Adaptive Beamforming for Uniformly-Spaced Linear Hydrophone Array Using Temporal Convolutional Neural Networks
The Journal of the Acoustical Society of America/The journal of the Acoustical Society of America(2018)
摘要
In oceanic remote sensing, large discrete linear hydrophone arrays are usually utilized to enhance the signal-to-noise ratio (SNR) by means of beamforming that attenuates noise coming from the directions outside the target direction. Although the widely adopted delay-and-sum (DS) and filter-and-sum (FS) beamforming techniques can improve the performance of linear hydrophone arrays in some scenarios, both DS and FS methods have limited adaptability to the changing oceanic environments especially with spatially correlated noise. Moreover, these beamforming techniques aims to optimize the SNR, which is not completely consistent with some objectives such as target recognition. In this work, a neural network adaptive beamforming framework for an uniformly-spaced linear hydrophone array is proposed to make use of the large-scale array signals and address the above issues. In particular, an architecture consists of temporal convolutional neural networks is designed to predict the beamforming filter coefficients in time domain by taking the raw multi-channel waveforms of sound pressure as the input. The filter prediction networks are also jointly trained with a convolutional neural network (CNN) based classification model with the purpose of increasing the target recognition accuracy. The proposed approach is validated by experiments carried out in the south coastal waters of China.
更多查看译文
AI 理解论文
溯源树
样例
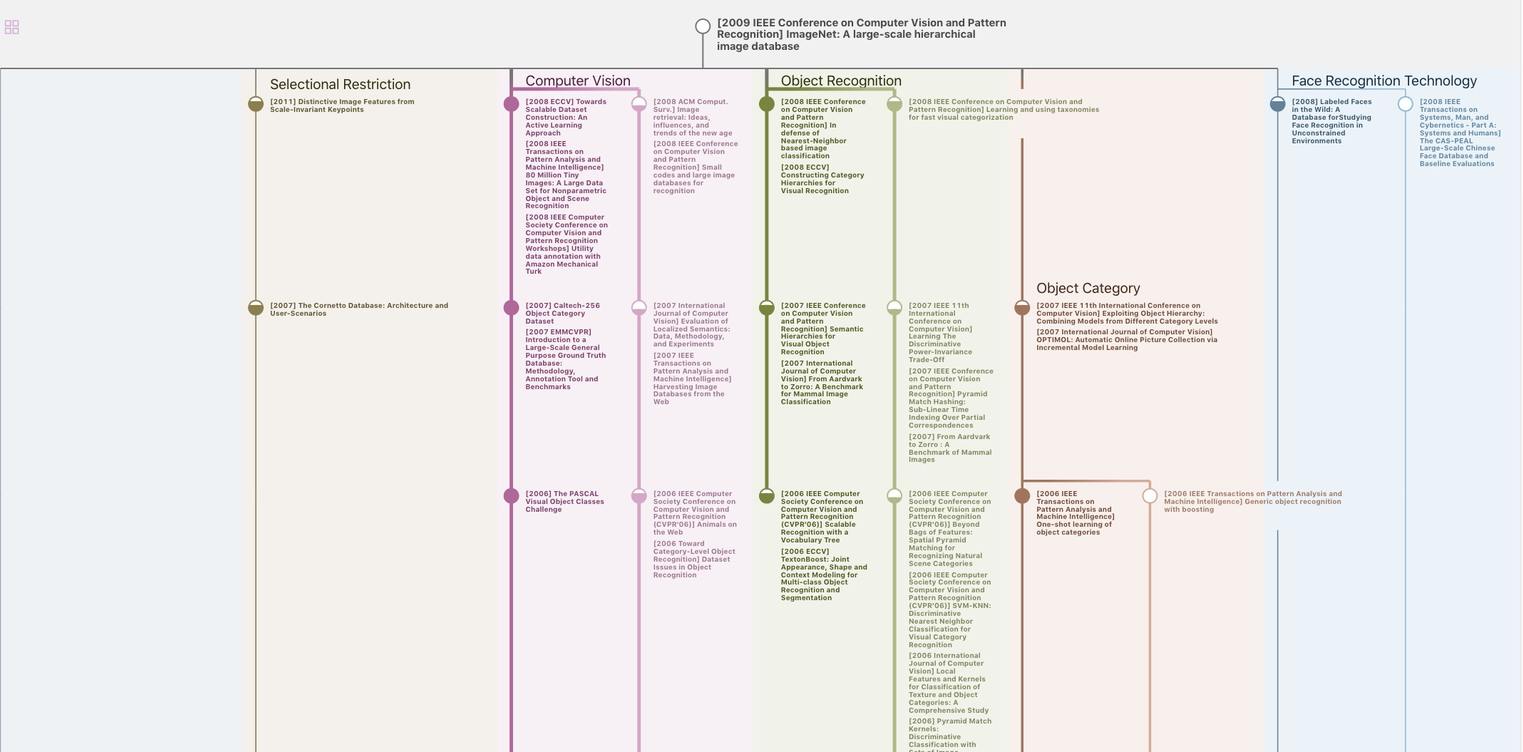
生成溯源树,研究论文发展脉络
Chat Paper
正在生成论文摘要