Reducing The Risk Of Readmission And Death After Hospital Discharge Using A Predictive Model
INTERNATIONAL JOURNAL OF INTEGRATED CARE(2018)
摘要
Introduction: Patient readmission represents a problem, given its impact on their quality of life and for the system, given the associated costs. The transition between hospital and primary care is a milestone associated with readmission. A high rate of readmission may be associated with poor health care provision and no ideal coordination between health care levels. Recently, various predictive models of readmission have been developed. One of these, the LACE index, has been developed and validated for the prediction of readmission and death within 30 days after hospital discharge. This easy-to-use index has been tested in different populations, providing inconclusive results. It has been recommended that it should first be validated in each target population before use. Interventions designed to ensure continuity of care between different levels of care could be effective in reducing the rate of readmission. It remains unclear which of them are the most effective. Objective : To validate a clinical prediction tool for hospital readmission and death to identify patients at high risk of them and to develop an intervention to reduce readmission and mortality. Design : We elaborated a predictive model based on the LACE index using data from patients discharged from hospital between February and March 2013 in two health areas, Bidasoa and Uribe Basque Country, Spain. We validated the model with a retrospective sample from 2012. The intervention was conducted in patients discharged between March and December 2014. Nurses carried out a structured telephone follow-up after hospital discharge. Normal practice was continued for the other patients.MAIN OUTCOME MEASURES: Performance indices of the predictive model. Differences in readmission and mortality rates. Results : Our model showed: Youden Index 0.360; sensitivity: 0.563; specificity 0.797. The data from the retrospective study confirm these results. We observed significant reductions in the risk of readmission or death in both intervention groups, adjusted OR= 0.38 0.28-1.50, showing the validity of the predictive model and the effectiveness of the intervention. Discussions : The UriBi LACE prediction tool has discriminatory power, with a C statistic of 0.74 for a cut-off of 6. Although the Youden index indicated a better discriminatory power for a cut-off of 3, this would imply additional follow-up of 45% of patients discharged; a J of 6 or higher would imply follow-up of 15.6% of discharged patients, which would be more feasible. Conclusions : The tool identify patients at high risk of hospital readmission or death. A structured intervention focused on improving patient knowledge about their condition, warning signs, usual medication and follow-up plan is effective. Lessons learned : We retrieve data on the variables included in the tool directly from the electronic health records. This enables us to identify a high-risk population that benefit from an intervention to strengthen the continuity of care after discharge from hospitals. Limitations : The sample size for the prospective study. We established a follow-up of 1 month, and expected to have more admissions, readmissions and deaths. This lead to a lack of accuracy in the tool, which had to be validated with data from the retrospective study.
更多查看译文
关键词
hospital readmissions, mortality, comorbidity, care continuity
AI 理解论文
溯源树
样例
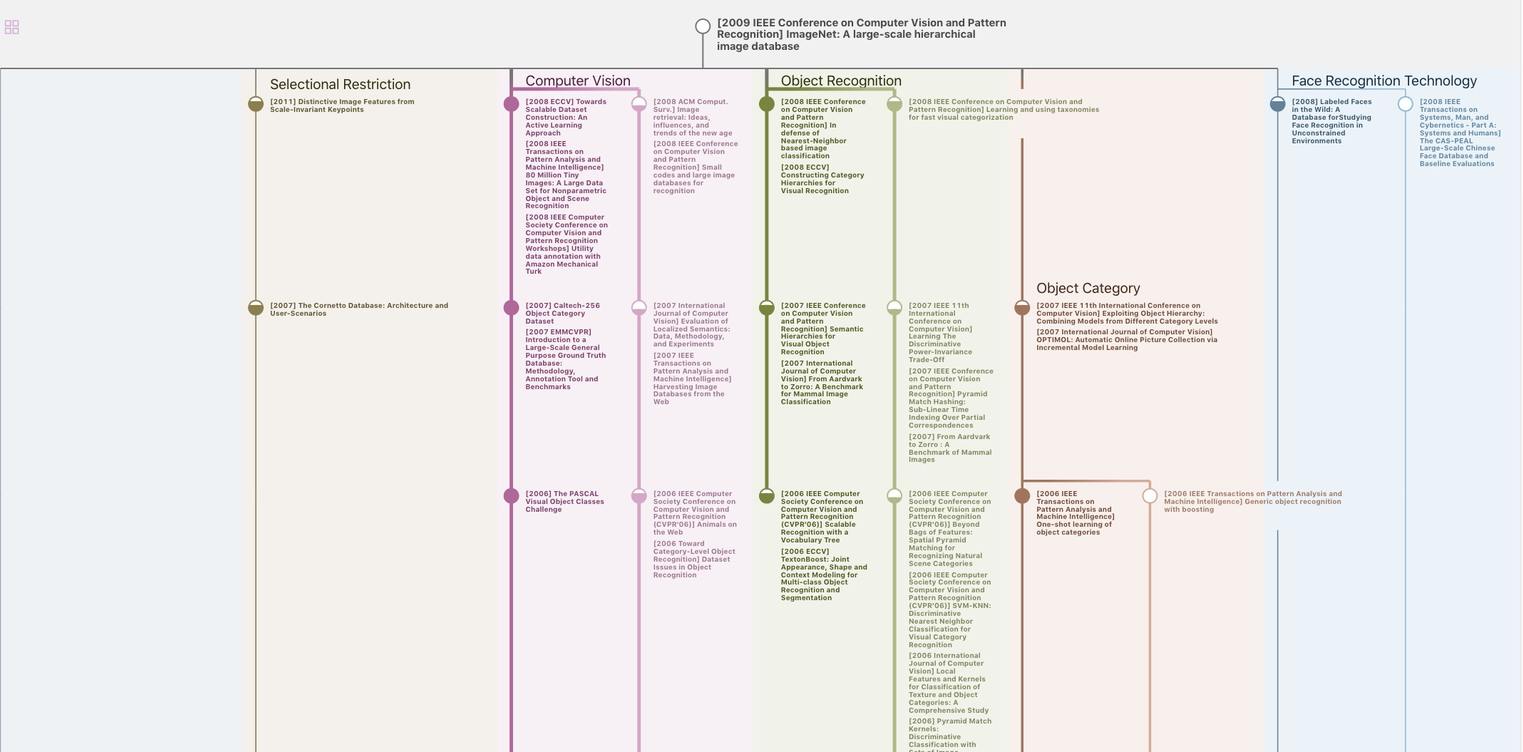
生成溯源树,研究论文发展脉络
Chat Paper
正在生成论文摘要