Assessment of current capabilities in modeling the ionospheric climatology for space weather applications: foF2 and hmF2
SPACE WEATHER-THE INTERNATIONAL JOURNAL OF RESEARCH AND APPLICATIONS(2018)
摘要
This paper presents preliminary results of a metrics-based assessment of current modeling capabilities in predicting the ionospheric climatology for two ionospheric characteristics, the foF2 and hmF2. This work is part of the activities of the Ionosphere Working Group of the International Forum for Space Weather Modeling Capabilities Assessment that is facilitated by the Community Coordinated Modeling Center. The results are based on the comparison between modeled and observed monthly medians of the foF2 and hmF2. Modeling formulations considered here include two empirical models (the International Reference Ionosphere [IRI] and Massachusetts Institute of Technology [MIT] Empirical model), as well as two physics-based, coupled ionosphere-thermosphere models (the Coupled Thermosphere Ionosphere Plasmasphere Electrodynamics [CTIPe] and Thermosphere-Ionosphere-Electrodynamics General Circulation Model [TIE-GCM]). The comparisons were carried out in year 2012 over seven ionospheric locations distributed worldwide in the Northern Hemisphere. Based on our findings, although significant errors are occasionally obtained from all modeling approaches, empirical models tend to provide systematically better correlation with the observed medians and follow the observed distributions more successfully, offering smaller prediction errors than the physics-based ones. In case of foF2, the averaged prediction accuracy in terms of root-mean-square error (RMSE) ranges around 1.5 MHz for physics-based models and 0.5 MHz for empirical models. In case of hmF2, it ranges around 30 and 20 km, respectively. The prediction accuracy presents strong seasonal and local time dependence, which differs for different models and ionospheric characteristics (i.e., foF2 or hmF2): it is strong for physics-based models, weaker for IRI, and very weak for MIT empirical model. This latter outcome may provide suggestive input for future improvements.
更多查看译文
AI 理解论文
溯源树
样例
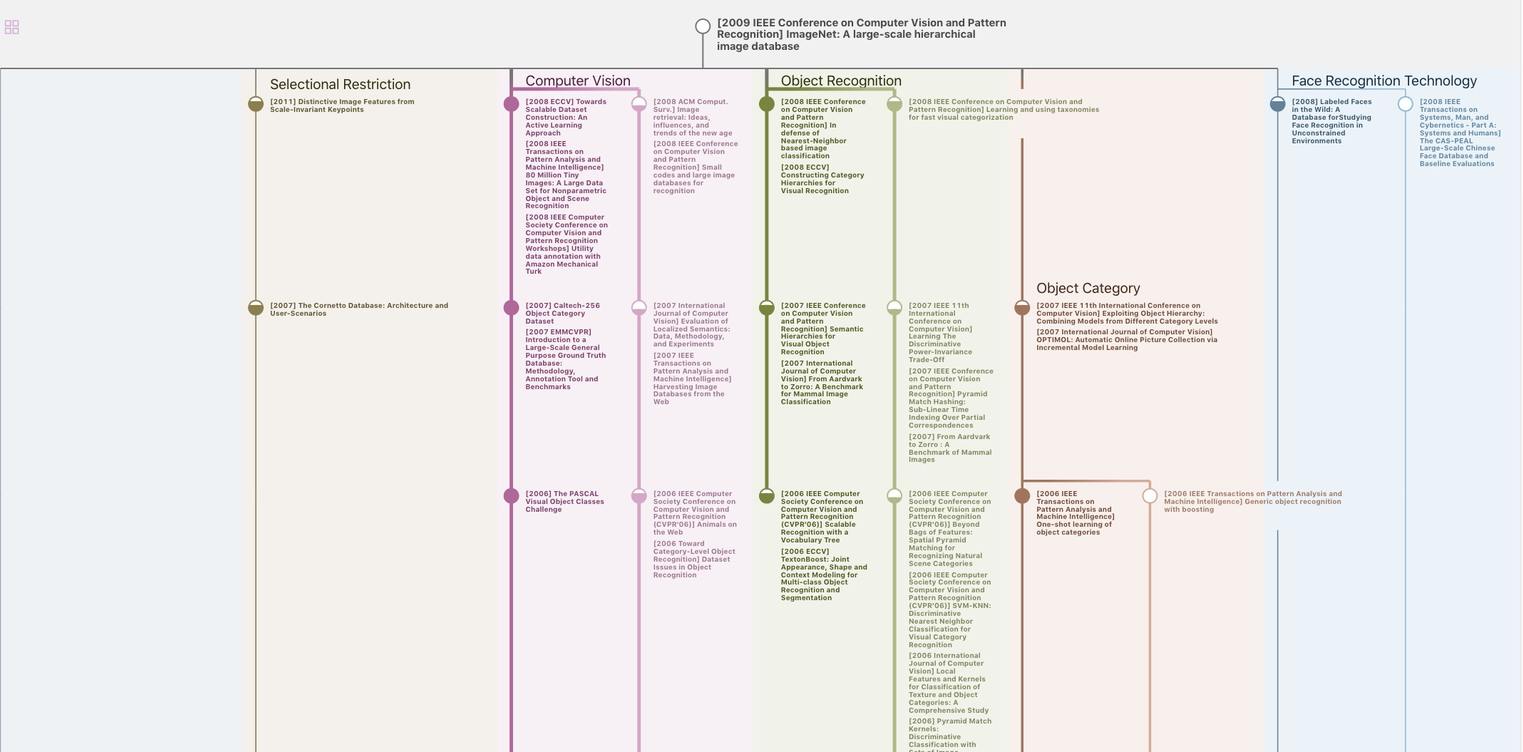
生成溯源树,研究论文发展脉络
Chat Paper
正在生成论文摘要