Comprehensive Analysis of Deep Learning based Vehicle Detection in Aerial Images
IEEE Transactions on Circuits and Systems for Video Technology(2018)
摘要
Vehicle detection in aerial images is a crucial image processing step for many applications like screening of large areas as used for surveillance, reconnaissance or rescue tasks. In recent years, several deep learning based frameworks have been proposed for object detection. However, these detectors were developed for datasets that considerably differ from aerial images. In this article, we systematically investigate the potential of Fast R-CNN and Faster R-CNN for aerial images, which achieve top performing results on common detection benchmark datasets. Therefore, the applicability of eight state-of-the-art object proposal methods used to generate a set of candidate regions and of both detectors is examined. Relevant adaptations to account for the characteristics of the aerial images are provided. To overcome the shortcomings of the original approach in case of handling small instances, we further propose our own networks that clearly outperform state-of-the-art methods for vehicle detection in aerial images. Furthermore, we analyze the impact of the different adaptations with respect to various ground sampling distances to provide a guideline for detecting small objects in aerial images. All experiments are performed on two publicly available datasets to account for differing characteristics such as varying object sizes, number of objects per image and varying backgrounds.
更多查看译文
关键词
Proposals,Microsoft Windows,Vehicle detection,Machine learning,Image edge detection,Detectors,Image segmentation
AI 理解论文
溯源树
样例
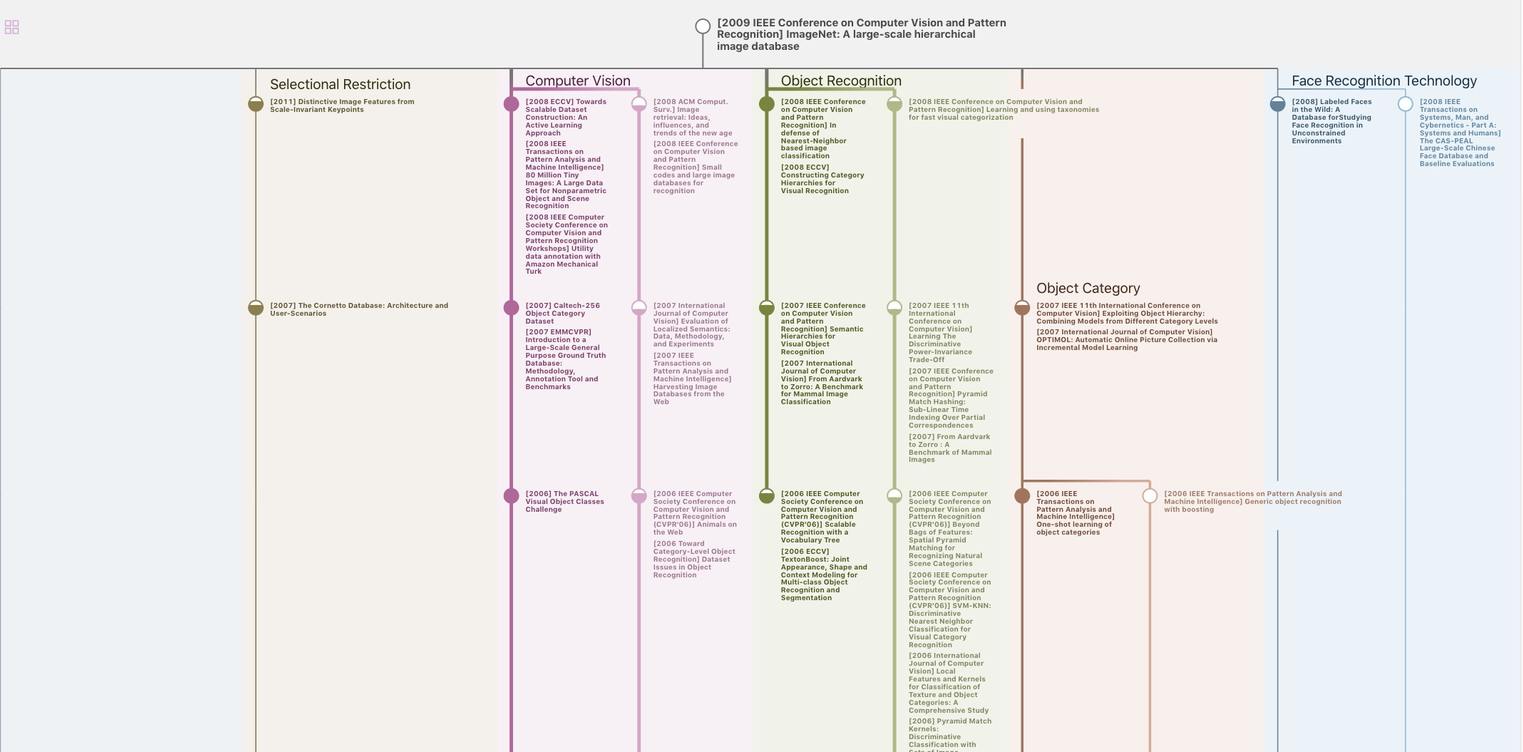
生成溯源树,研究论文发展脉络
Chat Paper
正在生成论文摘要