PANOPLY: A computational method for identification of promising drugs for a patient based on multidimensional data
bioRxiv(2018)
摘要
The majority of cancer patients receive treatments that are minimally informed by omics data. Our goal was to develop a precision medicine computational framework (PANOPLY: Precision cancer genomic report: single sample inventory) to identify and prioritize drug targets and cancer therapy regimens. The PANOPLY approach integrates clinical data with germline and somatic features obtained from multi-omics platforms, and apply machine learning, and network analysis approaches in the context of the individual patient and matched controls. The PANOPLY employs multiple steps including i) selection of matched controls, ii) preprocessing of data and identification of driver mutations and altered genes in the patient, iii) identification of suitable drugs using the driver-gene network and random forest analysis, and iv) a multi-omics case report of the patient with prioritization of anti-cancer drugs. The PANOPLY framework can be executed on a stand-alone virtual machine and is also available for download as an R package. We applied PANOPLY to multiple publicly accessible multi-omics tumor datasets with survival data available. We also applied the method to an institutional breast cancer neoadjuvant chemotherapy study which collected clinical and genomic data as well as patient-derived xenografts (PDXs) to investigate the prioritization offered by PANOPLY. We found that that the prioritized drug, olaparib, was more effective than placebo at treating the tumor in the chemotherapy resistant PDX model (P u003c 0.05). Further studies are ongoing to determine the efficacy of PANOPLY using additional PDXs. In summary, PANOPLY prioritizes drugs based on both clinical and multi-omics data, and it can aid oncologists in their decision-making for therapy in the individual patient.
更多查看译文
AI 理解论文
溯源树
样例
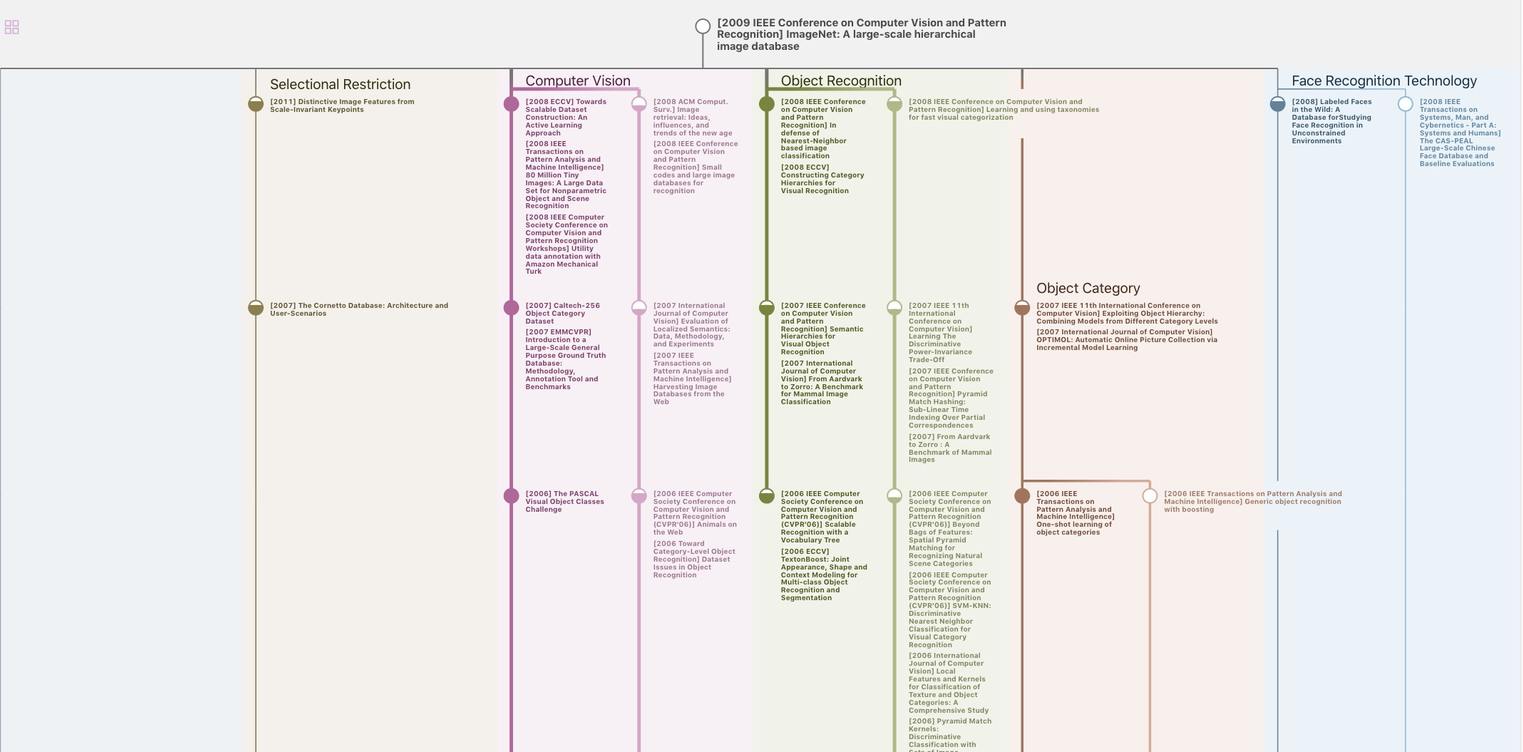
生成溯源树,研究论文发展脉络
Chat Paper
正在生成论文摘要