Mapping river bathymetries: evaluating topobathymetric LiDAR survey: River bathymetry revealed
EARTH SURFACE PROCESSES AND LANDFORMS(2019)
摘要
Advances in topobathymetric LiDARs could enable rapid surveys at sub-meter resolution over entire stream networks. This is the first step to improving our knowledge of riverine systems, both their morphology and role in ecosystems. The Experimental Advanced Airborne Research LiDAR B (EAARL-B) system is one such topobathymetric sensor, capable of mapping both terrestrial and aquatic systems. Whereas the original EAARL was developed to survey littoral areas, the new version, EAARL-B, was also designed for riverine systems but has yet to be tested. Thus, we evaluated the ability of EAARL-B to map bathymetry and floodplain topography at sub-meter resolution in a mid-size gravel-bed river. We coupled the EAARL-B survey with highly accurate field surveys (0.03m vertical accuracy and approximately 0.6 by 0.6m resolution) of three morphologically distinct reaches, approximately 200m long 15m wide, of the Lemhi River (Idaho, USA). Both point-to-point and raster-to-raster comparisons between ground and EAARL-B surveyed elevations show that differences (ground minus EAARL-B surveyed elevations) over the entire submerged topography are small (root mean square error, RMSE, and median absolute error, M, of 0.11m), and large differences (RMSE, between 0.15 and 0.38m and similar M) are mainly present in areas with abrupt elevation changes and covered by dense overhanging vegetation. RMSEs are as low as 0.03m over paved smooth surfaces, 0.07m in submerged, gradually varying topography, and as large as 0.24m along banks with and without dense, tall vegetation. EAARL-B performance is chiefly limited by point density in areas with strong elevation gradients and by LiDAR footprint size (0.2m) in areas with topographic features of similar size as the LiDAR footprint. (c) 2018 John Wiley & Sons, Ltd.
更多查看译文
关键词
topobathymetric LiDAR,streambed bathymetry,performance of green LiDAR
AI 理解论文
溯源树
样例
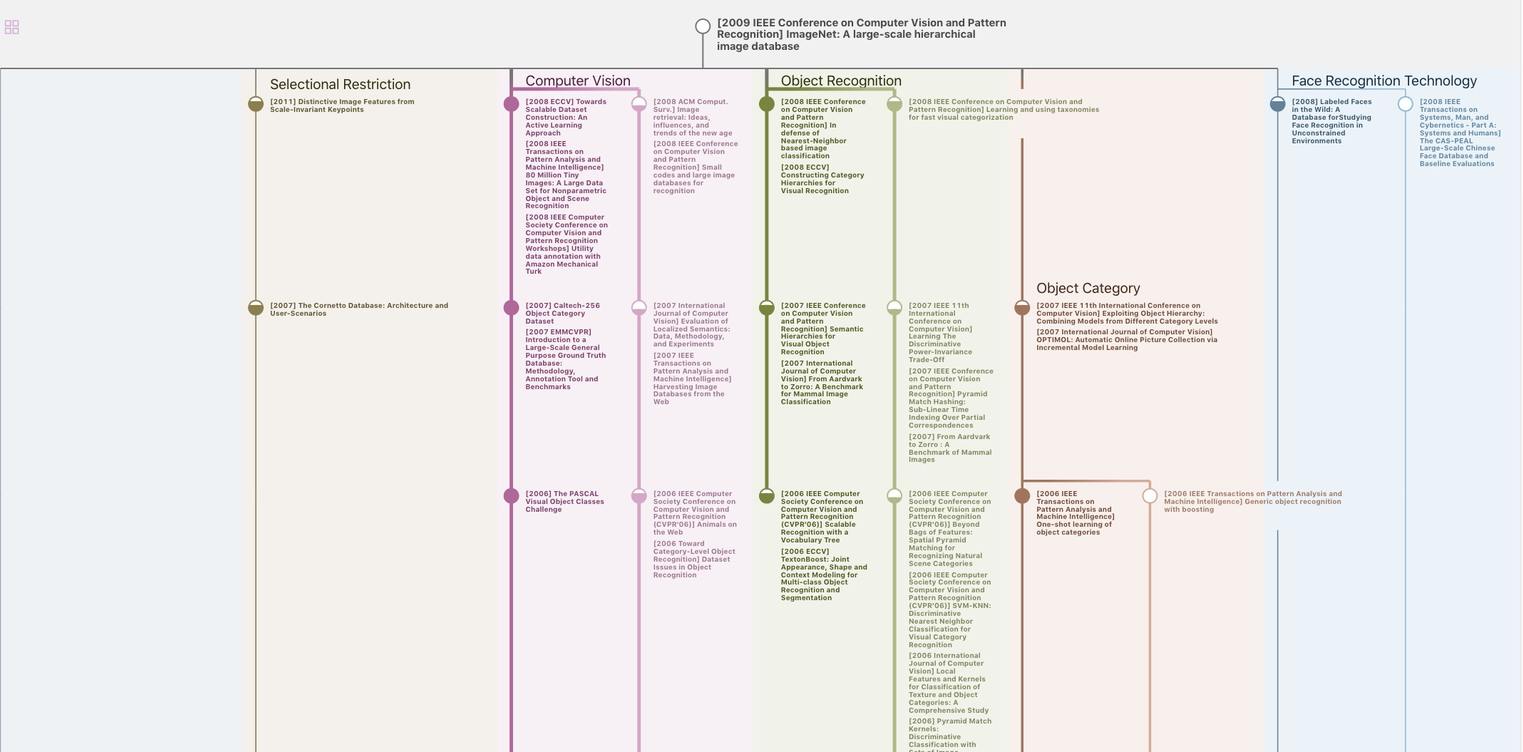
生成溯源树,研究论文发展脉络
Chat Paper
正在生成论文摘要