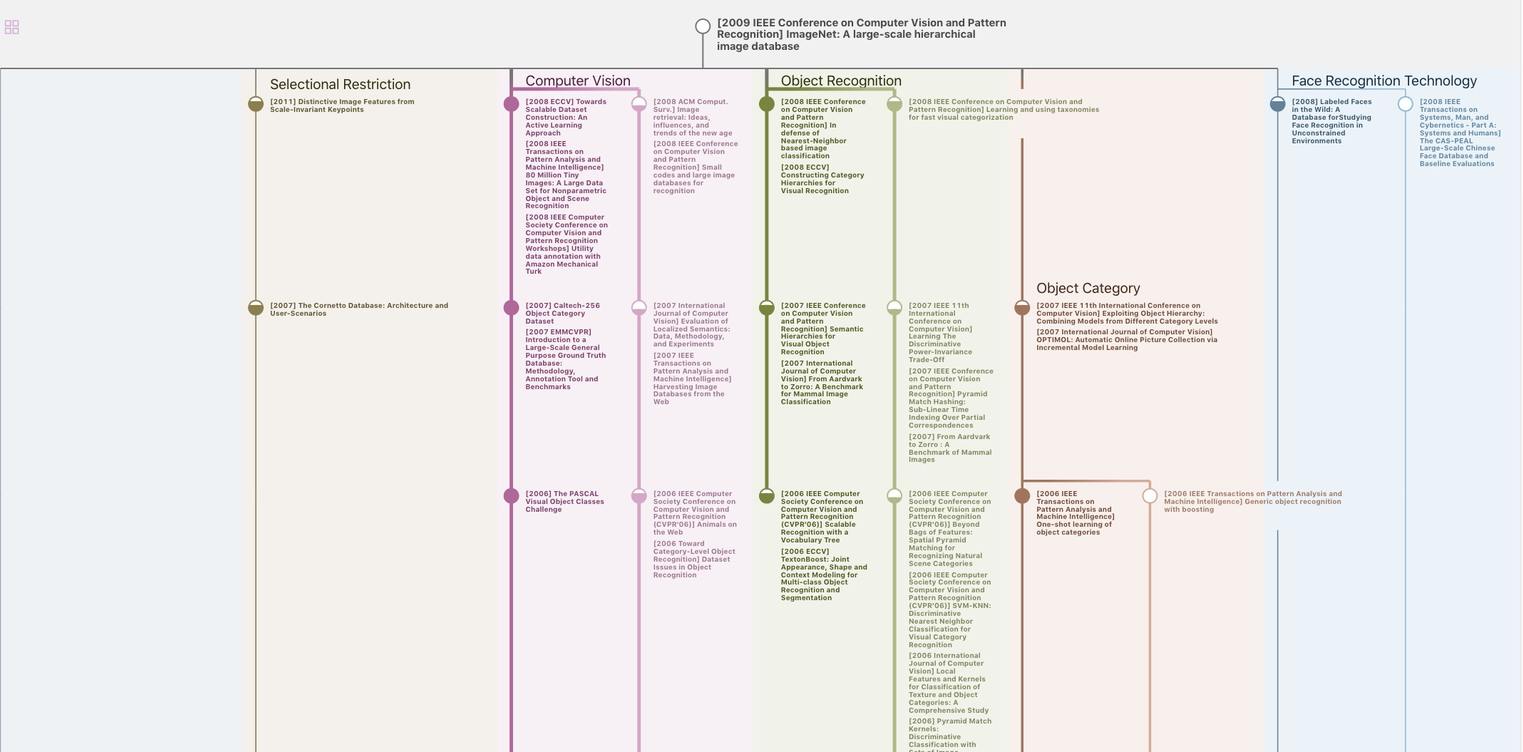
生成溯源树,研究论文发展脉络
Chat Paper
正在生成论文摘要