Data-driven region-of-interest selection for visual and attention ERP studies controls Type I error and increases power
Journal of Vision(2018)
摘要
Visual phenomena and their neural mechanisms are commonly studied with EEG or MEG measurements (e.g., N170 face-sensitive ERP component; N2pc for visual attention). During data analysis, it is often difficult to know, a priori, precisely where effects will occur on the scalp, in time and in frequency for oscillations work. To overcome this, researchers often identify regions-of-interest (ROIs) for testing, but have been criticized for sometimes using biased, data-driven methods and thereby inflating Type I error rates. Using simulations and analysis of visually evoked N170 and N2pc data, our results demonstrate an ROI-selection method which is data-driven (i.e., based on the collected data), nonetheless, does not inflate Type I error rate. Furthermore, it reduces the need for precise a priori specification of the time and location of the ROI. We identify the ROI using what we call the aggregated-grand average (AGAT) wave, which is a weighted average of trials. We demonstrate that this is orthogonal to the experimental contrast and, importantly, we show that common methods for computing orthogonal waveforms for ROI selection can inflate Type I error rate under some conditions. Based on our results, use of the AGAT overcomes this problem. Finally, we show that using the AGAT has statistical power that can exceed common a priori ROI selection methods by up to 60%. Our results demonstrate a simple, unbiased and data driven ROI selection method which is relevant for N170, N2pc and other visual and attention-related ERP components. Meeting abstract presented at VSS 2018
更多查看译文
关键词
attention erp studies,selection,data-driven,region-of-interest
AI 理解论文
溯源树
样例
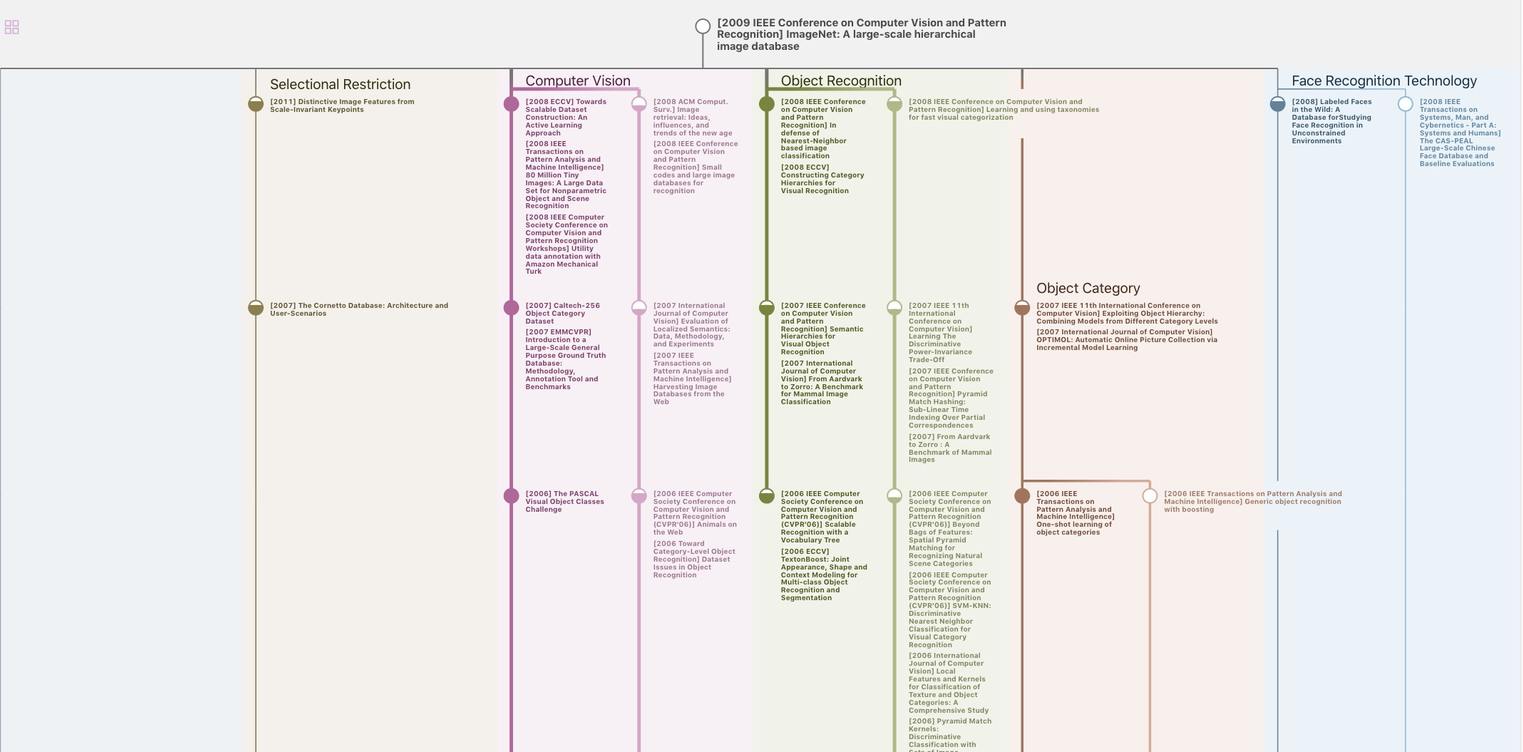
生成溯源树,研究论文发展脉络
Chat Paper
正在生成论文摘要