Convergence of a modified gradient-based learning algorithm with penalty for single-hidden-layer feed-forward networks
Neural Computing and Applications(2018)
摘要
Based on a novel algorithm, known as the upper-layer-solution-aware (USA), a new algorithm, in which the penalty method is introduced into the empirical risk, is studied for training feed-forward neural networks in this paper, named as USA with penalty. Both theoretical analysis and numerical results show that it can control the magnitude of weights of the networks. Moreover, the deterministic theoretical analysis of the new algorithm is proved. The monotonicity of the empirical risk with penalty term is guaranteed in the training procedure. The weak and strong convergence results indicate that the gradient of the total error function with respect to weights tends to zero, and the weight sequence goes to a fixed point when the iterations approach positive infinity. Numerical experiment has been implemented and effectively verifies the proved theoretical results.
更多查看译文
关键词
Neural networks,Penalty,Gradient,Monotonicity,Convergence
AI 理解论文
溯源树
样例
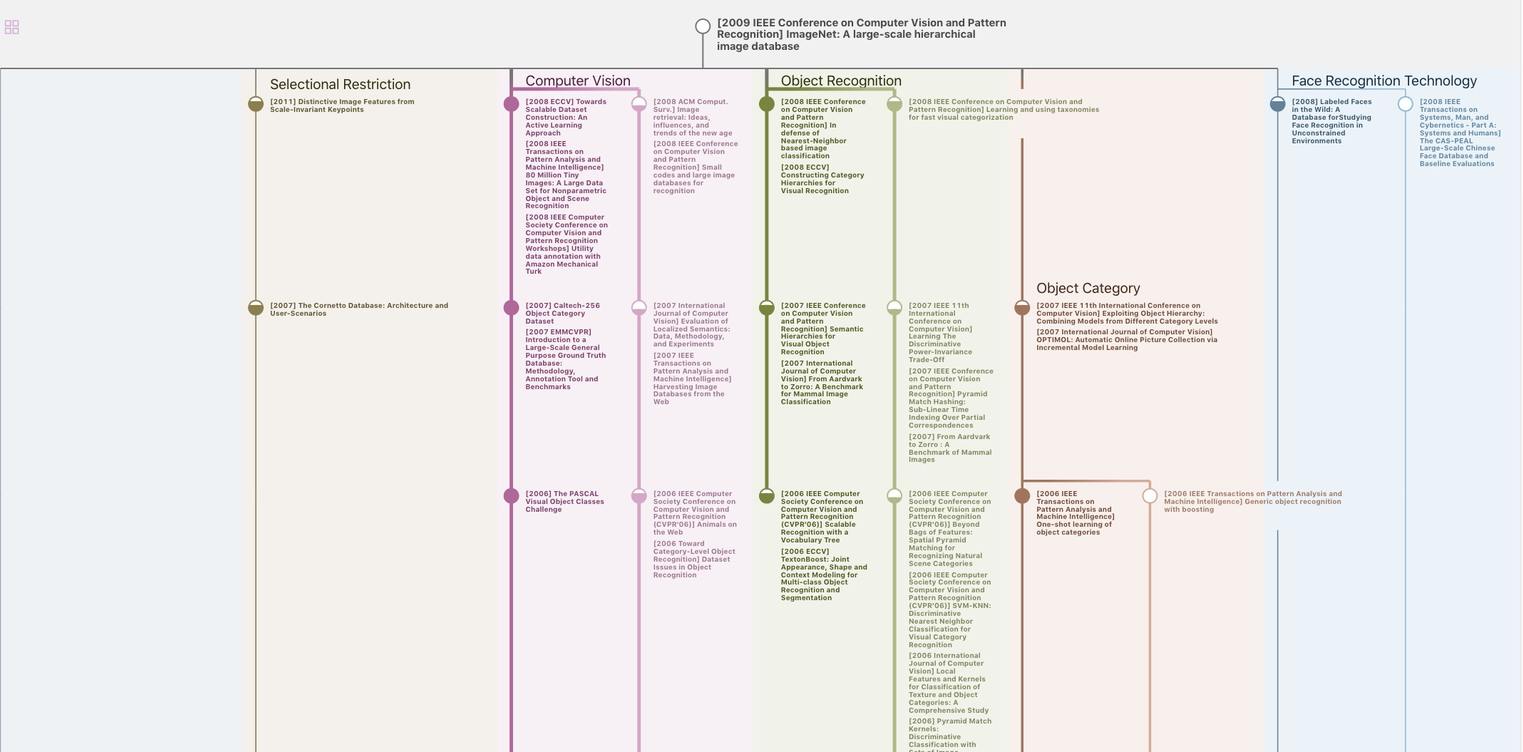
生成溯源树,研究论文发展脉络
Chat Paper
正在生成论文摘要