Quantifying The Model Risk Inherent In The Calibration And Recalibration Of Option Pricing Models
RISKS(2021)
摘要
We focus on two particular aspects of model risk: the inability of a chosen model to fit observed market prices at a given point in time (calibration error) and the model risk due to the recalibration of model parameters (in contradiction to the model assumptions). In this context, we use relative entropy as a pre-metric in order to quantify these two sources of model risk in a common framework, and consider the trade-offs between them when choosing a model and the frequency with which to recalibrate to the market. We illustrate this approach by applying it to the seminal Black/Scholes model and its extension to stochastic volatility, while using option data for Apple (AAPL) and Google (GOOG). We find that recalibrating a model more frequently simply shifts model risk from one type to another, without any substantial reduction of aggregate model risk. Furthermore, moving to a more complicated stochastic model is seen to be counterproductive if one requires a high degree of robustness, for example, as quantified by a 99% quantile of aggregate model risk.
更多查看译文
关键词
model risk, option pricing, relative entropy, model calibration, stochastic volatility
AI 理解论文
溯源树
样例
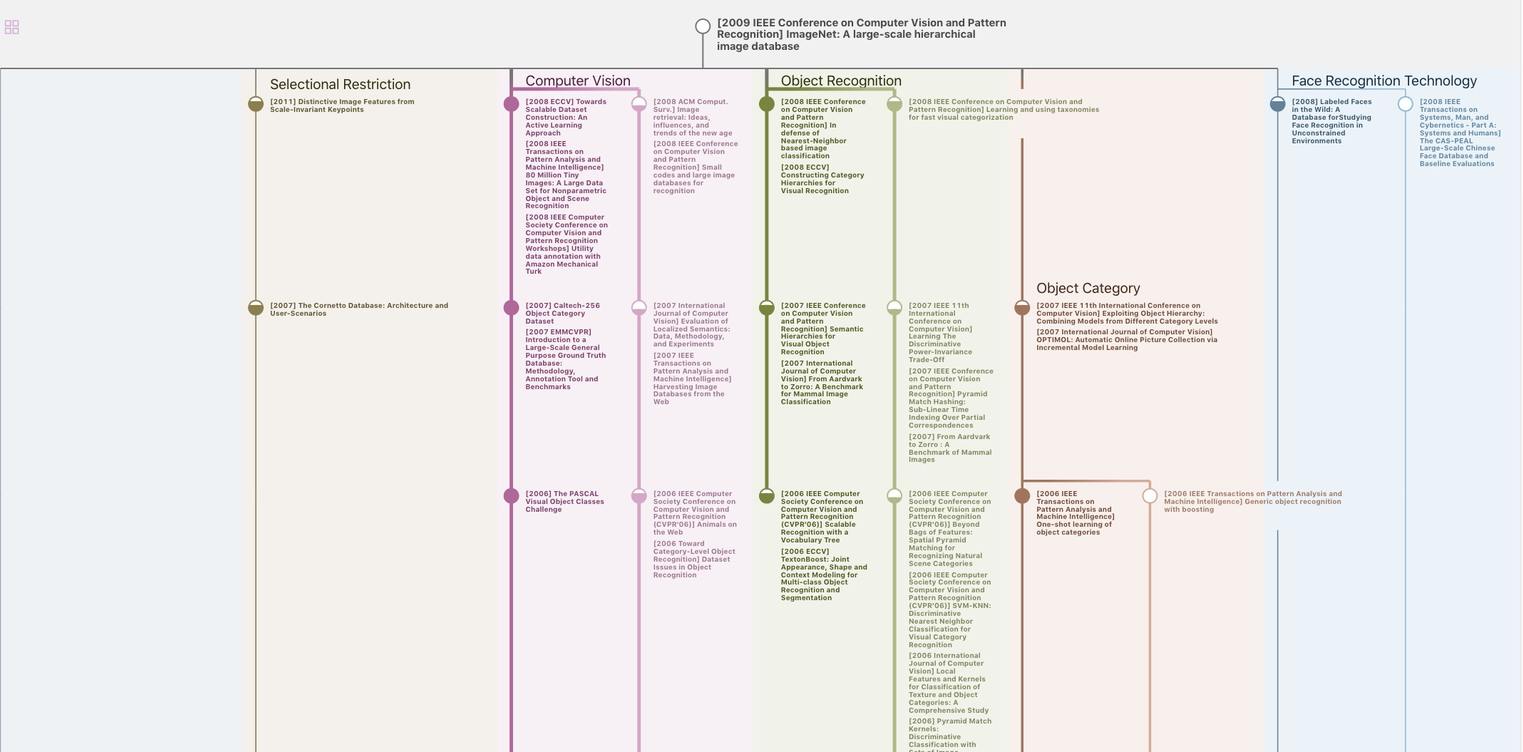
生成溯源树,研究论文发展脉络
Chat Paper
正在生成论文摘要