Random sampling and machine learning to understand good decompositions
Annals of Operations Research(2018)
摘要
Motivated by its implications in the development of general purpose solvers for decomposable Mixed Integer Programs (MIPs), we address a fundamental research question, that is how to exploit data-driven techniques to obtain automatic decomposition methods. We preliminary investigate the link between static properties of MIP input instances and good decomposition patterns. We devise a random sampling algorithm, considering a set of generic MIP base instances, and generate a large, balanced and well diversified set of decomposition patterns, that we analyze with machine learning tools. We also propose and test a minimal proof of concept framework performing data-driven automatic decomposition. The use of supervised techniques highlights interesting structures of random decompositions, as well as proving (under certain conditions) that data-driven methods are fruitful in our context, triggering at the same time perspectives for future research.
更多查看译文
关键词
Dantzig–Wolfe decomposition, Machine learning, Random sampling
AI 理解论文
溯源树
样例
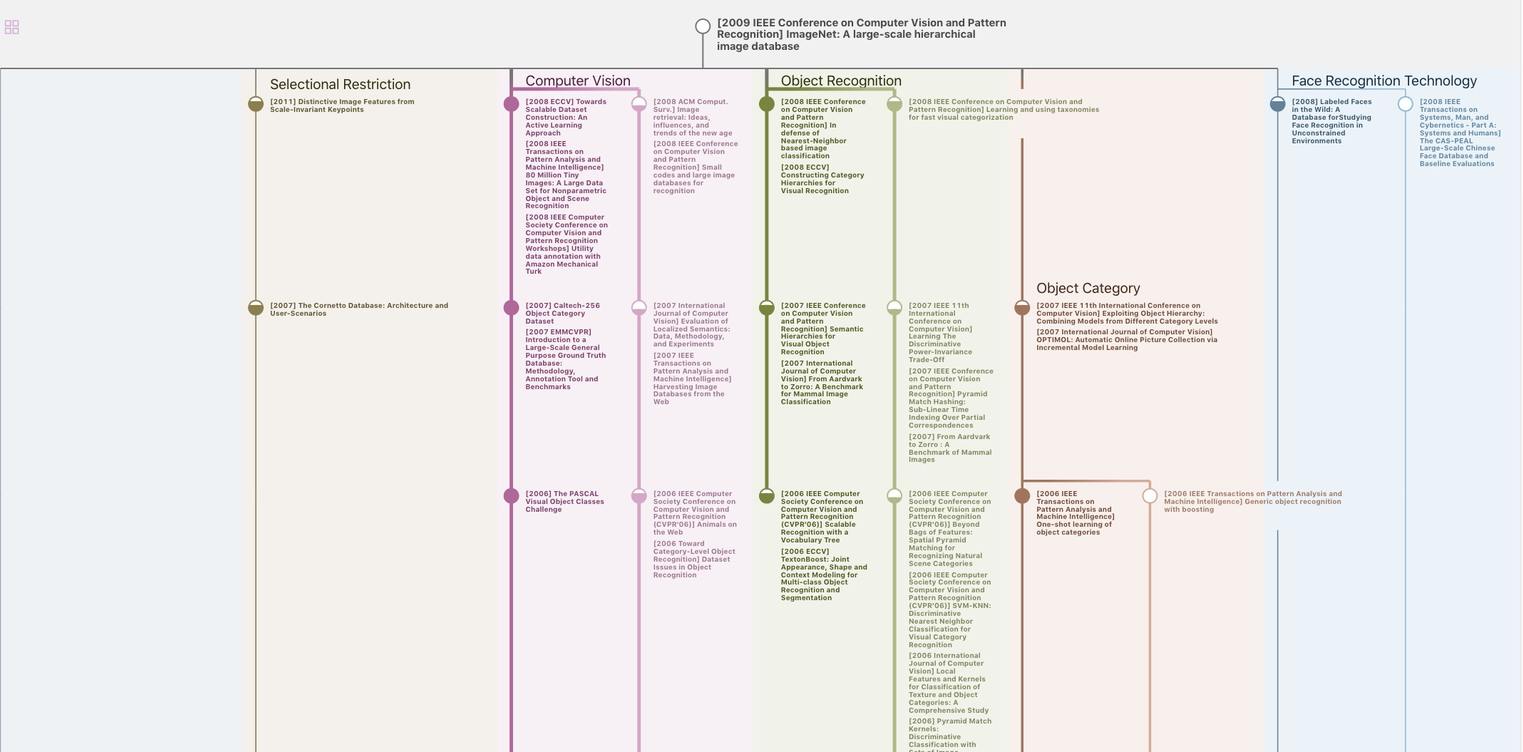
生成溯源树,研究论文发展脉络
Chat Paper
正在生成论文摘要