The role of temporal dependence in factor selection and forecasting oil prices
Empirical Economics(2018)
摘要
Extracting information from high-dimensional time series in the form of underlying factors is an increasingly popular methodology in forecasting applications. In this paper, principal component analysis (PCA) and three other methods for factor extraction are compared based on their deterministic and probabilistic forecasting performances using factor-augmented vector autoregressive (FAVAR) models. The existing PCA-based methods use only the contemporaneous covariance matrix of the data, while the other methods rely on weighted lagged cross-covariance matrices. Our empirical study considers four crude oil future price instruments and a 241 variable dataset of global energy prices and quantity, macroeconomic indicators, and financial series which are thought to influence oil price movements. Overall empirical findings are: (1) the PCA-based method performs better at shorter forecast horizons whereas the new methods involving lagged cross-covariance matrices tend to perform better at longer horizons (2 months or greater); (2) the performance ranking of the four methods under both deterministic and probabilistic forecasting is greatly affected by the number of factors included in the FAVAR models; (3) the forecast performances of the four methods are close to each other and no method performs uniformly better than the others. More research on the role of temporal dependence in determining the number of factors is warranted.
更多查看译文
关键词
Large data set,Probability forecasting,Evaluating forecasts,FAVAR,Energy price forecasting
AI 理解论文
溯源树
样例
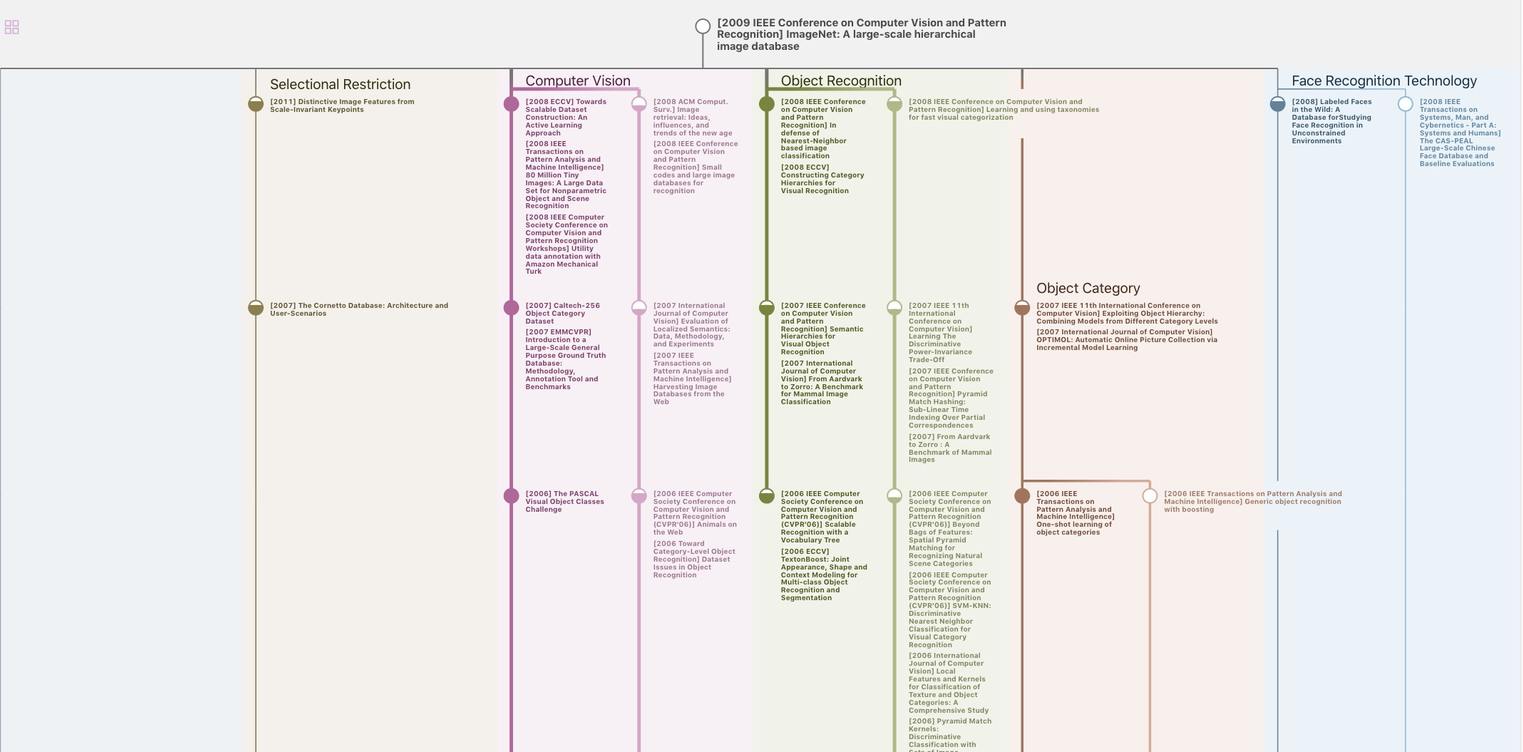
生成溯源树,研究论文发展脉络
Chat Paper
正在生成论文摘要