Learning Unknown Service Rates in Queues: A Multiarmed Bandit Approach
Operational Research(2016)
摘要
Traditional scheduling problems in stochastic queueing systems assume that the statistical parameters are known a priori. In ''Learning unknown service rates in queues: A multiarmed bandit approach'', Krishnasamy, Sen, Johari, and Shakkottai consider the problem of online scheduling in a parallel-server system when the statistical parameters are unknown. They study this question in the stochastic multiarmed bandits framework with the queue length as the performance objective. In contrast to the classic stochastic multiarmed bandits problem, where the regret scales logarithmically with time, the authors show that the queue regret (difference in expected queue length between a bandit algorithm and a genie policy) exhibits a more complex behavior. It grows logarithmically in the initial stage and eventually decays almost inversely with time. This remarkable behavior is explained through the analysis of regenerative cycle lengths, which shorten with time as the bandit algorithm learns to stabilize the queues.
更多查看译文
关键词
bandit algorithms,queueing systems,scheduling,regret
AI 理解论文
溯源树
样例
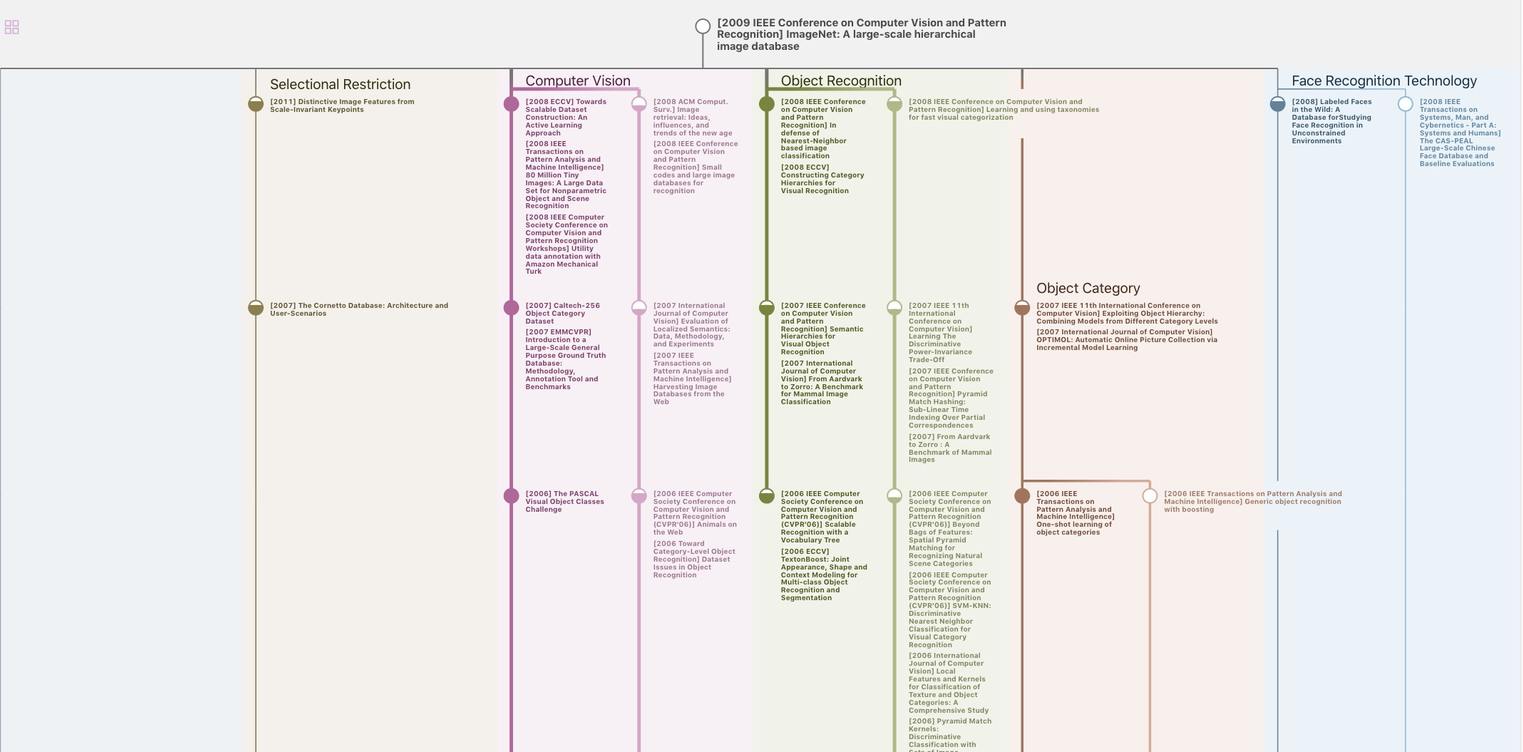
生成溯源树,研究论文发展脉络
Chat Paper
正在生成论文摘要