Multi-Scale Super-Resolution Reconstruction via a Deep Winner-Take-All Cascaded NetWork
2018 IEEE 3rd International Conference on Image, Vision and Computing (ICIVC)(2018)
摘要
Convolutional neural networks have been recently shown to have the highest accuracy for single-image super-resolution reconstruction (SISR). In this paper, we propose a Deep Winner-take-all Cascaded Network (DWTACN) that can gradually reconstruct robust images in multiple scales. This endeavor is achieved through a new network structure with two interacting sub-networks: a deep cascaded multi-branch prediction network (DCMBPN) and a deep block selection network (DBSN). In particular, in each cascade, DCMBPN predicts multiple reconstructed images progressively with its special winner-take-all and cascaded structure. DBSN then adaptively selects the predicted confident blocks from these reconstructed images. Our method does not require traditional interpolation methods to up sample the image as a preprocessing step. It thus greatly reduces the computational complexity. We use L2 and perception loss functions in each branch to obtain two high-quality reconstructions. In addition, for the cascade structure, our network can achieve reconstructions in different scales, such as 1.5x, 2x, 2.5x, 3x, 3.5x and 4x. Results of extensive experiments on several large benchmark datasets show that the proposed approach provides superior performance compared to existing methods in terms of accuracy and visual improvement. (Abstract)
更多查看译文
关键词
single image super-resolution,multi-scale,winner-take-all,cascaded,convolutional neural networks
AI 理解论文
溯源树
样例
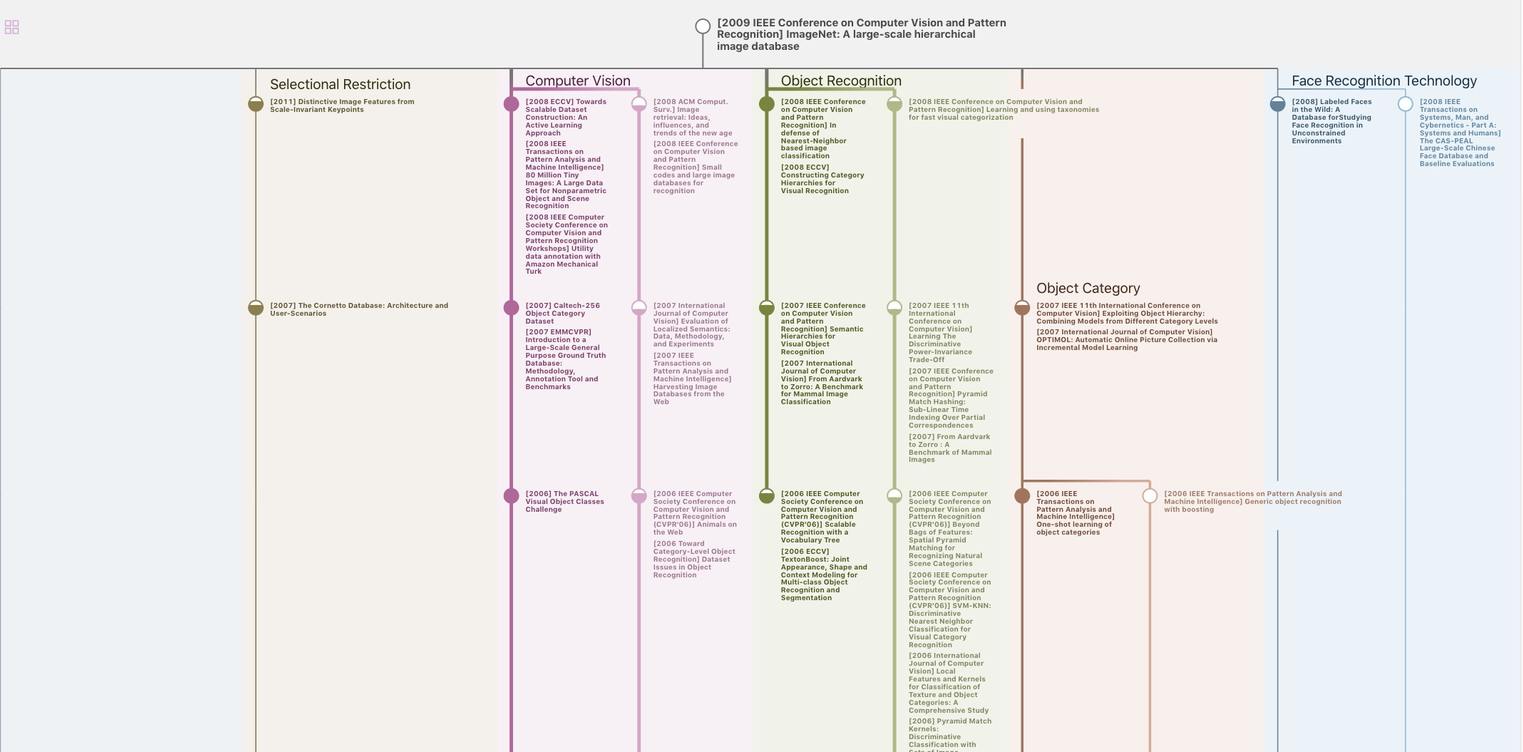
生成溯源树,研究论文发展脉络
Chat Paper
正在生成论文摘要